NeRFInvertor: High Fidelity NeRF-GAN Inversion for Single-Shot Real Image Animation
CVPR 2023(2023)
Abstract
Nerf-based Generative models have shown impressive capacity in generating high-quality images with consistent 3D geometry. Despite successful synthesis of fake identity images randomly sampled from latent space, adopting these models for generating face images of real subjects is still a challenging task due to its so-called inversion issue. In this paper, we propose a universal method to surgically fine-tune these NeRF-GAN models in order to achieve high-fidelity animation of real subjects only by a single image. Given the optimized latent code for an out-of-domain real image, we employ 2D loss functions on the rendered image to reduce the identity gap. Furthermore, our method leverages explicit and implicit 3D regularizations using the in-domain neighborhood samples around the optimized latent code to remove geometrical and visual artifacts. Our experiments confirm the effectiveness of our method in realistic, high-fidelity, and 3D consistent animation of real faces on multiple NeRF-GAN models across different datasets.
MoreTranslated text
Key words
3D from single images
AI Read Science
Must-Reading Tree
Example
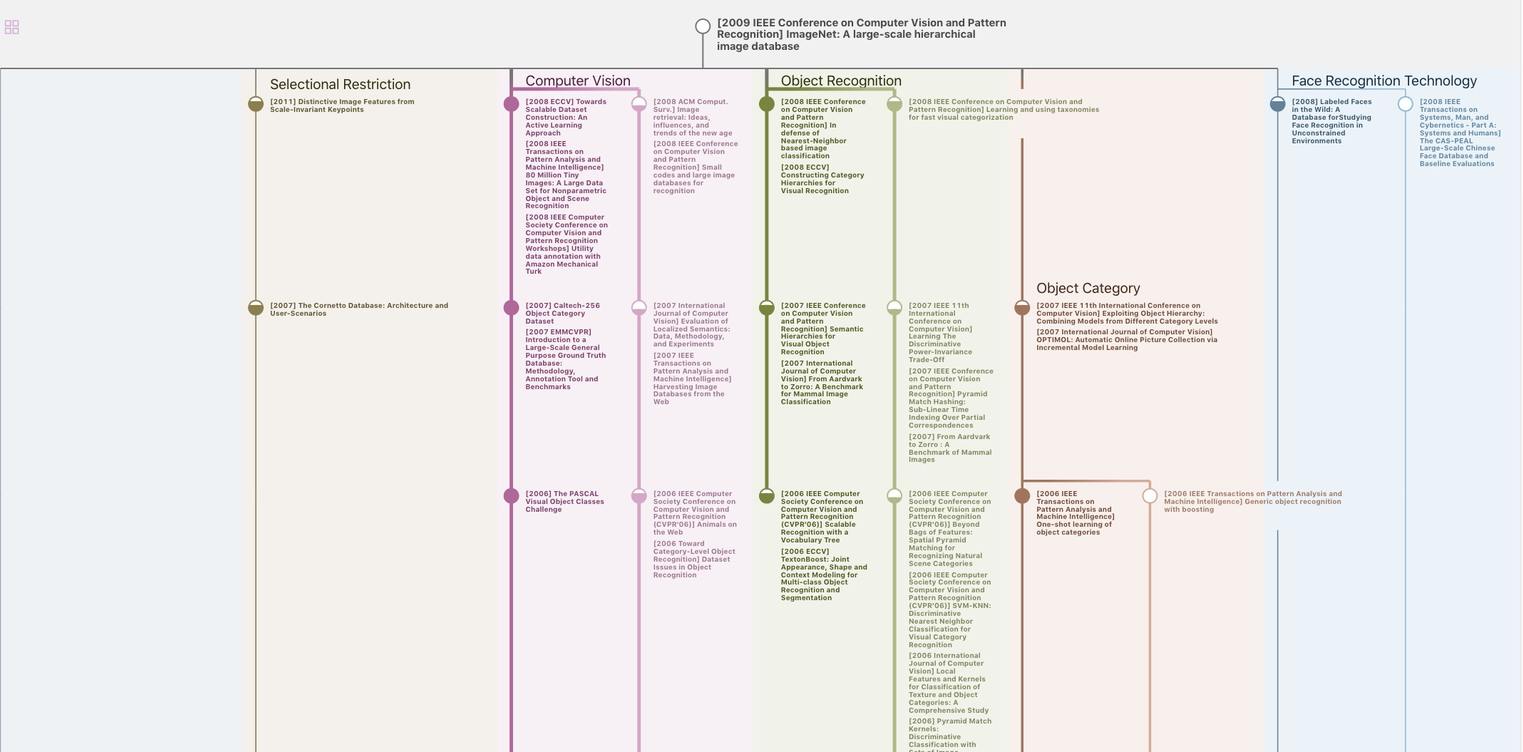
Generate MRT to find the research sequence of this paper
Chat Paper
Summary is being generated by the instructions you defined