Are advanced methods necessary to improve infant fNIRS data analysis? An assessment of baseline-corrected averaging, general linear model (GLM) and multivariate pattern analysis (MVPA) based approaches
arxiv(2022)
摘要
In the last decade, fNIRS has provided a non-invasive method to investigate neural activation in developmental populations. Despite its increasing use in developmental cognitive neuroscience, there is little consistency or consensus on how to pre-process and analyse infant fNIRS data. With this registered report, we investigated the feasibility of applying more advanced statistical analyses to infant fNIRS data and compared the most commonly used baseline-corrected averaging, General Linear Model (GLM)-based univariate, and Multivariate Pattern Analysis (MVPA) approaches, to show how the conclusions one would draw based on these different analysis approaches converge or differ. The different analysis methods were tested using a face inversion paradigm where changes in brain activation in response to upright and inverted face stimuli were measured in thirty 4-to-6-month-old infants. By including more standard approaches together with recent machine learning techniques, we aim to inform the fNIRS community on alternative ways to analyse infant fNIRS datasets.
更多查看译文
AI 理解论文
溯源树
样例
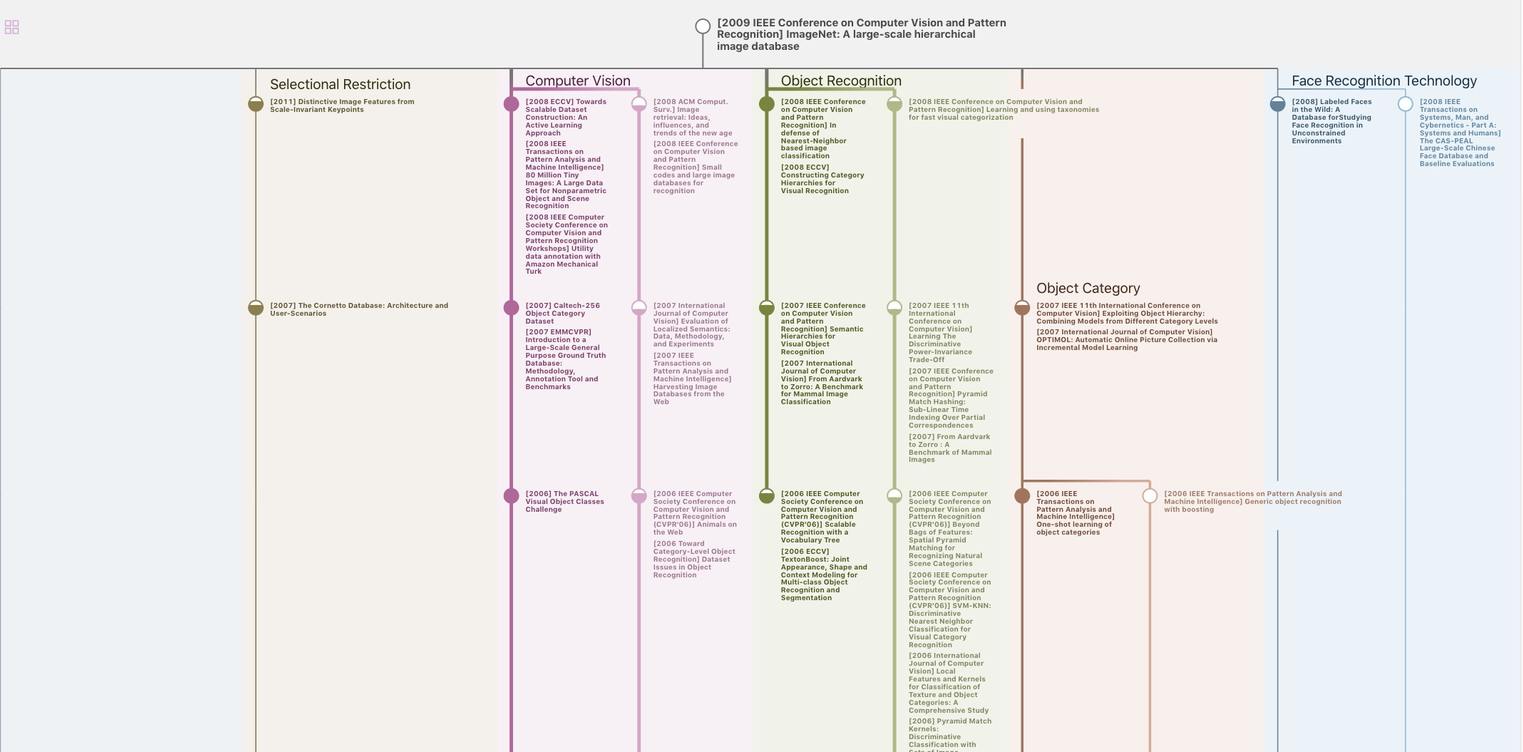
生成溯源树,研究论文发展脉络
Chat Paper
正在生成论文摘要