Learning Integrable Dynamics with Action-Angle Networks
arxiv(2022)
摘要
Machine learning has become increasingly popular for efficiently modelling the dynamics of complex physical systems, demonstrating a capability to learn effective models for dynamics which ignore redundant degrees of freedom. Learned simulators typically predict the evolution of the system in a step-by-step manner with numerical integration techniques. However, such models often suffer from instability over long roll-outs due to the accumulation of both estimation and integration error at each prediction step. Here, we propose an alternative construction for learned physical simulators that are inspired by the concept of action-angle coordinates from classical mechanics for describing integrable systems. We propose Action-Angle Networks, which learn a nonlinear transformation from input coordinates to the action-angle space, where evolution of the system is linear. Unlike traditional learned simulators, Action-Angle Networks do not employ any higher-order numerical integration methods, making them extremely efficient at modelling the dynamics of integrable physical systems.
更多查看译文
关键词
integrable dynamics,networks,learning,action-angle
AI 理解论文
溯源树
样例
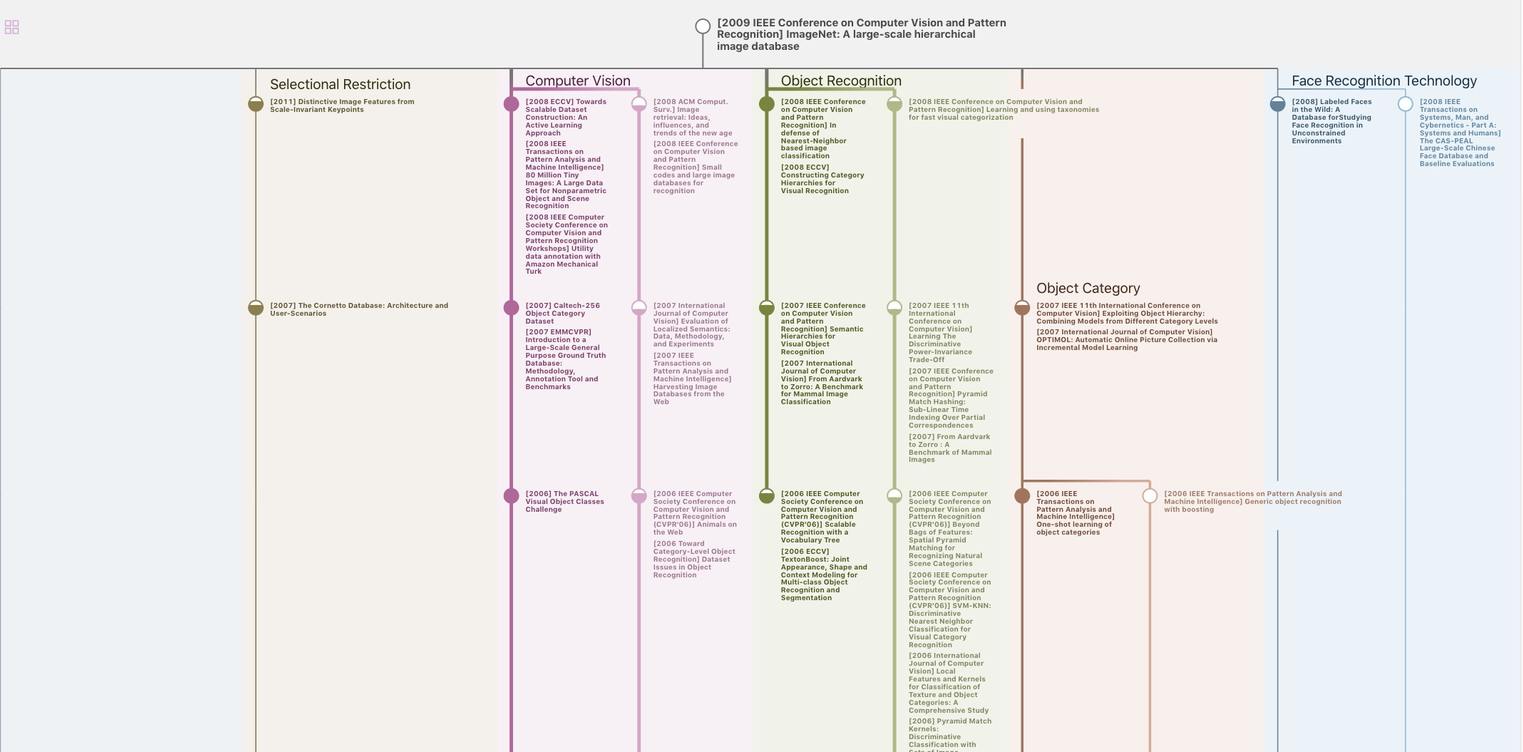
生成溯源树,研究论文发展脉络
Chat Paper
正在生成论文摘要