Comparative Analyses of Unsupervised PCA K-Means Change Detection Algorithm from the Viewpoint of Follow-Up Plan
SENSORS(2022)
摘要
In this study, principal component analysis and k-means clustering (PCAKM) methods for synthetic aperture radar (SAR) data are analyzed to reduce the sensitivity caused by changes in the parameters and input images of the algorithm, increase the accuracy, and make an improvement in the computation time, which are advantageous for scoring in the follow-up plan. Although there are many supervised methods described in the literature, unsupervised methods may be more appropriate in terms of computing time, data scarcity, and explainability in order to supply a trustworthy system. We consider the PCAKM algorithm, which is used as a benchmark method in many studies when making comparisons. Error metrics, computing times, and utility functions are calculated for 22 modified PCAKM regarding difference images and filtering methods. Various images with different characteristics affect the results of the configurations. However, it is evident that the PCAKM becomes less sensitive and more accurate for both the overall results and image results. Scoring by utilizing these results and other map information is a gap and innovation. Obtaining a change map in a fast, explainable, more robust and less sensitive way is one of the aims of our studies on scoring points in the follow-up plan.
更多查看译文
关键词
change detection, unsupervised learning, remote sensing, synthetic aperture radar, SAR image change detection, SAR images, follow-up plan, principal component analysis, k-means clustering
AI 理解论文
溯源树
样例
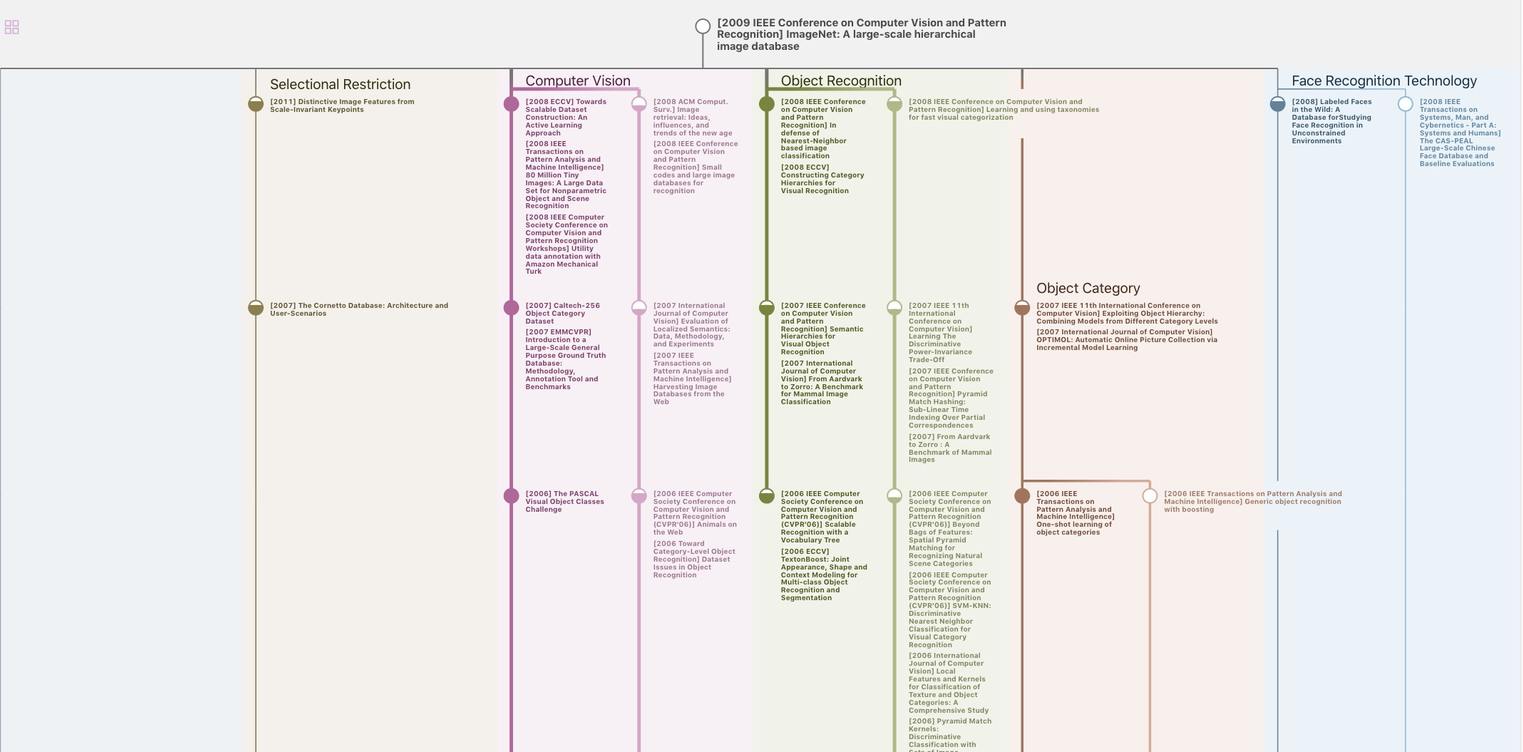
生成溯源树,研究论文发展脉络
Chat Paper
正在生成论文摘要