Work-in-Progress: Toward Energy-efficient Near STT-MRAM Processing Architecture for Neural Networks
2022 International Conference on Hardware/Software Codesign and System Synthesis (CODES+ISSS)(2022)
摘要
The size of parameters in artificial neural network (NN) applications grows quickly from a handful to the GB-level. The data transmission poses a key challenge for NN, and either neuron is removed or data compression reduces pressure on memory access but cannot successfully decrease data traffic. Therefore, we propose the near spin-transfer-torque magnetic random processing architecture for developing energy-efficient NNs. Our approach provides system architects with a preliminary scheme to obtain real-time transmission that near memory controller directly compresses non-zero elements, and encodes the corresponding index depending on the kernel size. Furthermore, it adjusts the number of multiplication accumulators and avoids unnecessary hardware overheads during computation. The preliminary experimental results demonstrated this design verified with weights that currently achieve up to 3.05x speedup and 29.6% power compared with the unoptimized one.
更多查看译文
关键词
STT-MRAM,Near-memory Processing,Neural Network,Energy-efficient
AI 理解论文
溯源树
样例
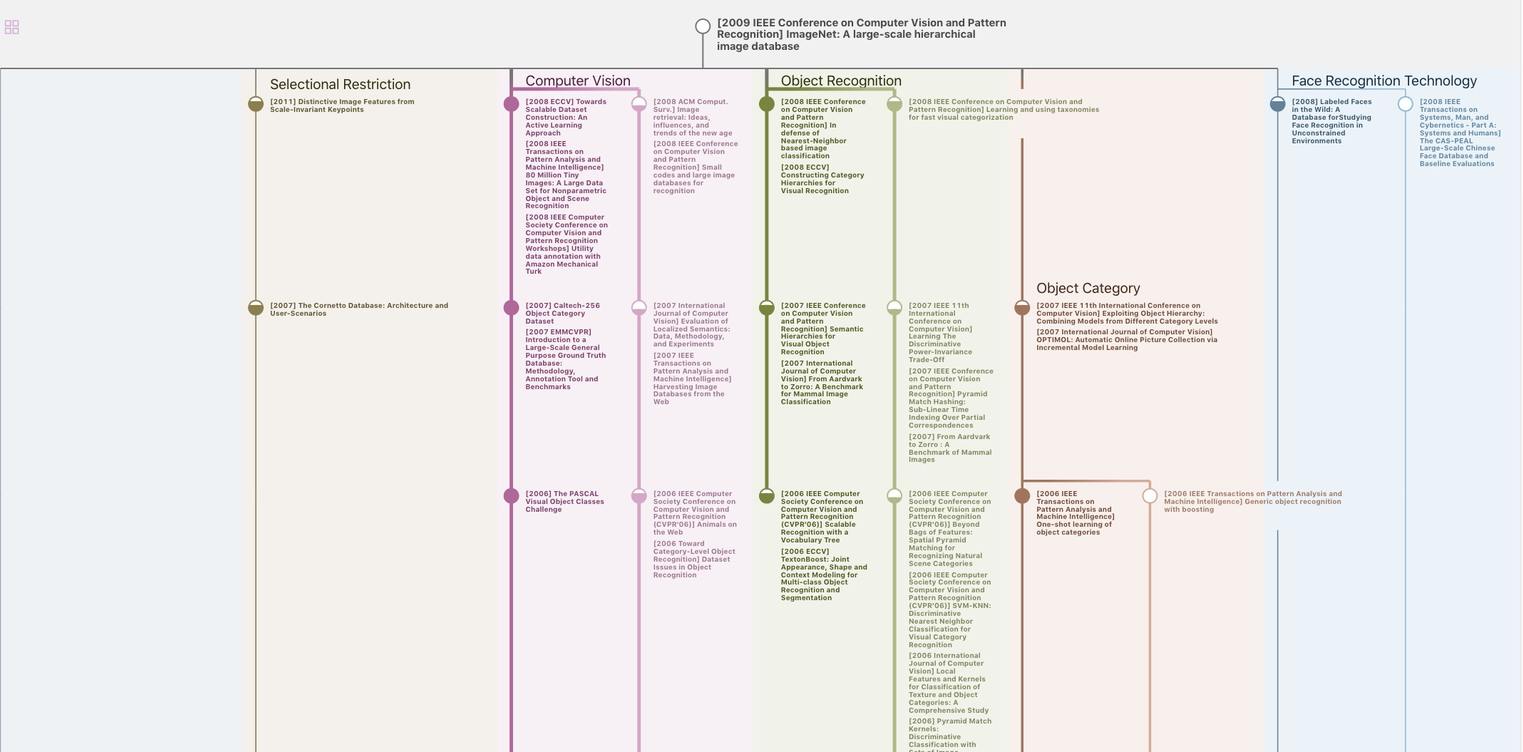
生成溯源树,研究论文发展脉络
Chat Paper
正在生成论文摘要