Convolutional neural network for high-resolution wetland mapping with open data: Variable selection and the challenges of a generalizable model
Science of The Total Environment(2023)
摘要
Landscape scale wetland conservation requires accurate, up-to-date wetland maps. The most useful approaches to creating such maps are automated, spatially generalizable, temporally repeatable, and can be applied at large spatial scales. However, mapping wetlands with predictive models is challenging due to the highly variable characteristics of wetlands in both space and time. Currently, most approaches are limited by coarse resolution, commercial data, and geographic specificity. Here, we trained a deep learning model and evaluated its ability to automatically map wetlands at landscape scale in a variety of geographies. We trained a U-Net architecture to map wetlands at 1-meter spatial resolution with the following remotely sensed covariates: multispectral data from the National Agriculture Imagery Program and the Sentinel-2 satellite system, and two LiDAR-derived datasets, intensity and geomorphons.
更多查看译文
关键词
Deep learning,Geomorphon,LiDAR,Model transferability,Remote sensing,U-Net
AI 理解论文
溯源树
样例
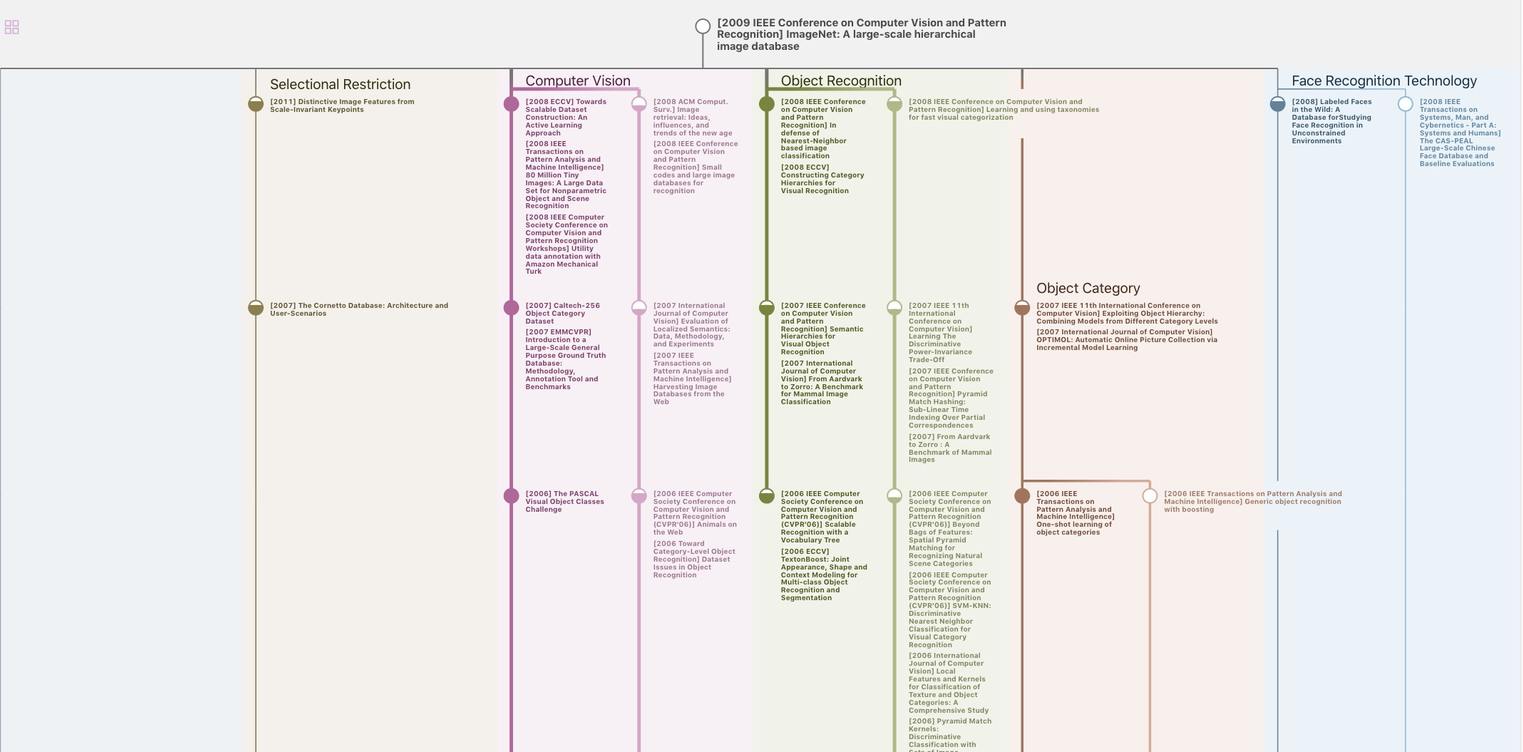
生成溯源树,研究论文发展脉络
Chat Paper
正在生成论文摘要