The Smoothed Complexity of Policy Iteration for Markov Decision Processes
PROCEEDINGS OF THE 55TH ANNUAL ACM SYMPOSIUM ON THEORY OF COMPUTING, STOC 2023(2023)
摘要
We showsubexponential lower bounds (i.e., 2(Omega(nc))) on the smoothed complexity of the classical Howard's Policy Iteration algorithm for Markov Decision Processes. The bounds hold for the total reward and the average reward criteria. The constructions are robust in the sense that the subexponential bound holds not only on the average for independent random perturbations of the MDP parameters (transition probabilities and rewards), but for all arbitrary perturbations within an inverse polynomial range. We show also an exponential lower bound on the worst-case complexity for the simple reachability objective.
更多查看译文
关键词
Policy Iteration,Smoothed Analysis
AI 理解论文
溯源树
样例
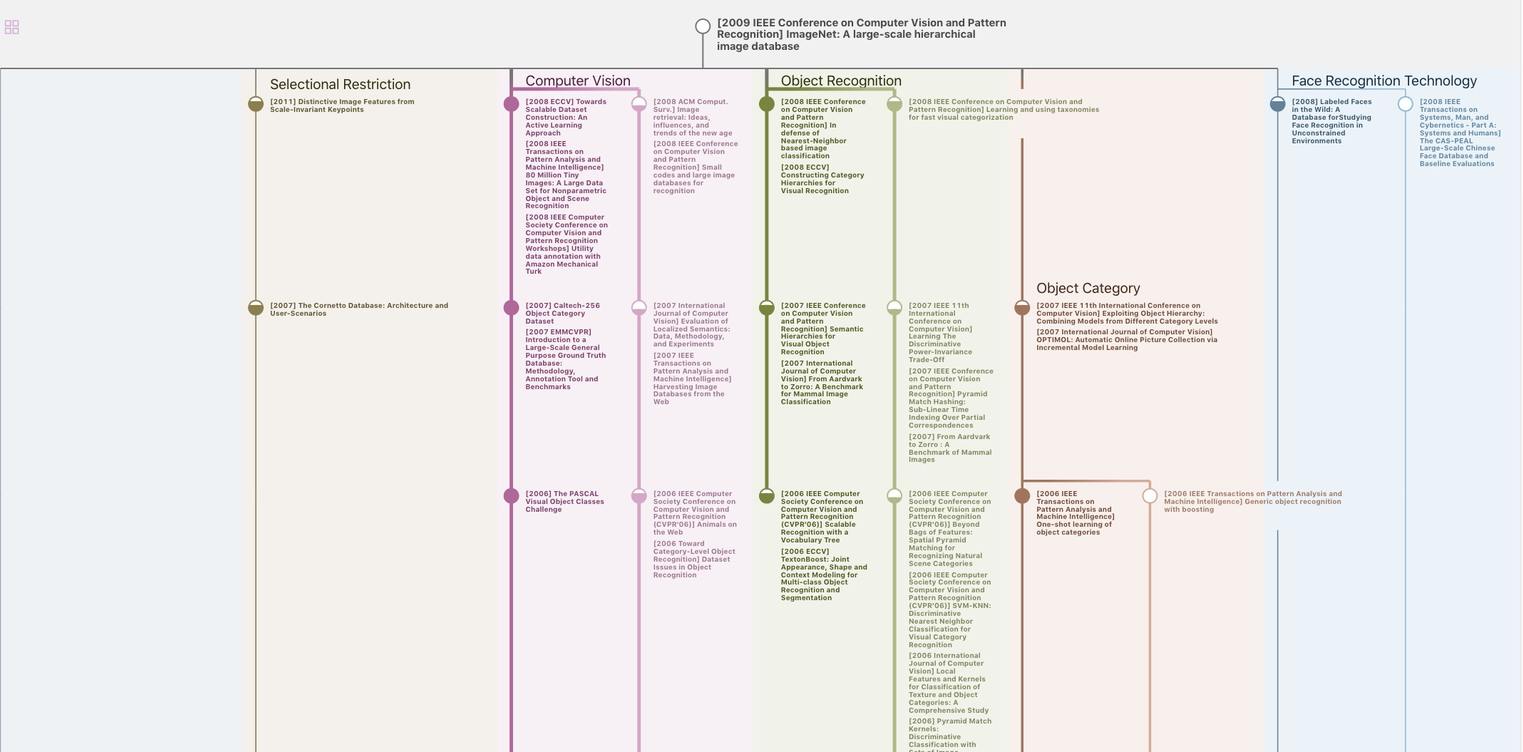
生成溯源树,研究论文发展脉络
Chat Paper
正在生成论文摘要