Hint-dynamic Knowledge Distillation
arxiv(2022)
摘要
Knowledge Distillation (KD) transfers the knowledge from a high-capacity teacher model to promote a smaller student model. Existing efforts guide the distillation by matching their prediction logits, feature embedding, etc., while leaving how to efficiently utilize them in junction less explored. In this paper, we propose Hint-dynamic Knowledge Distillation, dubbed HKD, which excavates the knowledge from the teacher' s hints in a dynamic scheme. The guidance effect from the knowledge hints usually varies in different instances and learning stages, which motivates us to customize a specific hint-learning manner for each instance adaptively. Specifically, a meta-weight network is introduced to generate the instance-wise weight coefficients about knowledge hints in the perception of the dynamical learning progress of the student model. We further present a weight ensembling strategy to eliminate the potential bias of coefficient estimation by exploiting the historical statics. Experiments on standard benchmarks of CIFAR-100 and Tiny-ImageNet manifest that the proposed HKD well boost the effect of knowledge distillation tasks.
更多查看译文
关键词
Knowledge Distillation,Dynamic Network,Meta-Learning
AI 理解论文
溯源树
样例
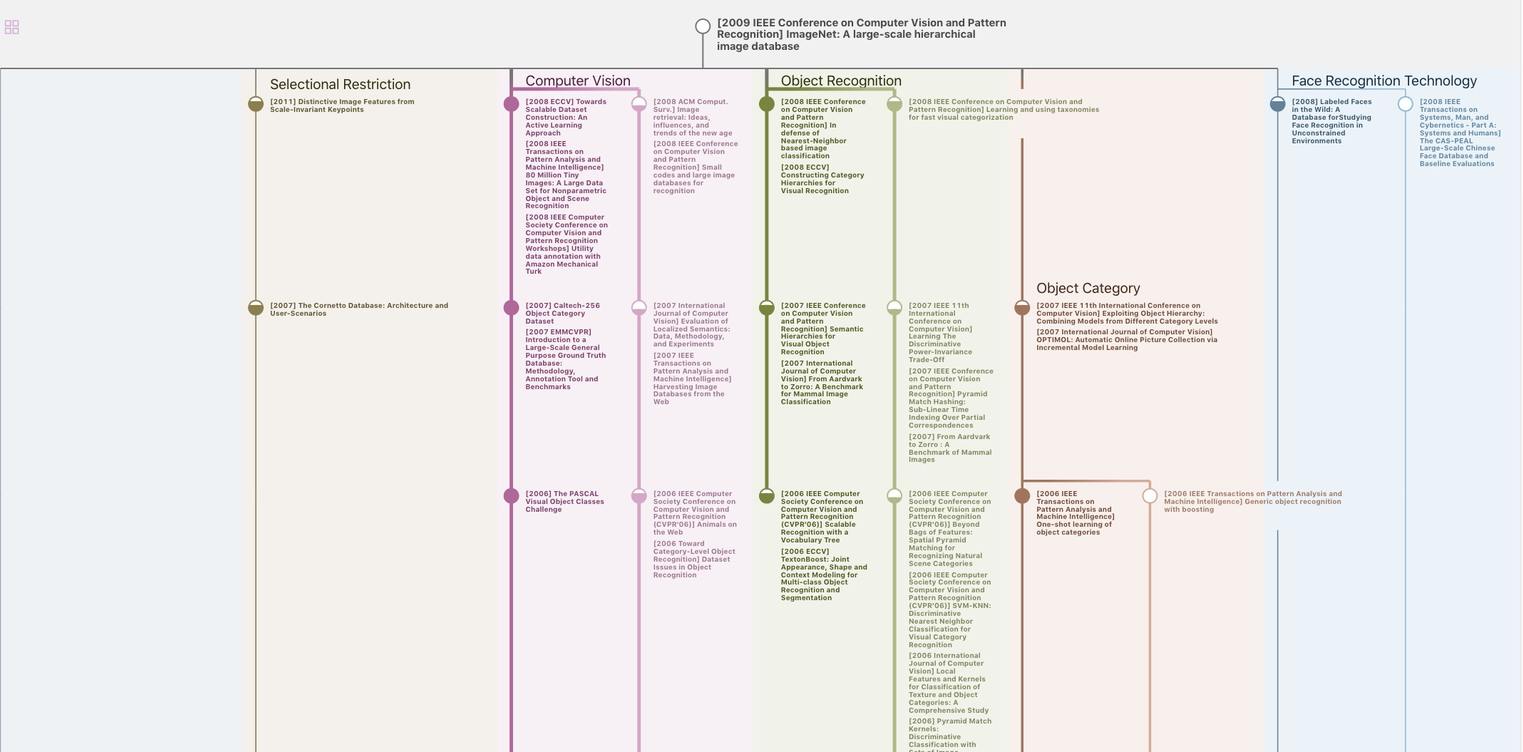
生成溯源树,研究论文发展脉络
Chat Paper
正在生成论文摘要