An Efficient Attention-Based Convolutional Neural Network That Reduces the Effects of Spectral Variability for Hyperspectral Unmixing
APPLIED SCIENCES-BASEL(2022)
摘要
The purpose of hyperspectral unmixing (HU) is to obtain the spectral features of materials (endmembers) and their proportion (abundance) in a hyperspectral image (HSI). Due to the existence of spectral variabilities (SVs), it is difficult to obtain accurate spectral features. At the same time, the performance of unmixing is not only affected by SVs but also depends on the effective spectral and spatial information. To solve these problems, this study proposed an efficient attention-based convolutional neural network (EACNN) and an efficient convolution block attention module (ECBAM). The EACNN is a two-stream network, which is learned from nearly pure endmembers through an additional network, and the aggregated spectral and spatial information can be obtained effectively with the help of the ECBAM, which can reduce the influence of SVs and improve the performance. The unmixing network helps the whole network to pay attention to meaningful feature information by using efficient channel attention (ECA) and guides the unmixing process by sharing parameters. Experimental results on three HSI datasets showed that the method proposed in this study outperformed other unmixing methods.
更多查看译文
关键词
hyperspectral unmixing,convolutional neural network,endmember bundle,spectral variability,attention mechanism
AI 理解论文
溯源树
样例
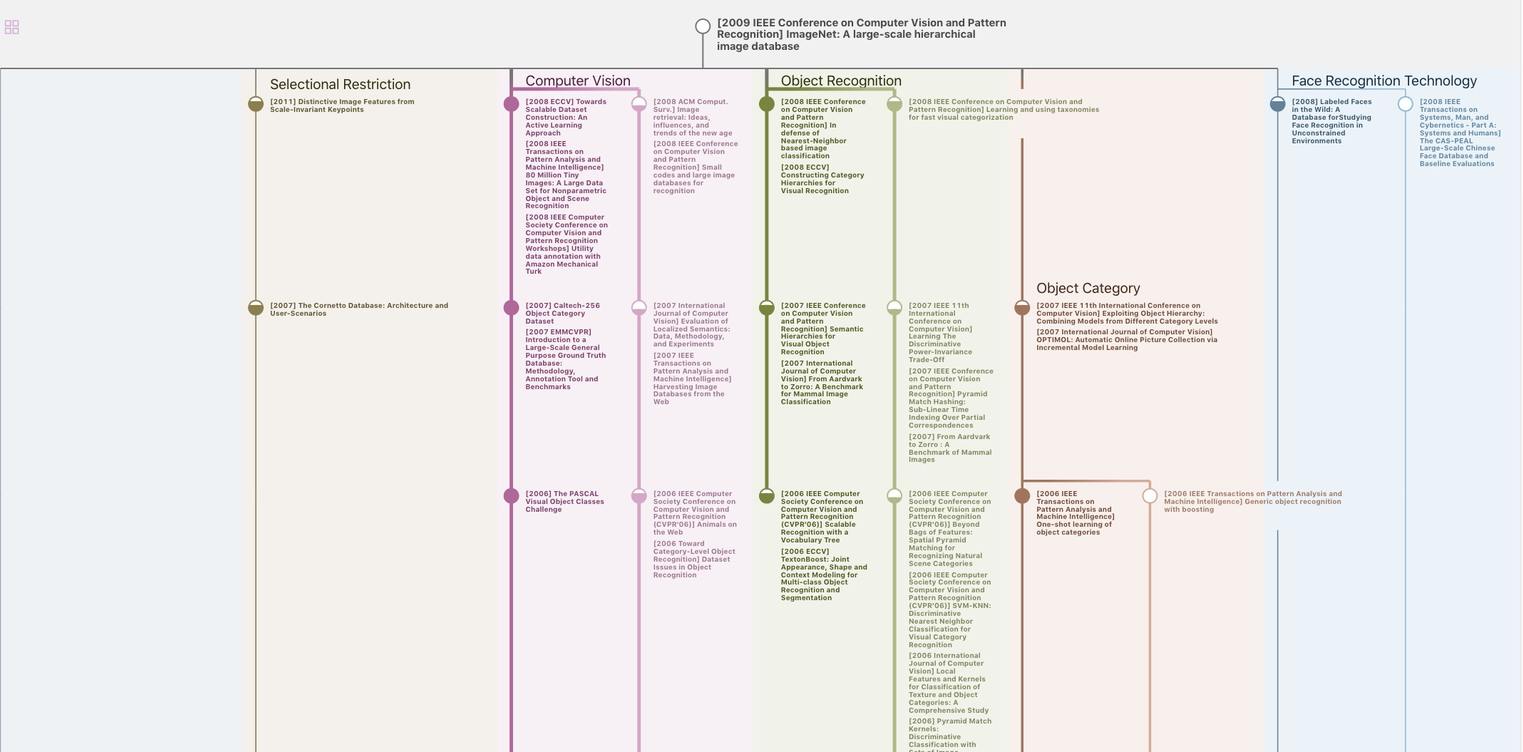
生成溯源树,研究论文发展脉络
Chat Paper
正在生成论文摘要