Digital twin of an industrial workstation: A novel method of an auto-labeled data generator using virtual reality for human action recognition in the context of human–robot collaboration
Engineering Applications of Artificial Intelligence(2023)
Abstract
The recognition of human actions based on artificial intelligence methods to enable Human–Robot Collaboration (HRC) inside working environments remains a challenge, especially because of the necessary huge training datasets needed. Meanwhile, Digital Twins (DTs) of human centered productions are increasingly developed and used in the design and operation phases. As instance, DTs are already helping industries to design, visualize, monitor, manage, and maintain their assets more effectively. However, few works are dealing with using DTs as a dataset generator tool. Therefore, this paper explores the use of a DT of a real industrial workstation involving assembly tasks with a robotic arm interfaced with Virtual Reality (VR) to extract a digital human model. The DT simulates assembly operations performed by humans aiming to generate self-labeled data. Thereby, a Human Action Recognition dataset named InHARD-DT was created to validate a real use case in which we use the acquired auto-labeled DT data of the virtual representation of the InHARD dataset to train a Spatial–Temporal Graph Convolutional Neural Network with skeletal data on one hand. On the other hand, the Physical Twin (PT) data of the InHARD dataset was used for testing. Obtained results show the effectiveness of the proposed method.
MoreTranslated text
Key words
Digital Twins (DTs),Industry 4.0,Auto-labeled data generation,Virtual Reality (VR),Human Action Recognition (HAR),Human–Robot Collaboration (HRC)
AI Read Science
Must-Reading Tree
Example
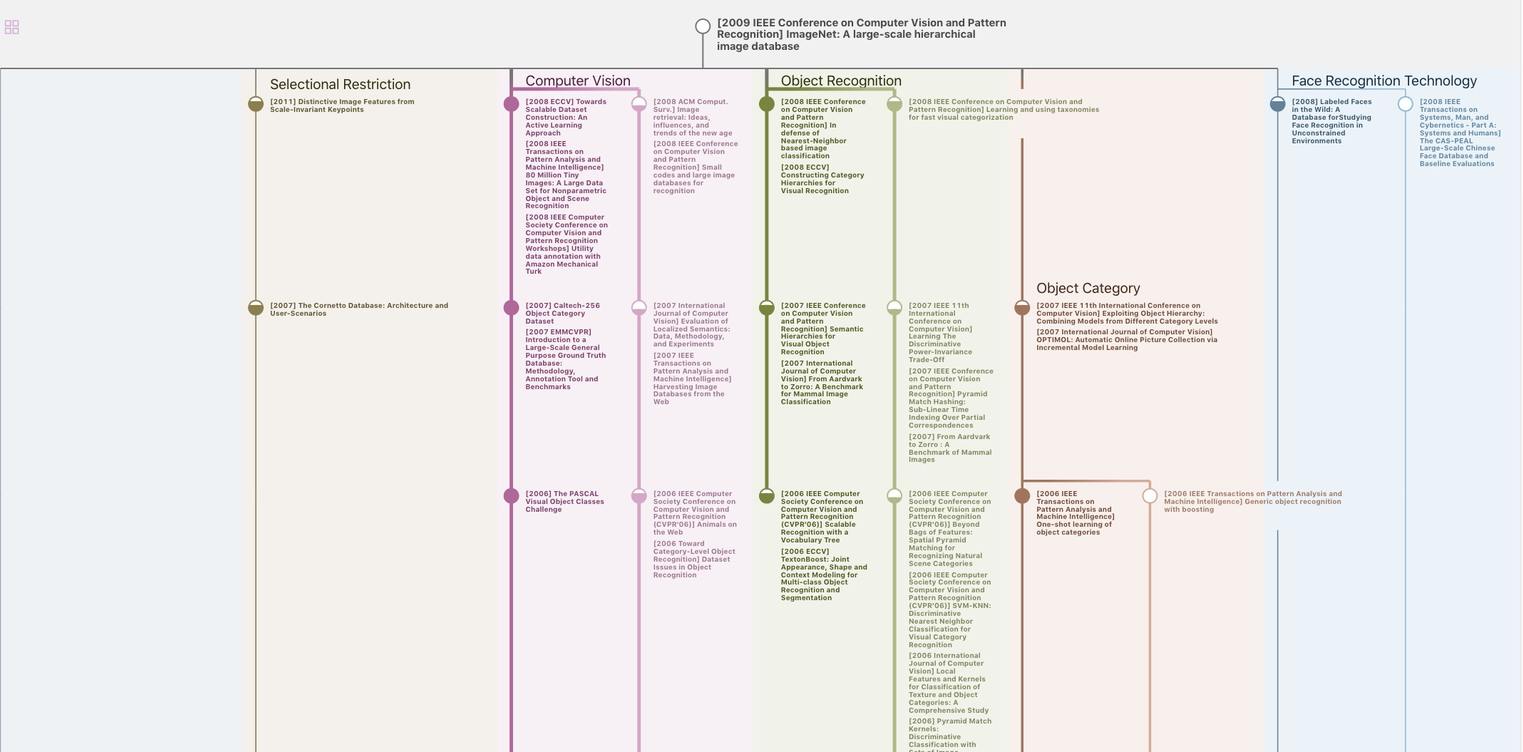
Generate MRT to find the research sequence of this paper
Chat Paper
Summary is being generated by the instructions you defined