Active Pairwise Constraint Learning in Constrained Time-Series Clustering for Crop Mapping from Airborne SAR Imagery
REMOTE SENSING(2022)
摘要
Airborne SAR is an important data source for crop mapping and has important applications in agricultural monitoring and food safety. However, the incidence-angle effects of airborne SAR imagery decrease the crop mapping accuracy. An active pairwise constraint learning method (APCL) is proposed for constrained time-series clustering to address this problem. APCL constructs two types of instance-level pairwise constraints based on the incidence angles of the samples and a non-iterative batch-mode active selection scheme: the must-link constraint, which links two objects of the same crop type with large differences in backscattering coefficients and the shapes of time-series curves; the cannot-link constraint, which links two objects of different crop types with only small differences in the values of backscattering coefficients. Experiments were conducted using 12 time-series images with incidence angles ranging from 21.2 degrees to 64.3 degrees, and the experimental results prove the effectiveness of APCL in improving crop mapping accuracy. More specifically, when using dynamic time warping (DTW) as the similarity measure, the kappa coefficient obtained by APCL was increased by 9.5%, 8.7%, and 5.2% compared to the results of the three other methods. It provides a new solution for reducing the incidence-angle effects in the crop mapping of airborne SAR time-series images.
更多查看译文
关键词
synthetic aperture radar (SAR),crop mapping,time-series images,constrained clustering,active constraint learning
AI 理解论文
溯源树
样例
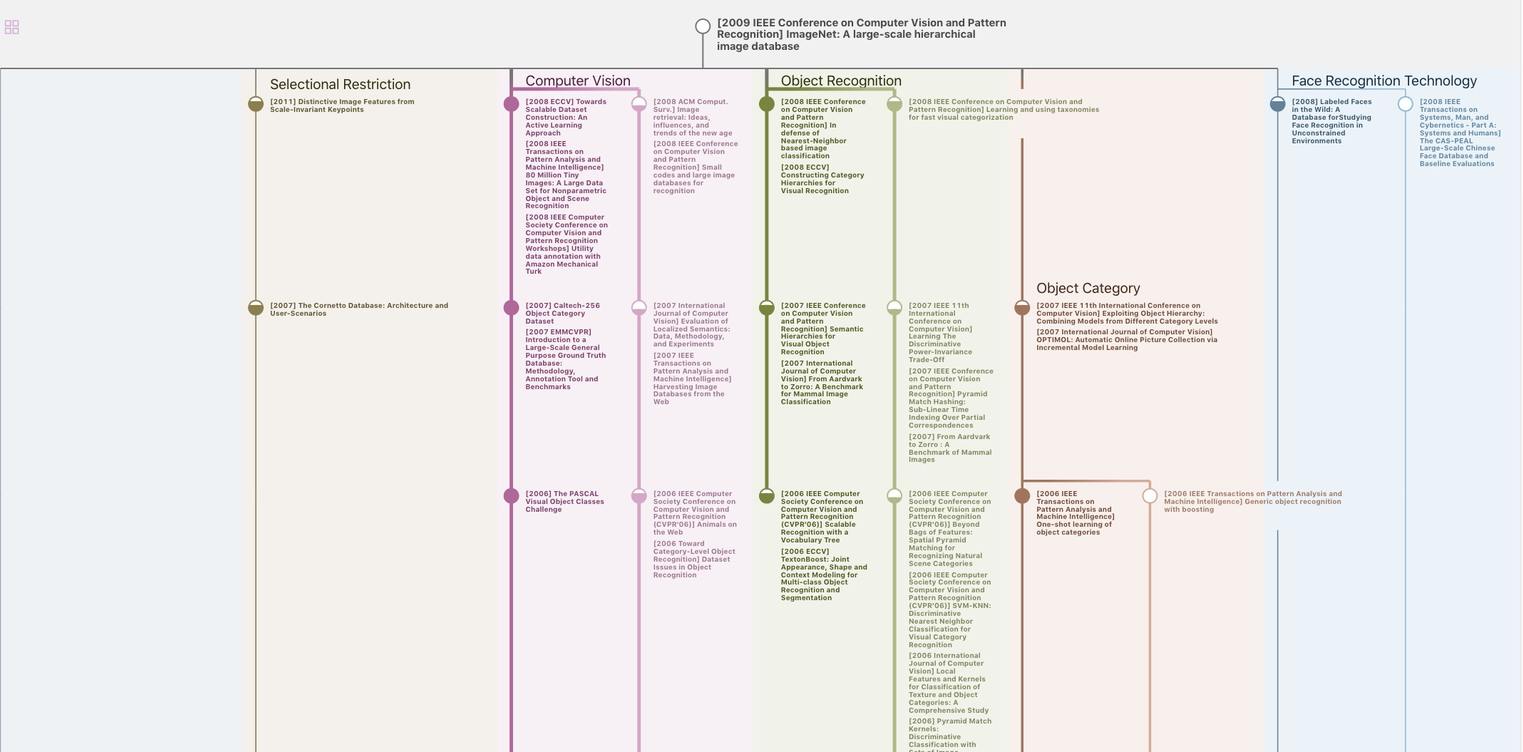
生成溯源树,研究论文发展脉络
Chat Paper
正在生成论文摘要