Robust co-teaching learning with consistency-based noisy label correction for medical image classification
International journal of computer assisted radiology and surgery(2022)
摘要
Purpose Deep neural networks (DNNs) have made great achievements in computer-aided diagnostic systems, but the success highly depends on massive data with high-quality labels. However, for many medical image datasets, a considerable number of noisy labels are introduced by inter- and intra-observer variability, thus hampering DNNs’ performance. To address this problem, a robust noisy label correction method with the co-teaching learning paradigm is proposed. Methods The proposed method aims to reduce the effect of noisy labels by correcting or removing them. It consists of two modules. An adaptive noise rate estimation module is employed to calculate the dataset’s noise rate, which is helpful to detect noisy labels but is usually unavailable in clinical applications. A consistency-based noisy label correction module aims to detect noisy labels and correct them to reduce the disturbance from noisy labels and exploit useful information in data. Results Experiments are conducted on the public skin lesion dataset ISIC-2017, ISIC-2019, and our constructed thyroid ultrasound image dataset. The results demonstrate that the proposed method outperforms other noisy label learning methods in medical image classification tasks. It is also evaluated on the natural image dataset CIFAR-10 to show its generalization. Conclusion This paper proposes a noisy label correction method to handle noisy labels in medical image datasets. Experimental results show that it can self-adapt to different datasets and efficiently correct noisy labels, which is suitable for medical image classification.
更多查看译文
关键词
Noisy label correction,Co-teaching,Noise rate estimation,Medical image classification
AI 理解论文
溯源树
样例
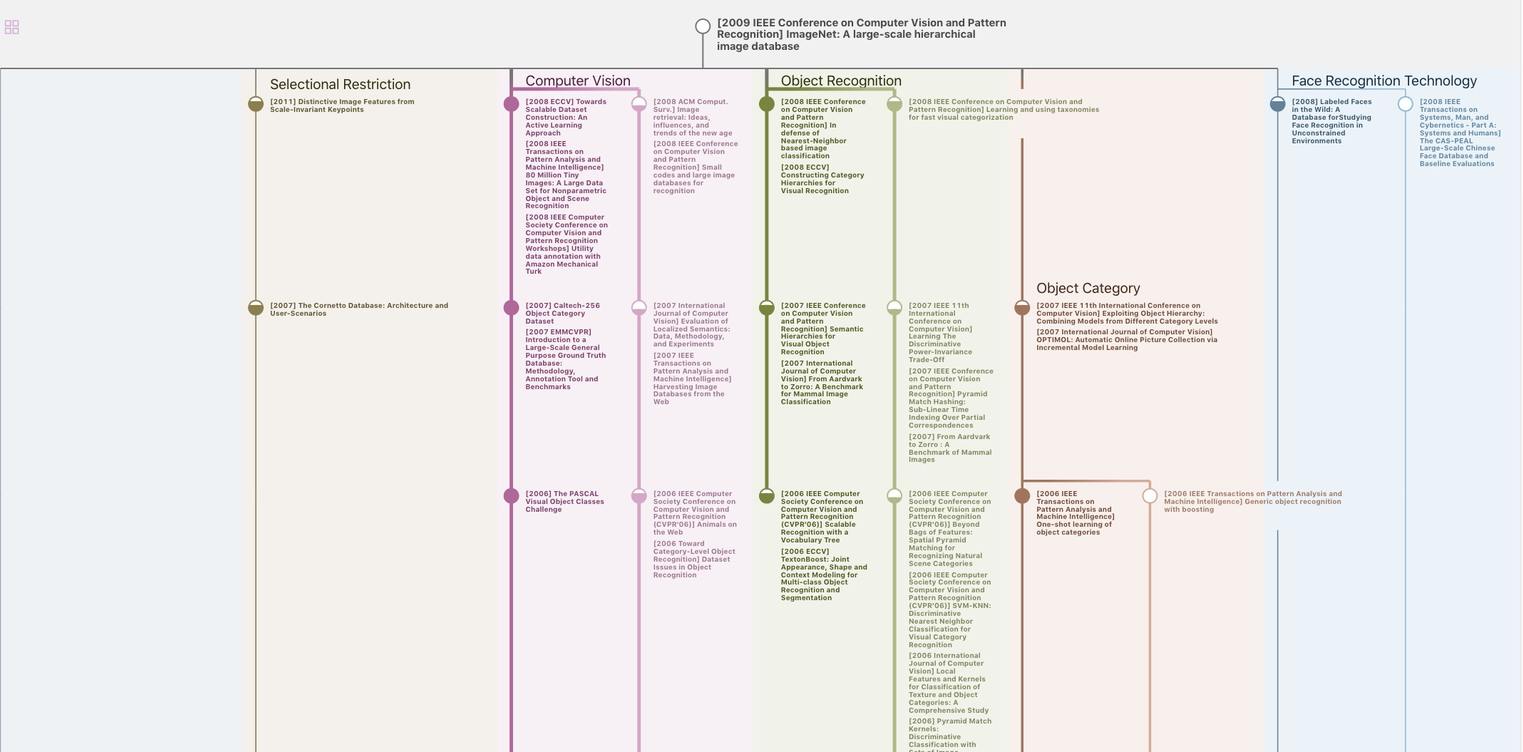
生成溯源树,研究论文发展脉络
Chat Paper
正在生成论文摘要