Zero-order optimization for Gaussian process-based model predictive control
EUROPEAN JOURNAL OF CONTROL(2023)
摘要
By enabling constraint-aware online model adaptation, model predictive control using Gaussian process (GP) regression has exhibited impressive performance in real-world applications and received considerable attention in the learning-based control community. Yet, solving the resulting optimal control problem in real-time generally remains a major challenge, due to (i) the increased number of augmented states in the optimization problem, as well as (ii) computationally expensive evaluations of the posterior mean and covariance and their respective derivatives. To tackle these challenges, we employ (i) a tailored Jacobian approximation in a sequential quadratic programming (SQP) approach and combine it with (ii) a parallelizable GP inference and automatic differentiation framework. Reducing the numerical complexity with respect to the state dimension nx for each SQP iteration from O(nx6 ) to O(nx3 ), and accelerating GP evaluations on a graphical processing unit, the proposed algorithm computes suboptimal, yet feasible, solutions at drastically reduced computation times and exhibits favorable local convergence properties. Numerical experiments verify the scaling properties and investigate the runtime distribution across different parts of the algorithm.(c) 2023 The Author(s). Published by Elsevier Ltd on behalf of European Control Association. This is an open access article under the CC BY license ( http://creativecommons.org/licenses/by/4.0/ )
更多查看译文
关键词
Real-time model predictive control,Nonlinear optimization,Inexact sequential quadratic programming
AI 理解论文
溯源树
样例
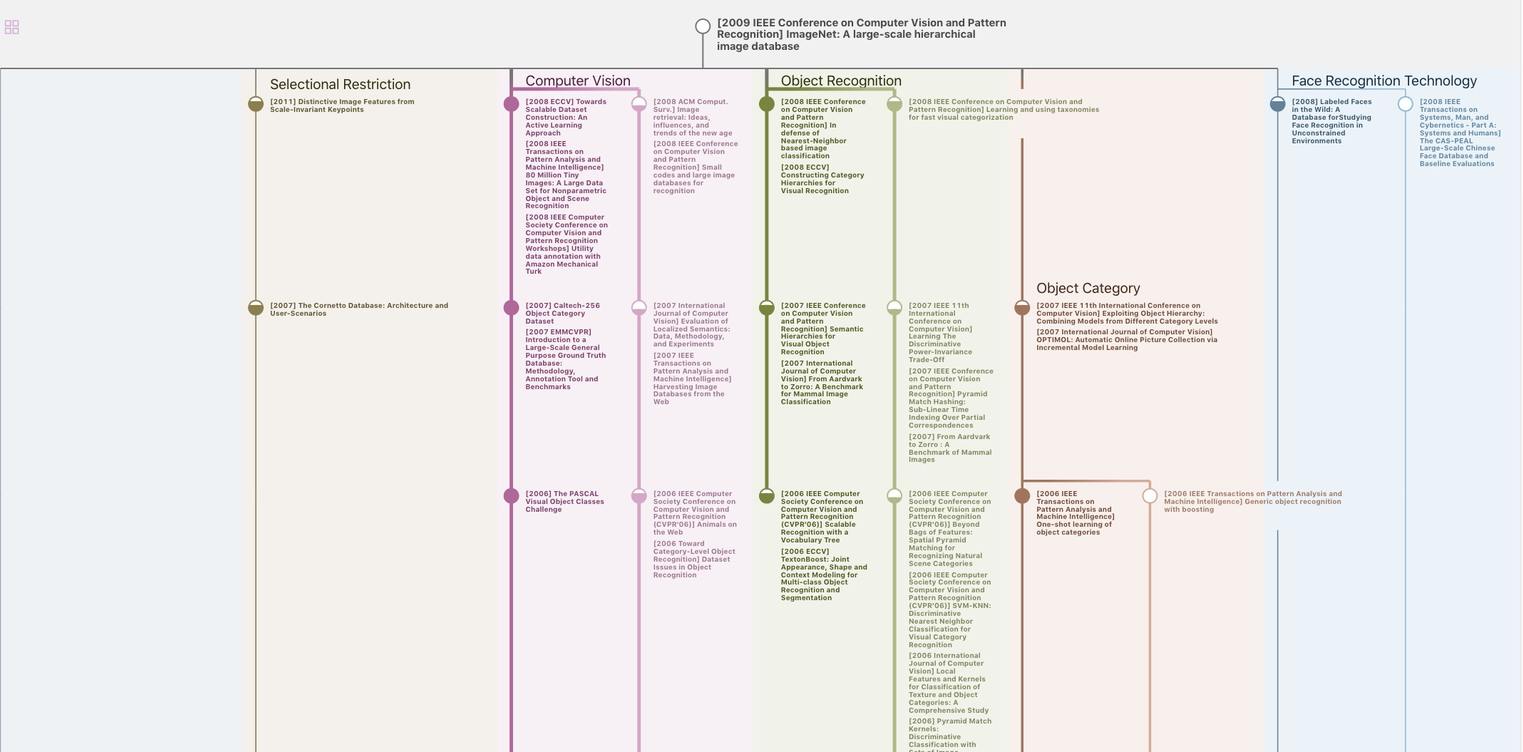
生成溯源树,研究论文发展脉络
Chat Paper
正在生成论文摘要