Deep Learning Allows Assessment of Risk of Metastatic Relapse from Invasive Breast Cancer Histological Slides
biorxiv(2022)
摘要
Background : Correctly classifying early estrogen receptor-positive and HER2-negative (ER+/HER2) breast cancer (EBC) cases allows to propose an adapted adjuvant systemic treatment strategy. We developed a new AI-based tool to assess the risk of distant relapse at 5 years for ER+/HER2- EBC patients from pathological slides.
Patients and Methods : The discovery dataset (GrandTMA) included 1429 ER+/HER2- EBC patients, with long-term follow-up and an available hematoxylin-eosin and saffron (HES) whole slide image (WSI). A Deep Learning (DL) network was trained to predict metastasis free survival (MFS) at five years, based on the HES WSI only (termed RlapsRisk). A combined score was then built using RlapsRisk and well established prognostic factors. A threshold corresponding to a probability of MFS event of 5% at 5 years was applied to dichotomize patients into low or high-risk groups. The external validation, as well as assessment of the additional prognosis value of the DL model beyond standard clinico-pathologic factors were carried out on an independent, prospective cohort (CANTO, [NCT01993498][1]) including 889 HES WSI of ER+/HER2- EBC patients.
Results :RlapsRisk was an independent prognostic factor of MFS in multivariable analysis adjusted for established clinico-pathological factors (p<0.005 in GrandTMA and CANTO). Combining RlapsRisk score and the clinico-pathological factors improved the prognostic discrimination as compared to the clinico-pathological factors alone (increment of c-index in the validation set 0.80 versus 0.76, +0.04, p-value < 0.005). After dichotomization, the Combined Model showed a higher cumulative sensitivity on the entire population (0.76 vs 0.61) for an equal dynamic specificity (0.76) in comparison with the clinical score alone.
Conclusions :Our deep learning model developed on digitized HES slides provided additional prognostic information as compared to current clinico-pathological factors and has the potential of valuably informing the decision making process in the adjuvant setting when combined with current clinico-pathological factors.
### Competing Interest Statement
Suzette Delaloge reports grants and non-financial support from Pfizer, grants from Novartis, grants and non-financial support from AstraZeneca, grants from Roche Genentech, grants from Lilly, grants from Orion, grants from Amgen, grants from Sanofi, grants from Genomic Health, grants from Servier, grants from MSD, grants from BMS, grants from Pierre Fabre, grants from Exact Sciences, grants from Besins, grants from European Commission grants, grants from French government grants, grants from Fondation ARC grants, grants from Taiho, grants from Elsan, outside the submitted work. Fabrice Andre declares institutional financial interests, research grants with Novartis, Pfizer, Astra Zeneca, Eli Lilly, Daiichi, Roche.
[1]: /lookup/external-ref?link_type=CLINTRIALGOV&access_num=NCT01993498&atom=%2Fbiorxiv%2Fearly%2F2022%2F11%2F29%2F2022.11.28.518158.atom
更多查看译文
关键词
breast cancer,deep learning,metastatic relapse
AI 理解论文
溯源树
样例
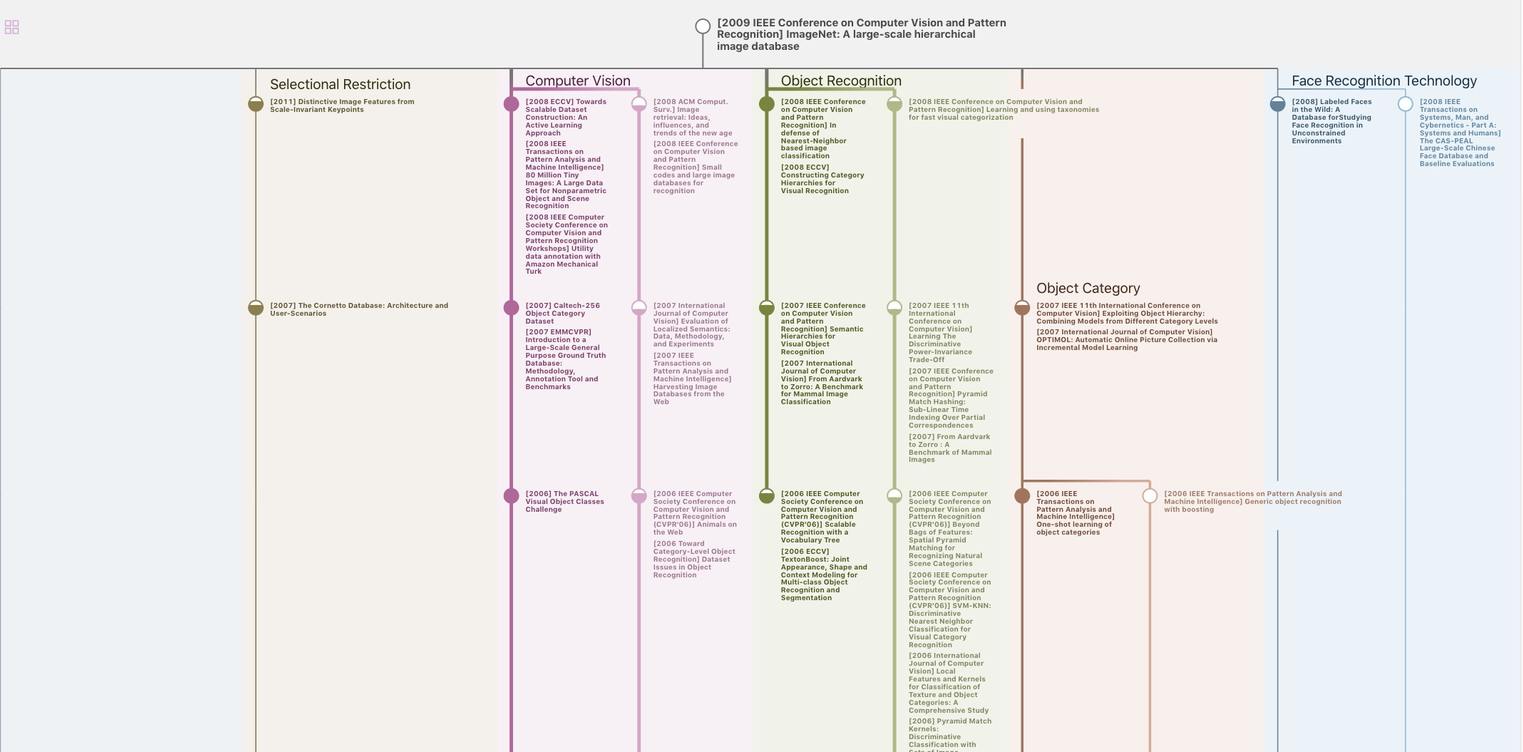
生成溯源树,研究论文发展脉络
Chat Paper
正在生成论文摘要