Chaining Simultaneous Thoughts for Numerical Reasoning
arxiv(2022)
摘要
Given that rich information is hidden behind ubiquitous numbers in text, numerical reasoning over text should be an essential skill of AI systems. To derive precise equations to solve numerical reasoning problems, previous work focused on modeling the structures of equations, and has proposed various structured decoders. Though structure modeling proves to be effective, these structured decoders construct a single equation in a pre-defined autoregressive order, potentially placing an unnecessary restriction on how a model should grasp the reasoning process. Intuitively, humans may have numerous pieces of thoughts popping up in no pre-defined order; thoughts are not limited to the problem at hand, and can even be concerned with other related problems. By comparing diverse thoughts and chaining relevant pieces, humans are less prone to errors. In this paper, we take this inspiration and propose CANTOR, a numerical reasoner that models reasoning steps using a directed acyclic graph where we produce diverse reasoning steps simultaneously without pre-defined decoding dependencies, and compare and chain relevant ones to reach a solution. Extensive experiments demonstrated the effectiveness of CANTOR under both fully-supervised and weakly-supervised settings.
更多查看译文
关键词
simultaneous thoughts,reasoning
AI 理解论文
溯源树
样例
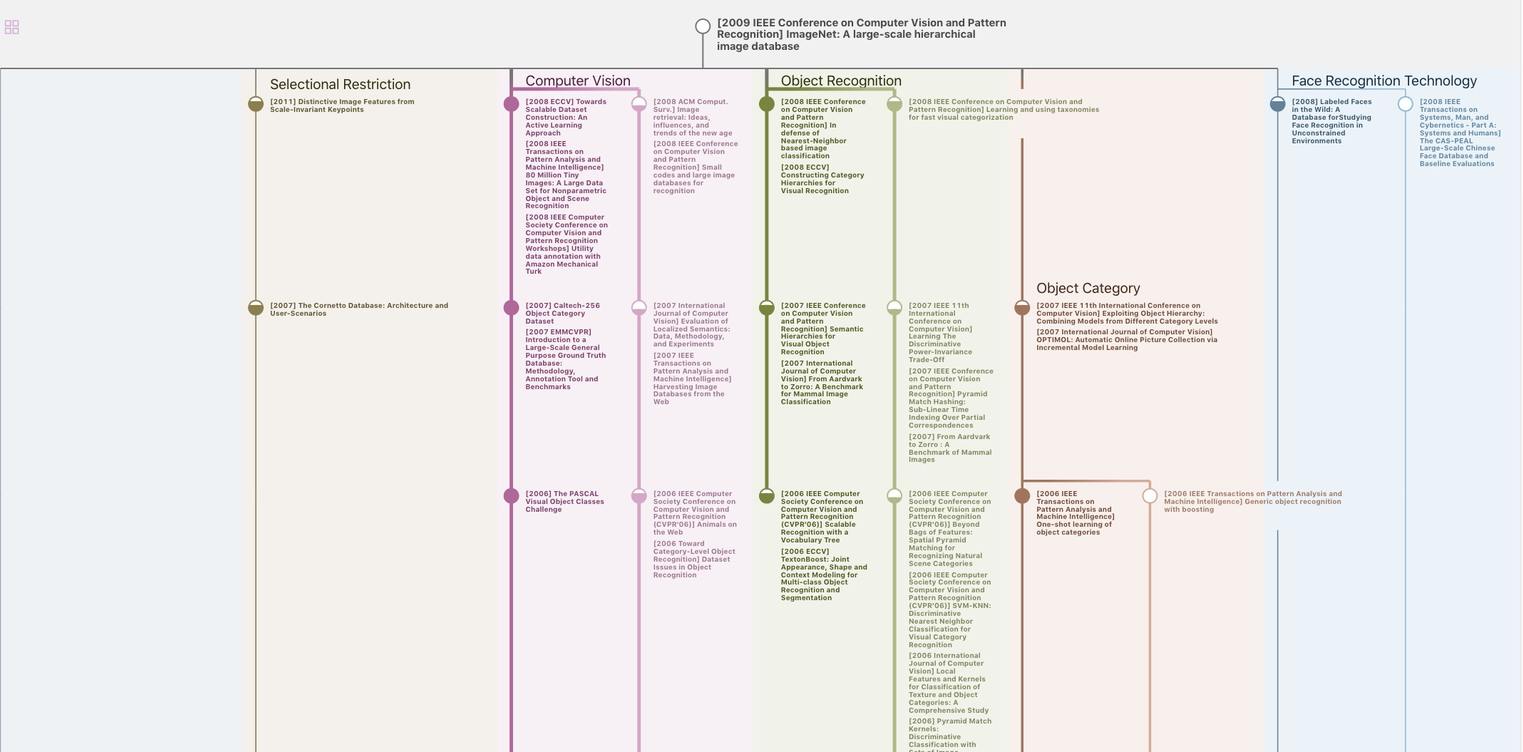
生成溯源树,研究论文发展脉络
Chat Paper
正在生成论文摘要