Cross-dataset performance evaluation of deep learning distracted driver detection algorithms
MATEC Web of Conferences(2022)
Abstract
Deep learning has gained traction due its supremacy in terms of accuracy and ability to automatically learn features from input data. However, deep learning algorithms can sometimes be flawed due to many factors such as training dataset, parameters, and choice of algorithms. Few studies have evaluated the robustness of deep learning distracted driver detection algorithms. The studies evaluate the algorithms on a single dataset and do not consider cross-dataset performance. A problem arises because cross-dataset performance often implies model generalisation ability. Deploying a model in the real world without knowing its cross-dataset performance could lead to catastrophic events. The paper investigates the cross-dataset performance of deep learning distracted driver detection algorithms. Experimental results found reveal that deep learning distracted driver detection algorithms do not generalise well on unknown datasets for CNN models that use the whole image for prediction. The cross-dataset performance evaluations shed light on future research in developing robust deep learning distracted driver detection algorithms.
MoreTranslated text
Key words
deep learning,performance,cross-dataset
AI Read Science
Must-Reading Tree
Example
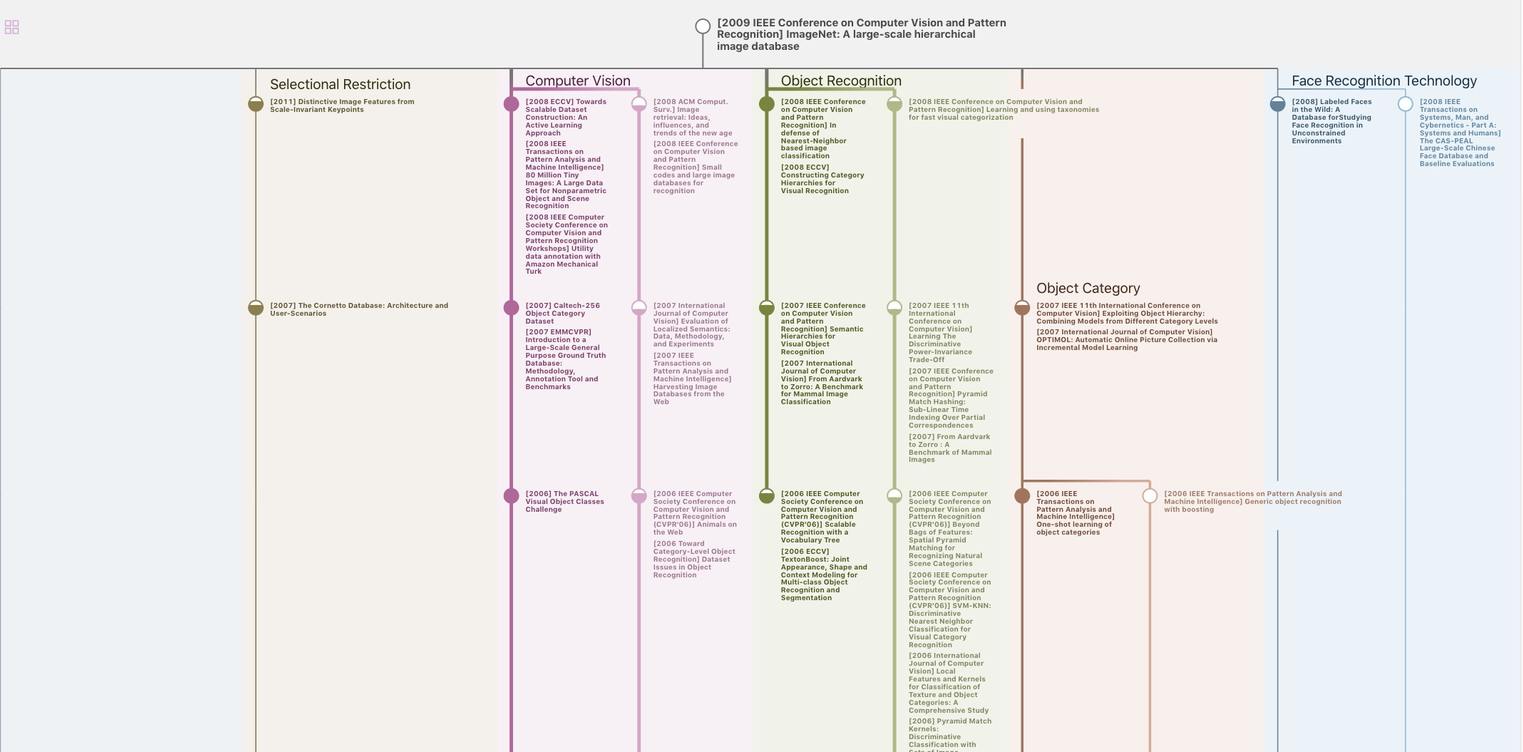
Generate MRT to find the research sequence of this paper
Chat Paper
Summary is being generated by the instructions you defined