Interpretable deep learning translation of GWAS and multi-omics findings to identify pathobiology and drug repurposing in Alzheimer’s disease
Cell Reports(2022)
摘要
Translating human genetic findings (genome-wide association studies [GWAS]) to pathobiology and therapeutic discovery remains a major challenge for Alzheimer’s disease (AD). We present a network topology-based deep learning framework to identify disease-associated genes (NETTAG). We leverage non-coding GWAS loci effects on quantitative trait loci, enhancers and CpG islands, promoter regions, open chromatin, and promoter flanking regions under the protein-protein interactome. Via NETTAG, we identified 156 AD-risk genes enriched in druggable targets. Combining network-based prediction and retrospective case-control observations with 10 million individuals, we identified that usage of four drugs (ibuprofen, gemfibrozil, cholecalciferol, and ceftriaxone) is associated with reduced likelihood of AD incidence. Gemfibrozil (an approved lipid regulator) is significantly associated with 43% reduced risk of AD compared with simvastatin using an active-comparator design (95% confidence interval 0.51–0.63, p < 0.0001). In summary, NETTAG offers a deep learning methodology that utilizes GWAS and multi-genomic findings to identify pathobiology and drug repurposing in AD.
更多查看译文
关键词
Alzheimer’s disease,AD,deep learning,drug repurposing,drug target,electronic health record,EHR,genome-wide association studies,GWAS,gemfibrozil,multi-omics,pathobiology,protein-protein Interactome
AI 理解论文
溯源树
样例
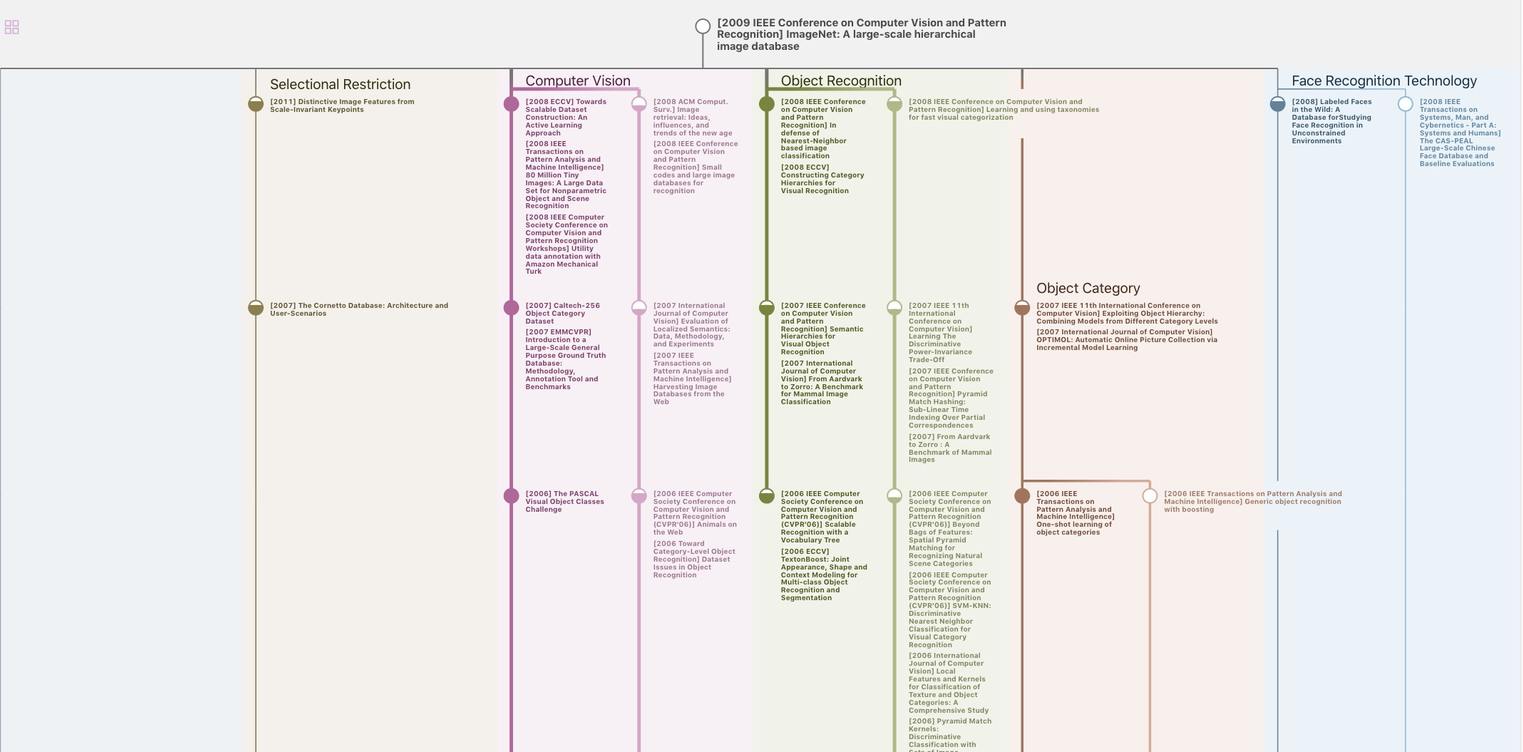
生成溯源树,研究论文发展脉络
Chat Paper
正在生成论文摘要