Collaborative learning model predictive control for repetitive tasks
arxiv(2022)
摘要
This paper presents a cloud-based learning model predictive controller that integrates three interacting components: a set of agents, which must learn to perform a finite set of tasks with the minimum possible local cost; a coordinator, which assigns the tasks to the agents; and the cloud, which stores data to facilitate the agents' learning. The tasks consist in traveling repeatedly between a set of target states while satisfying input and state constraints. In turn, the state constraints may change in time for each of the possible tasks. To deal with it, different modes of operation, which establish different restrictions, are defined. The agents' inputs are found by solving local model predictive control (MPC) problems where the terminal set and cost are defined from previous trajectories. The data collected by each agent is uploaded to the cloud and made accessible to all their peers. Likewise, similarity between tasks is exploited to accelerate the learning process. The applicability of the proposed approach is illustrated by simulation results.
更多查看译文
关键词
agents,collaborative learning model predictive control,different restrictions,finite set,interacting components,learning process,local model predictive control problems,minimum possible local cost,model predictive controller,possible tasks,repetitive tasks,state constraints,stores data,target states,terminal set
AI 理解论文
溯源树
样例
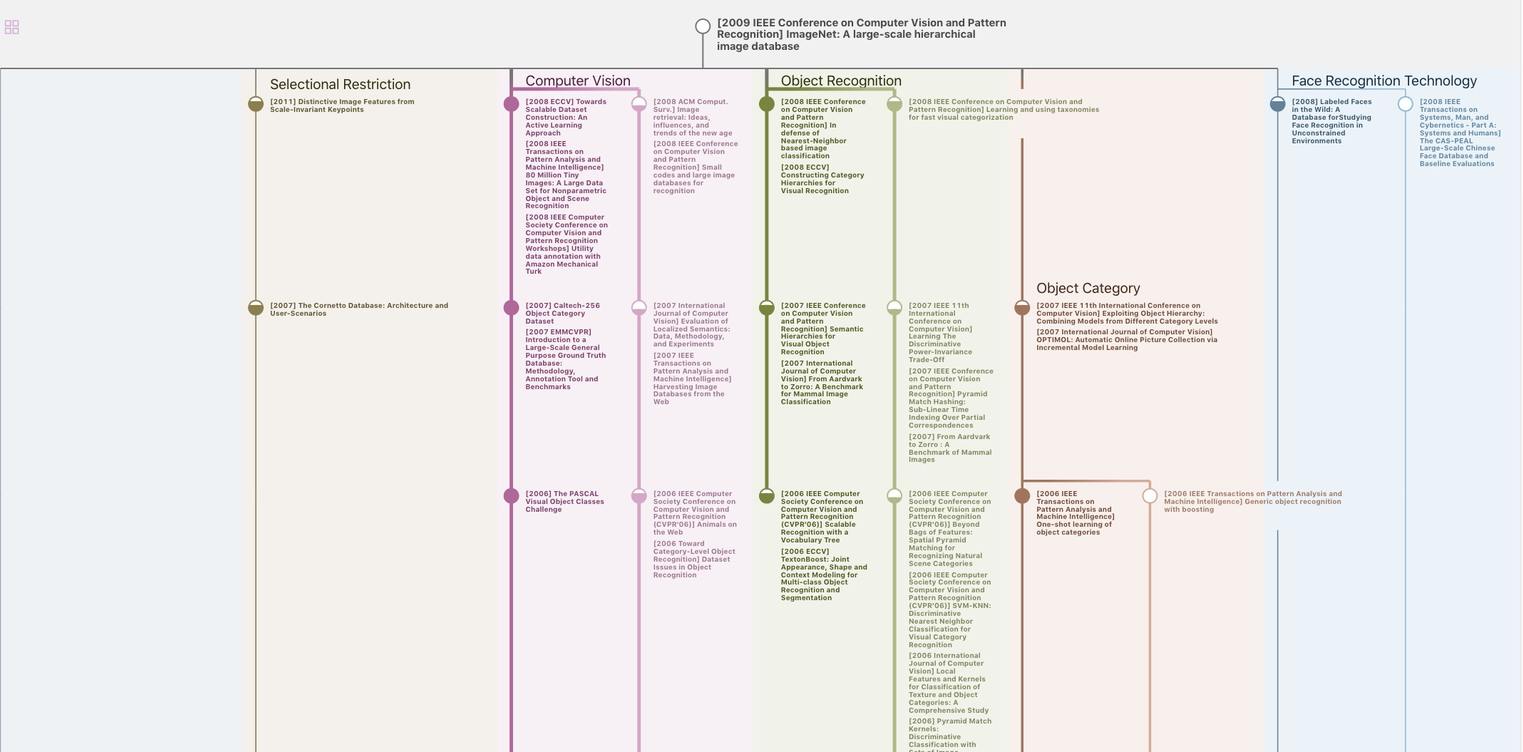
生成溯源树,研究论文发展脉络
Chat Paper
正在生成论文摘要