A Time Series is Worth 64 Words: Long-term Forecasting with Transformers
ICLR 2023(2022)
摘要
We propose an efficient design of Transformer-based models for multivariate time series forecasting and self-supervised representation learning. It is based on two key components: (i) segmentation of time series into subseries-level patches which are served as input tokens to Transformer; (ii) channel-independence where each channel contains a single univariate time series that shares the same embedding and Transformer weights across all the series. Patching design naturally has three-fold benefit: local semantic information is retained in the embedding; computation and memory usage of the attention maps are quadratically reduced given the same look-back window; and the model can attend longer history. Our channel-independent patch time series Transformer (PatchTST) can improve the long-term forecasting accuracy significantly when compared with that of SOTA Transformer-based models. We also apply our model to self-supervised pre-training tasks and attain excellent fine-tuning performance, which outperforms supervised training on large datasets. Transferring of masked pre-trained representation on one dataset to others also produces SOTA forecasting accuracy. Code is available at: https://github.com/yuqinie98/PatchTST.
更多查看译文
关键词
time series,transformer,forecasting,channel-independence,self-supervised learning,representation learning
AI 理解论文
溯源树
样例
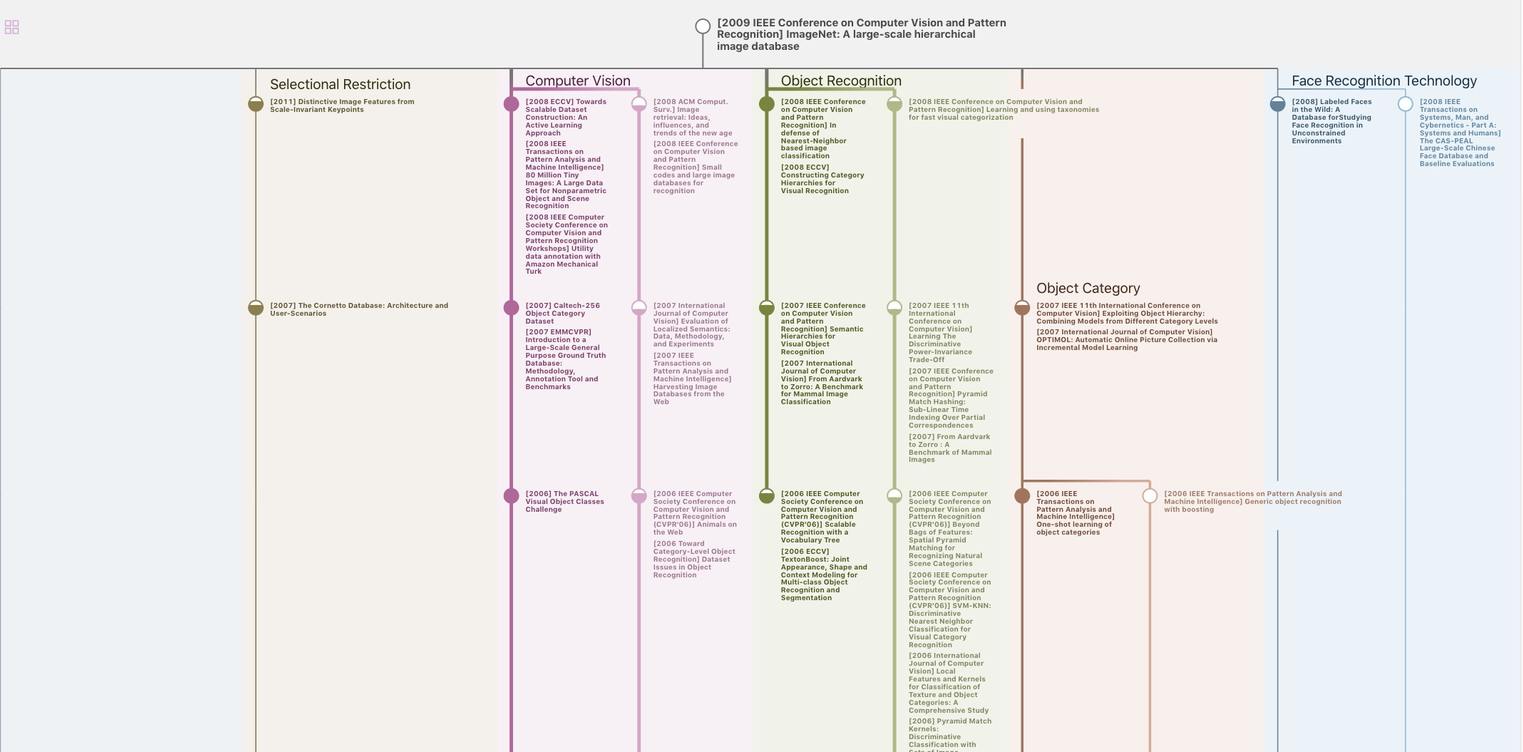
生成溯源树,研究论文发展脉络
Chat Paper
正在生成论文摘要