AutoCAD: Automatically Generating Counterfactuals for Mitigating Shortcut Learning
EMNLP (Findings)(2022)
摘要
Recent studies have shown the impressive efficacy of counterfactually augmented data (CAD) for reducing NLU models' reliance on spurious features and improving their generalizability. However, current methods still heavily rely on human efforts or task-specific designs to generate counterfactuals, thereby impeding CAD's applicability to a broad range of NLU tasks. In this paper, we present AutoCAD, a fully automatic and task-agnostic CAD generation framework. AutoCAD first leverages a classifier to unsupervisedly identify rationales as spans to be intervened, which disentangles spurious and causal features. Then, AutoCAD performs controllable generation enhanced by unlikelihood training to produce diverse counterfactuals. Extensive evaluations on multiple out-of-domain and challenge benchmarks demonstrate that AutoCAD consistently and significantly boosts the out-of-distribution performance of powerful pre-trained models across different NLU tasks, which is comparable or even better than previous state-of-the-art human-in-the-loop or task-specific CAD methods. The code is publicly available at https://github.com/thu-coai/AutoCAD.
更多查看译文
关键词
shortcut learning,automatically generating counterfactuals
AI 理解论文
溯源树
样例
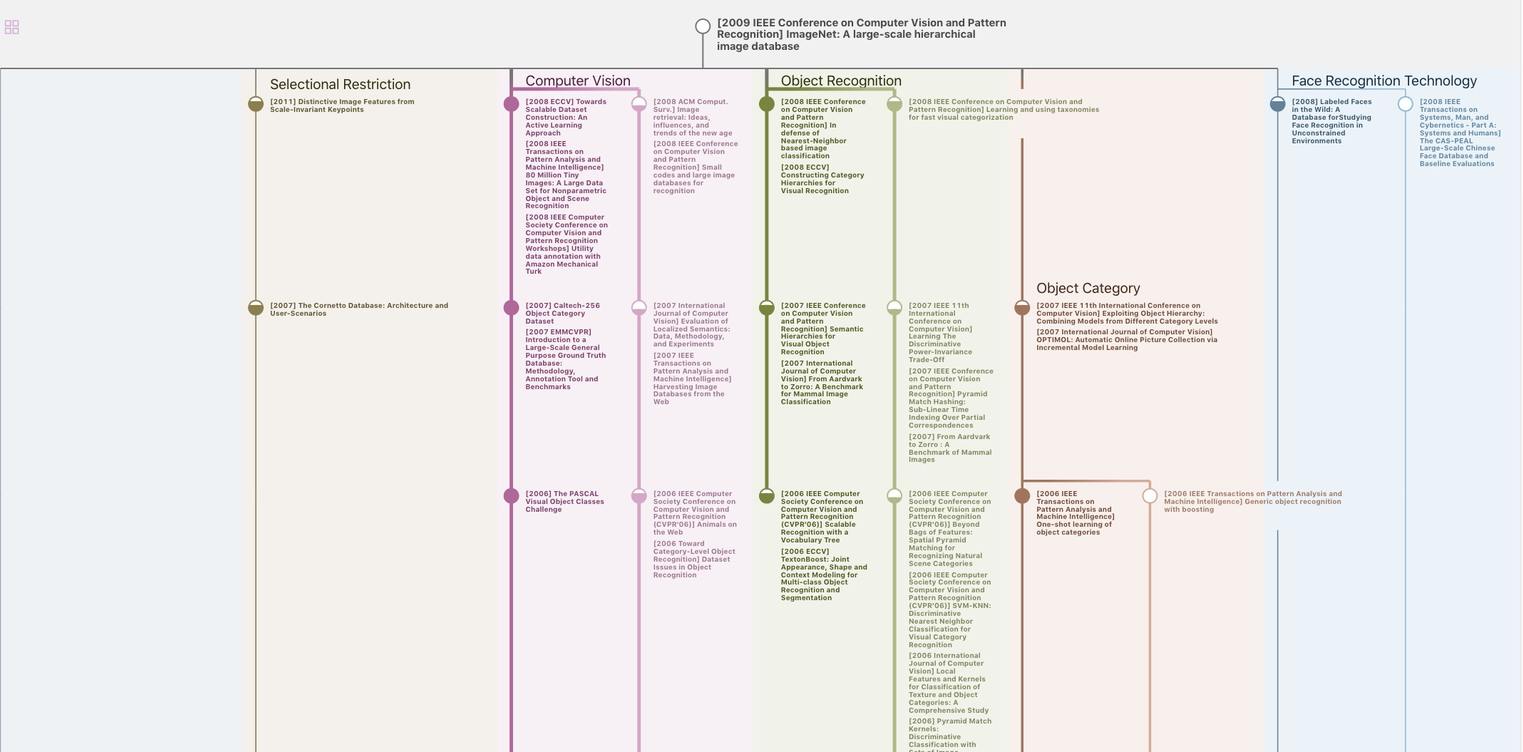
生成溯源树,研究论文发展脉络
Chat Paper
正在生成论文摘要