Higher Order Knowledge Transfer for Dynamic Community Detection With Great Changes
IEEE TRANSACTIONS ON EVOLUTIONARY COMPUTATION(2024)
摘要
Network structure evolves with time in the real world, and the discovery of changing communities in dynamic networks is an important research topic that poses challenging tasks. Most existing methods assume that no significant change occurs; namely, the difference between adjacent snapshots is slight. However, great change exists in the real world usually. The great change in the network will result in the community detection algorithms are difficulty obtaining valuable information from the previous snapshot, leading to negative transfer for the next time steps. This article focuses on dynamic community detection with substantial changes by integrating higher order knowledge from the previous snapshots to aid the subsequent snapshots. Moreover, to improve search efficiency, a higher order knowledge transfer strategy is designed to determine first-order and higher order knowledge by detecting the similarity of the adjacency matrix of snapshots. In this way, our proposal can keep the advantages of previous community detection results and transfer them to the next task. We conduct the experiments on four real-world networks, including the networks with great or minor changes. Experimental results in the low-similarity datasets demonstrate that higher order knowledge is more valuable than first-order knowledge when the network changes significantly and keeps the advantage even if handling the high-similarity datasets. Our proposal can also guide other dynamic optimization problems with great changes.
更多查看译文
关键词
Heuristic algorithms,Optimization,Knowledge transfer,Proposals,Linear programming,Task analysis,Evolutionary computation,Dynamic community detection (DCD),evolutionary algorithm (EA),multiobjective optimization,transfer learning
AI 理解论文
溯源树
样例
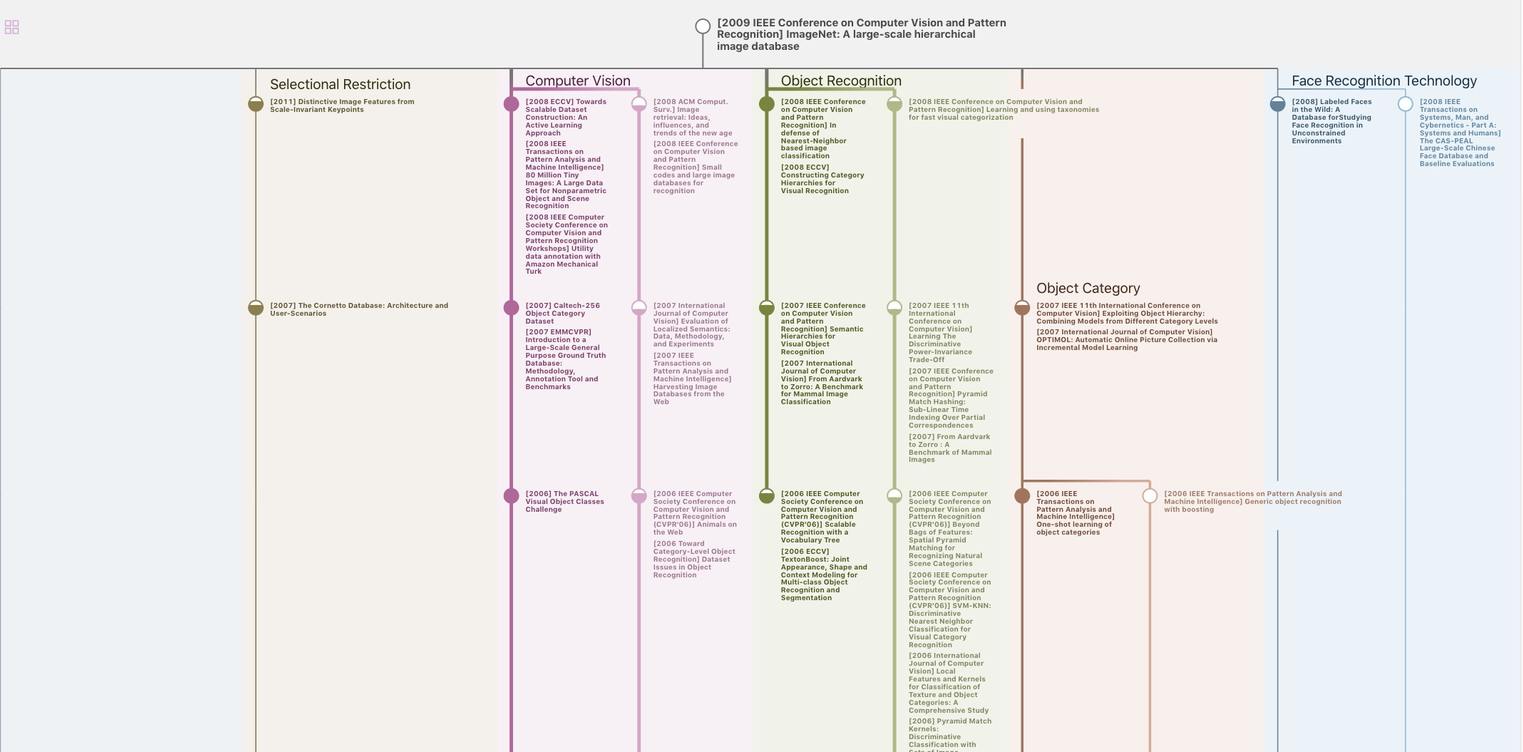
生成溯源树,研究论文发展脉络
Chat Paper
正在生成论文摘要