Towards Better Evaluation of Topic Model Quality
2022 32nd Conference of Open Innovations Association (FRUCT)(2022)
摘要
Topic modelling is a popular unsupervised method for text corpora processing to obtain interpreted knowledge of the data. However, there is an automatic quality measurement gap between existing metrics, human evaluation and performance on the target tasks. That is a big challenge for automatic hyperparameter tuning methods as they heavily rely on the output signal to define the optimization direction. Currently, this process of evaluating the effectiveness of the topic model faces a number of difficulties and keeps being a labour-intensive routine performed manually due to the absence of a universal metric that may show strong correspondence with human assessment. The development of a quality metric that may satisfy this condition is essential to provide valuable feedback for the optimization algorithm when working with flexible and complex models, such as models based on additive regularisation or neural networks. To address the quality measurement gap, we performed an experimental study of existing scores on a specially created dataset containing topic models for several different text corpora in two languages accompanied by evaluated existing metrics and scores obtained from human assessment. The study results show how the situation with automatic quality estimation may be improved and pave the way to metrics learning with ensembles of machine learning algorithms.
更多查看译文
关键词
topic modeling,quality function,optimization,artm
AI 理解论文
溯源树
样例
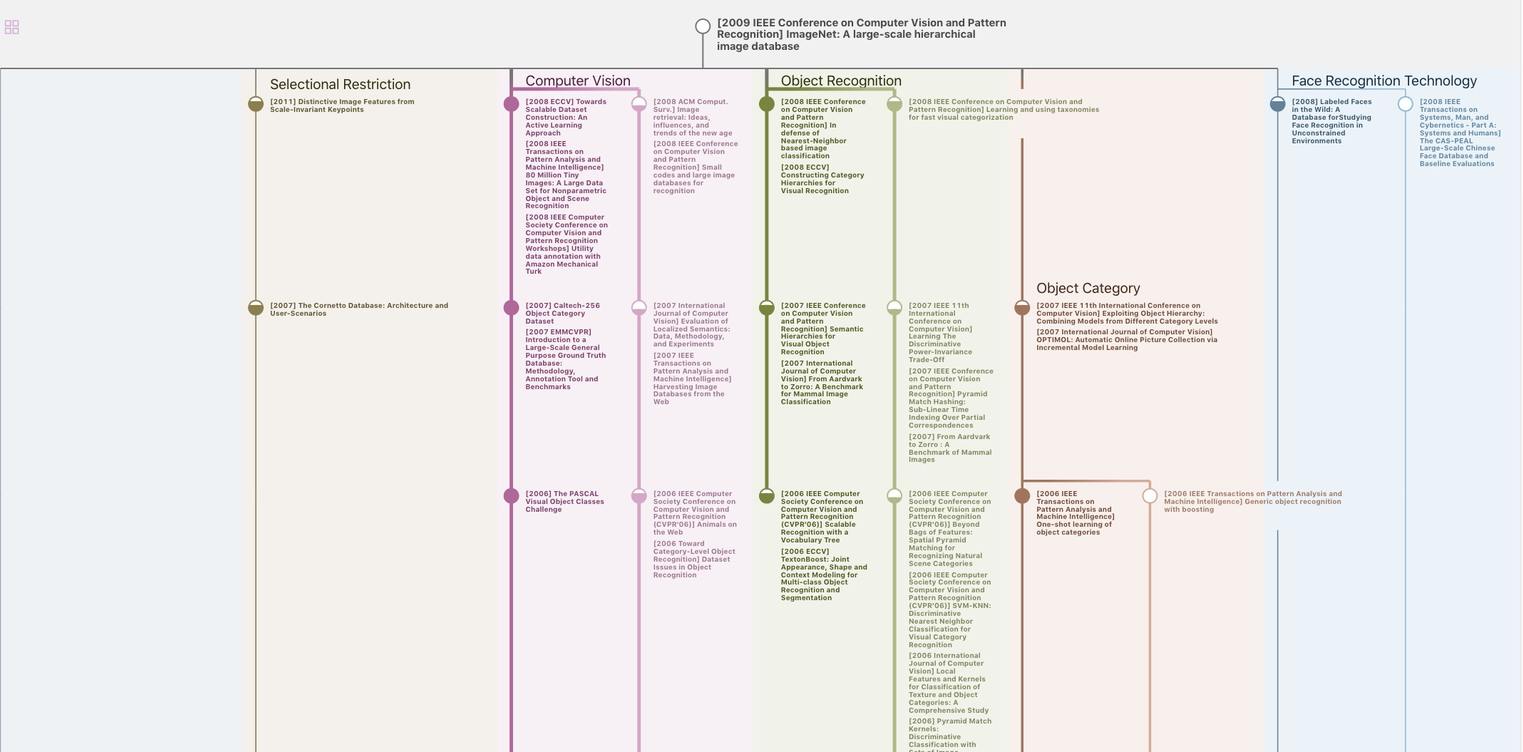
生成溯源树,研究论文发展脉络
Chat Paper
正在生成论文摘要