Is Conditional Generative Modeling all you need for Decision-Making?
ICLR(2022)
摘要
Recent improvements in conditional generative modeling have made it possible to generate high-quality images from language descriptions alone. We investigate whether these methods can directly address the problem of sequential decision-making. We view decision-making not through the lens of reinforcement learning (RL), but rather through conditional generative modeling. To our surprise, we find that our formulation leads to policies that can outperform existing offline RL approaches across standard benchmarks. By modeling a policy as a return-conditional diffusion model, we illustrate how we may circumvent the need for dynamic programming and subsequently eliminate many of the complexities that come with traditional offline RL. We further demonstrate the advantages of modeling policies as conditional diffusion models by considering two other conditioning variables: constraints and skills. Conditioning on a single constraint or skill during training leads to behaviors at test-time that can satisfy several constraints together or demonstrate a composition of skills. Our results illustrate that conditional generative modeling is a powerful tool for decision-making.
更多查看译文
关键词
Offline Reinforcement Learning,Conditional Generative Modeling,Sequential Decision Making,Diffusion Models
AI 理解论文
溯源树
样例
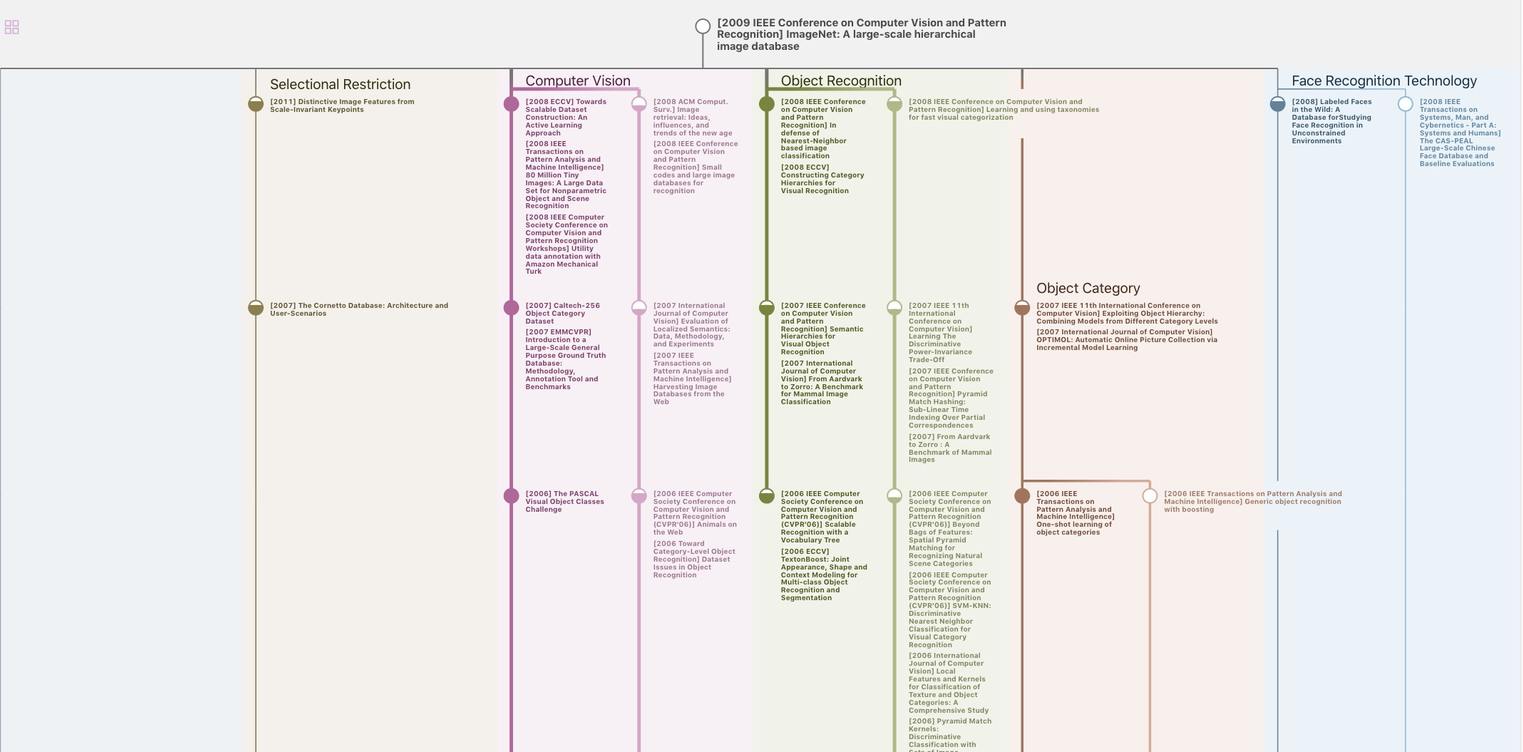
生成溯源树,研究论文发展脉络
Chat Paper
正在生成论文摘要