Automated anomaly-aware 3D segmentation of bones and cartilages in knee MR images from the Osteoarthritis Initiative
MEDICAL IMAGE ANALYSIS(2024)
摘要
In medical image analysis, automated segmentation of multi -component anatomical entities, with the possible presence of variable anomalies or pathologies, is a challenging task. In this work, we develop a multi -step approach using U -Net -based models to initially detect anomalies (bone marrow lesions, bone cysts) in the distal femur, proximal tibia and patella from 3D magnetic resonance (MR) images in individuals with varying grades of knee osteoarthritis. Subsequently, the extracted data are used for downstream tasks involving semantic segmentation of individual bone and cartilage volumes as well as bone anomalies. For anomaly detection, U -Net -based models were developed to reconstruct bone volume profiles of the femur and tibia in images via inpainting so anomalous bone regions could be replaced with close to normal appearances. The reconstruction error was used to detect bone anomalies. An anomaly -aware segmentation network, which was compared to anomaly -naive segmentation networks, was used to provide a final automated segmentation of the individual femoral, tibial and patellar bone and cartilage volumes from the knee MR images which contain a spectrum of bone anomalies. The anomaly -aware segmentation approach provided up to 58% reduction in Hausdorff distances for bone segmentations compared to the results from anomaly -naive segmentation networks. In addition, the anomaly -aware networks were able to detect bone anomalies in the MR images with greater sensitivity and specificity (area under the receiver operating characteristic curve [AUC] up to 0.896) compared to anomaly -naive segmentation networks (AUC up to 0.874).
更多查看译文
关键词
Anomaly detection,Segmentation,U-Net,Knee osteoarthritis,MRI
AI 理解论文
溯源树
样例
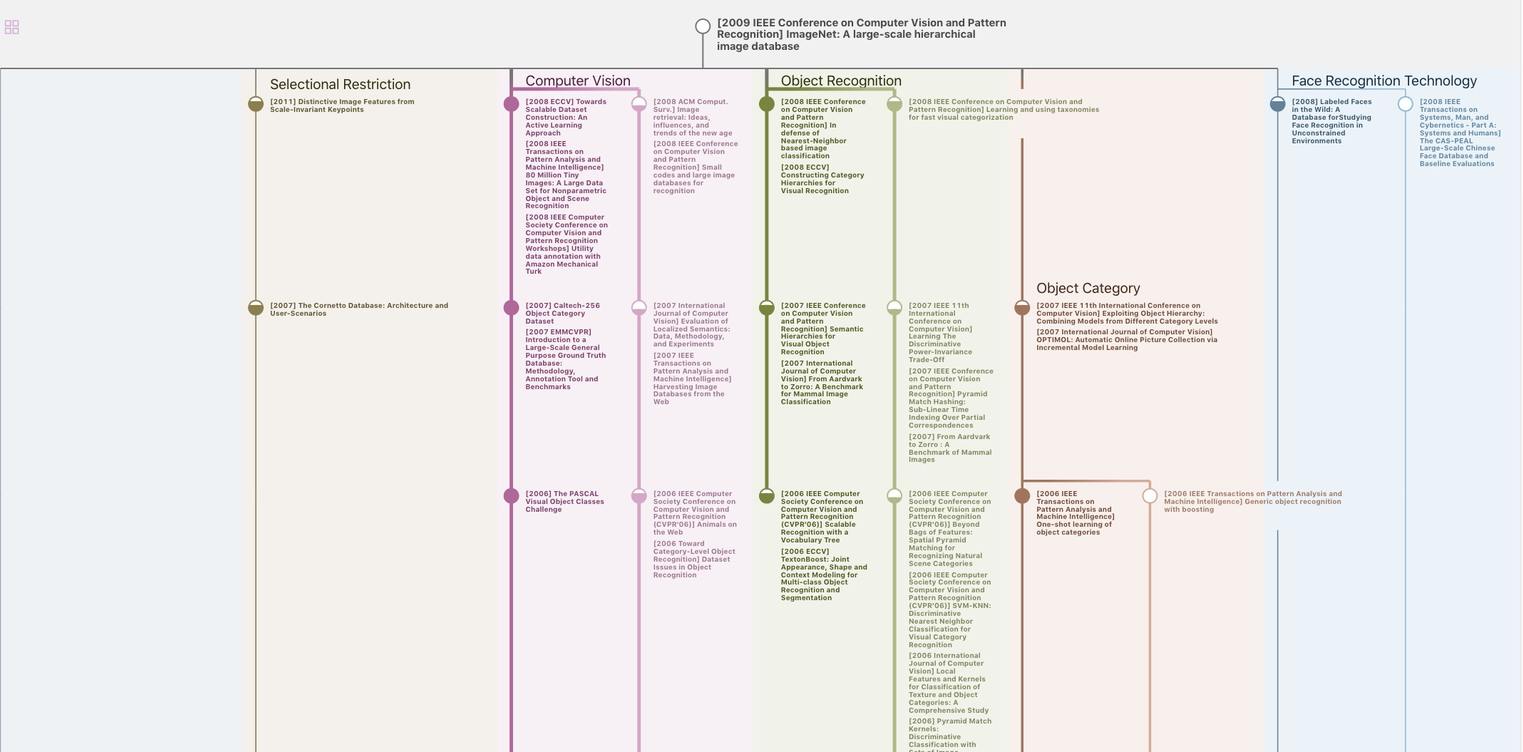
生成溯源树,研究论文发展脉络
Chat Paper
正在生成论文摘要