Multiple imputation for logistic regression models: incorporating an interaction
arxiv(2022)
摘要
Background: Multiple imputation is often used to reduce bias and gain efficiency when there is missing data. The most appropriate imputation method depends on the model the analyst is interested in fitting. Several imputation approaches have been proposed for when this model is a logistic regression model with an interaction term that contains a binary partially observed variable; however, it is not clear which performs best under certain parameter settings. Methods: Using 1000 simulations, each with 10,000 observations, under six data-generating mechanisms (DGM), we investigate the performance of four methods: (i) 'passive imputation', (ii) 'just another variable' (JAV), (iii) 'stratify-impute-append' (SIA), and (iv) 'substantive model compatible fully conditional specifica-tion' (SMCFCS). The application of each method is shown in an empirical example using England-based cancer registry data. Results: SMCFCS and SIA showed the least biased estimate of the coefficients for the fully, and partially, observed variable and the interaction term. SMCFCS and SIA showed good coverage and low relative error for all DGMs. SMCFCS had a large bias when there was a low prevalence of the fully observed variable in the interaction. SIA performed poorly when the fully observed variable in the interaction had a continuous underlying form. Conclusion: SMCFCS and SIA give consistent estimation for logistic regression models with an interaction term when data are missing at random, and either can be used in most analyses. SMCFCS performed better than SIA when the fully observed variable in the interaction had an underlying continuous form. Researchers should be cautious when using SMCFCS when there is a low prevalence of the fully observed variable in the interaction.
更多查看译文
关键词
multiple imputation,logistic regression models,logistic regression,interaction
AI 理解论文
溯源树
样例
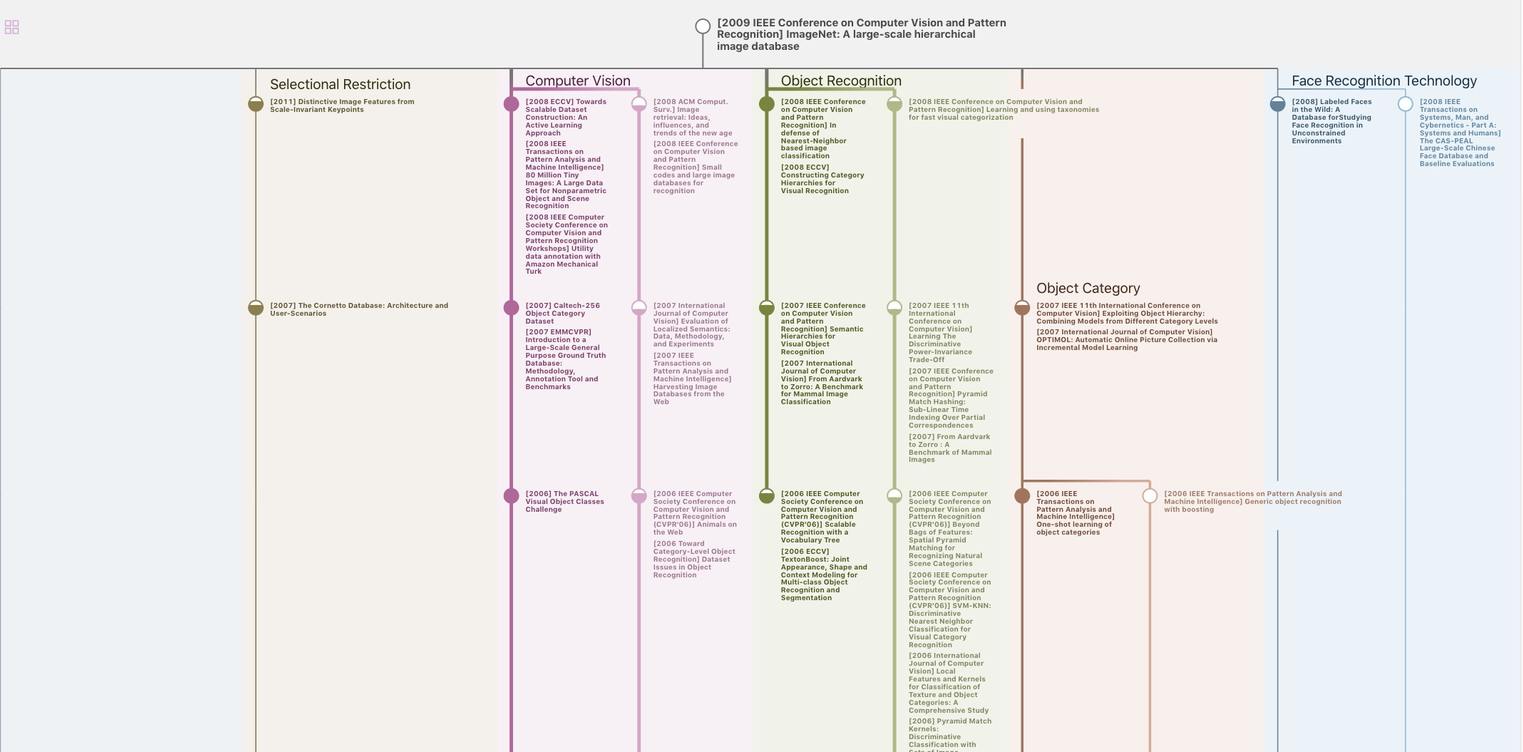
生成溯源树,研究论文发展脉络
Chat Paper
正在生成论文摘要