CerealNet: A Hybrid Deep Learning Architecture for Cereal Crop Mapping Using Sentinel-2 Time-Series
Informatics(2022)
摘要
Remote sensing-based crop mapping has continued to grow in economic importance over the last two decades. Given the ever-increasing rate of population growth and the implications of multiplying global food production, the necessity for timely, accurate, and reliable agricultural data is of the utmost importance. When it comes to ensuring high accuracy in crop maps, spectral similarities between crops represent serious limiting factors. Crops that display similar spectral responses are notorious for being nearly impossible to discriminate using classical multi-spectral imagery analysis. Chief among these crops are soft wheat, durum wheat, oats, and barley. In this paper, we propose a unique multi-input deep learning approach for cereal crop mapping, called “CerealNet”. Two time-series used as input, from the Sentinel-2 bands and NDVI (Normalized Difference Vegetation Index), were fed into separate branches of the LSTM-Conv1D (Long Short-Term Memory Convolutional Neural Networks) model to extract the temporal and spectral features necessary for the pixel-based crop mapping. The approach was evaluated using ground-truth data collected in the Gharb region (northwest of Morocco). We noted a categorical accuracy and an F1-score of 95% and 94%, respectively, with minimal confusion between the four cereal classes. CerealNet proved insensitive to sample size, as the least-represented crop, oats, had the highest F1-score. This model was compared with several state-of-the-art crop mapping classifiers and was found to outperform them. The modularity of CerealNet could possibly allow for injecting additional data such as Synthetic Aperture Radar (SAR) bands, especially when optical imagery is not available.
更多查看译文
关键词
crop mapping,CerealNet,spectral similarity,deep learning,LSTM,CNN,time-series
AI 理解论文
溯源树
样例
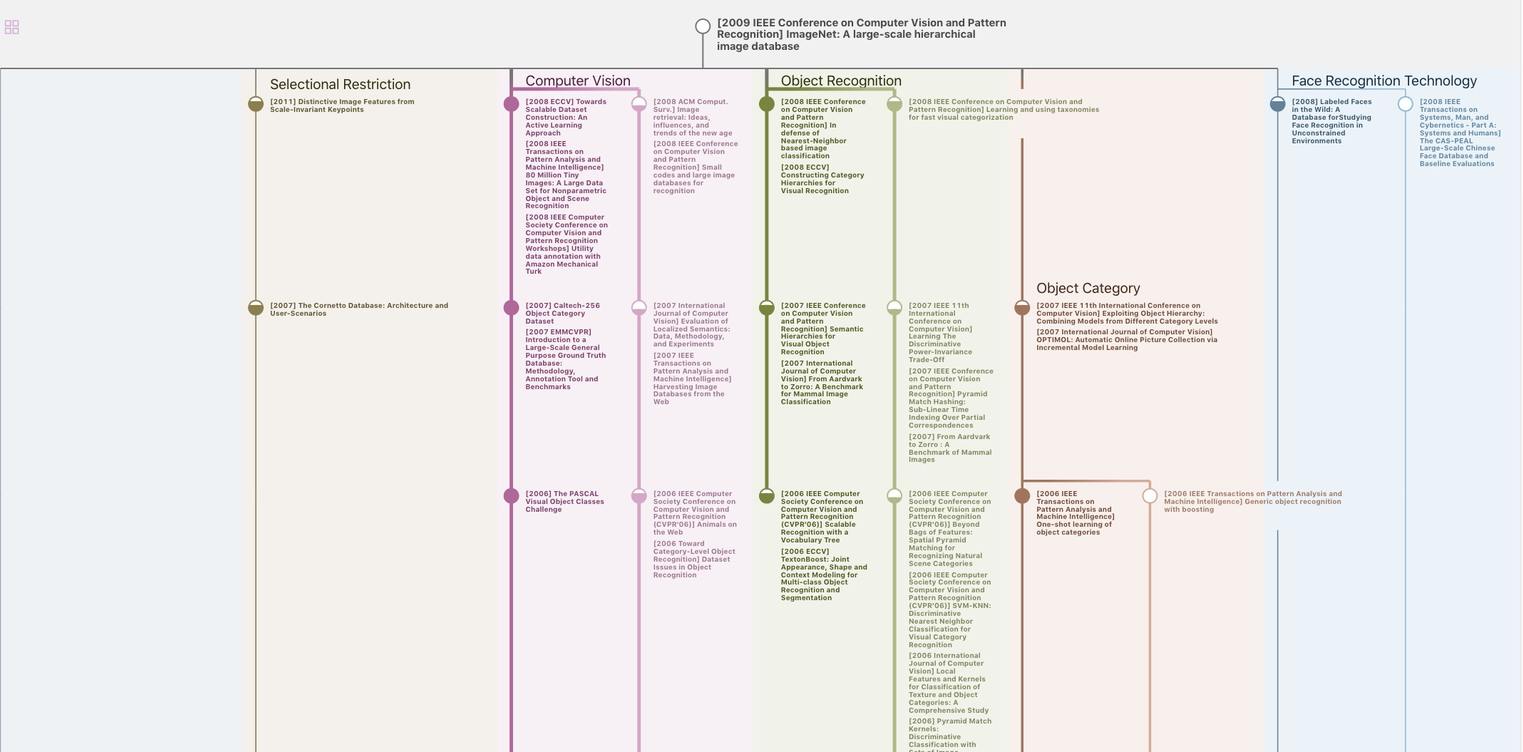
生成溯源树,研究论文发展脉络
Chat Paper
正在生成论文摘要