Unsupervised Superpixel Generation using Edge-Sparse Embedding
arxiv(2022)
摘要
Partitioning an image into superpixels based on the similarity of pixels with respect to features such as colour or spatial location can significantly reduce data complexity and improve subsequent image processing tasks. Initial algorithms for unsupervised superpixel generation solely relied on local cues without prioritizing significant edges over arbitrary ones. On the other hand, more recent methods based on unsupervised deep learning either fail to properly address the trade-off between superpixel edge adherence and compactness or lack control over the generated number of superpixels. By using random images with strong spatial correlation as input, \ie, blurred noise images, in a non-convolutional image decoder we can reduce the expected number of contrasts and enforce smooth, connected edges in the reconstructed image. We generate edge-sparse pixel embeddings by encoding additional spatial information into the piece-wise smooth activation maps from the decoder's last hidden layer and use a standard clustering algorithm to extract high quality superpixels. Our proposed method reaches state-of-the-art performance on the BSDS500, PASCAL-Context and a microscopy dataset.
更多查看译文
关键词
edge-sparse
AI 理解论文
溯源树
样例
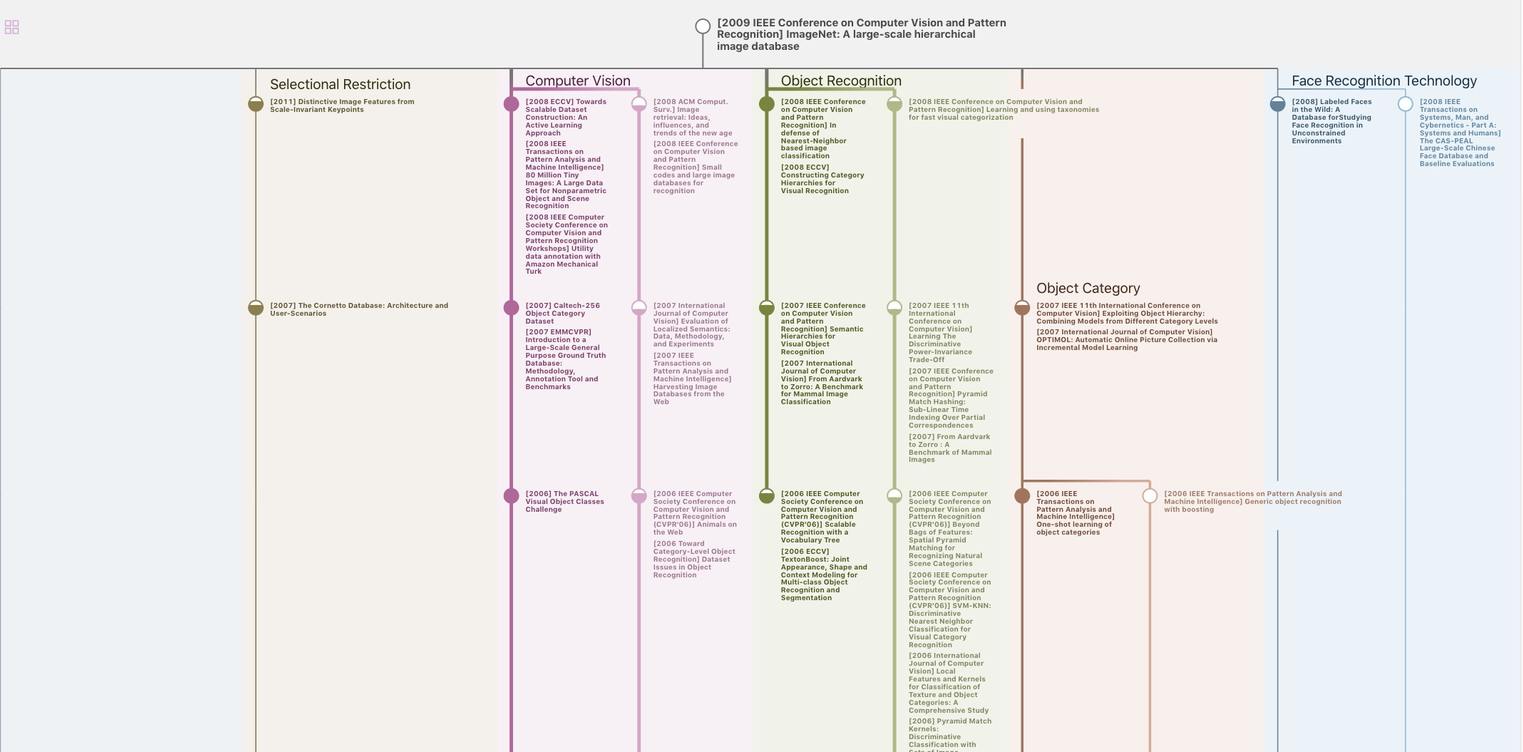
生成溯源树,研究论文发展脉络
Chat Paper
正在生成论文摘要