Non-inferiority of Deep Learning Acute Ischemic Stroke Segmentation on Non-Contrast CT Compared to Expert Neuroradiologists
arxiv(2023)
摘要
Purpose: To show a deep learning model that segments acute ischemic stroke on NCCT at a level comparable to neuroradiologists. Materials and Methods: This included 227 Head NCCT examinations from 200 patients enrolled in the multi-center DEFUSE 3 trial. Three experienced neuroradiologists independently segmented the acute infarct on each scan. The neuroradiologists were divided into training experts (A) and test experts (B and C). The dataset was randomly split, by patient, into 5 folds with training and validation cases. A 3D deep Convolutional Neural Network (CNN) architecture was trained and optimized to predict the segmentations of expert A from NCCT. The performance of the model was assessed using a set of volume, overlap, and distance metrics. The optimized model was compared to the test experts B and C. We used a one-sided Wilcoxon signed-rank test to test for the non-inferiority of the model-expert compared to the inter-expert agreement. Results: The model-expert agreement was non-inferior to the inter-expert agreement as evaluated with a paired one-sided test procedure for differences in medians with lower boundaries of 10%, 2ml, and 5mm, p < 0.05, n=160. Conclusion: The 3d CNN trained on one neuroradiologist generalizes to acute ischemic stroke segmentation on NCCT of other neuroradiologists.
更多查看译文
AI 理解论文
溯源树
样例
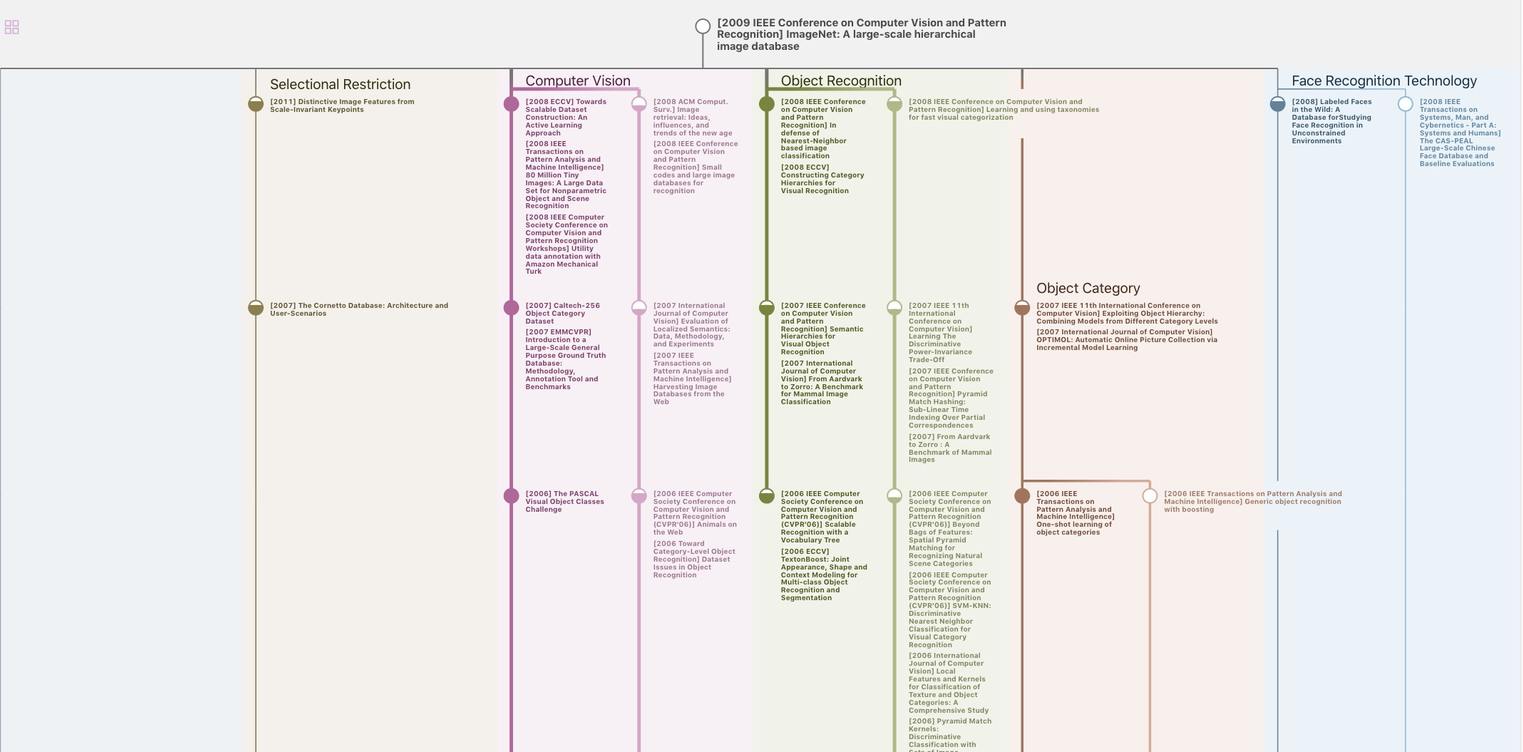
生成溯源树,研究论文发展脉络
Chat Paper
正在生成论文摘要