Toward Unlimited Self-Learning Monte Carlo with Annealing Process Using VAE's Implicit Isometricity
arxiv(2022)
摘要
Self-learning Monte Carlo (SLMC) methods are recently proposed to accelerate Markov chain Monte Carlo (MCMC) methods by using a machine learning model.With generative models having latent variables, SLMC methods realize efficient Monte Carlo updates with less autocorrelation. However, SLMC methods are difficult to directly apply to multimodal distributions for which training data are difficult to obtain. In this paper, we propose a novel SLMC method called the ``annealing VAE-SLMC" to drastically expand the range of applications. Our VAE-SLMC utilizes a variational autoencoder (VAE) as a generative model to make efficient parallel proposals independent of any previous state by applying the theoretically derived implicit isometricity of the VAE. We combine an adaptive annealing process to the VAE-SLMC, making our method applicable to the cases where obtaining unbiased training data is difficult in practical sense due to slow mixing. We also propose a parallel annealing process and an exchange process between chains to make the annealing operation more precise and efficient. Experiments validate that our method can proficiently obtain unbiased samples from multiple multimodal toy distributions and practical multimodal posterior distributions, which is difficult to achieve with the existing SLMC methods.
更多查看译文
AI 理解论文
溯源树
样例
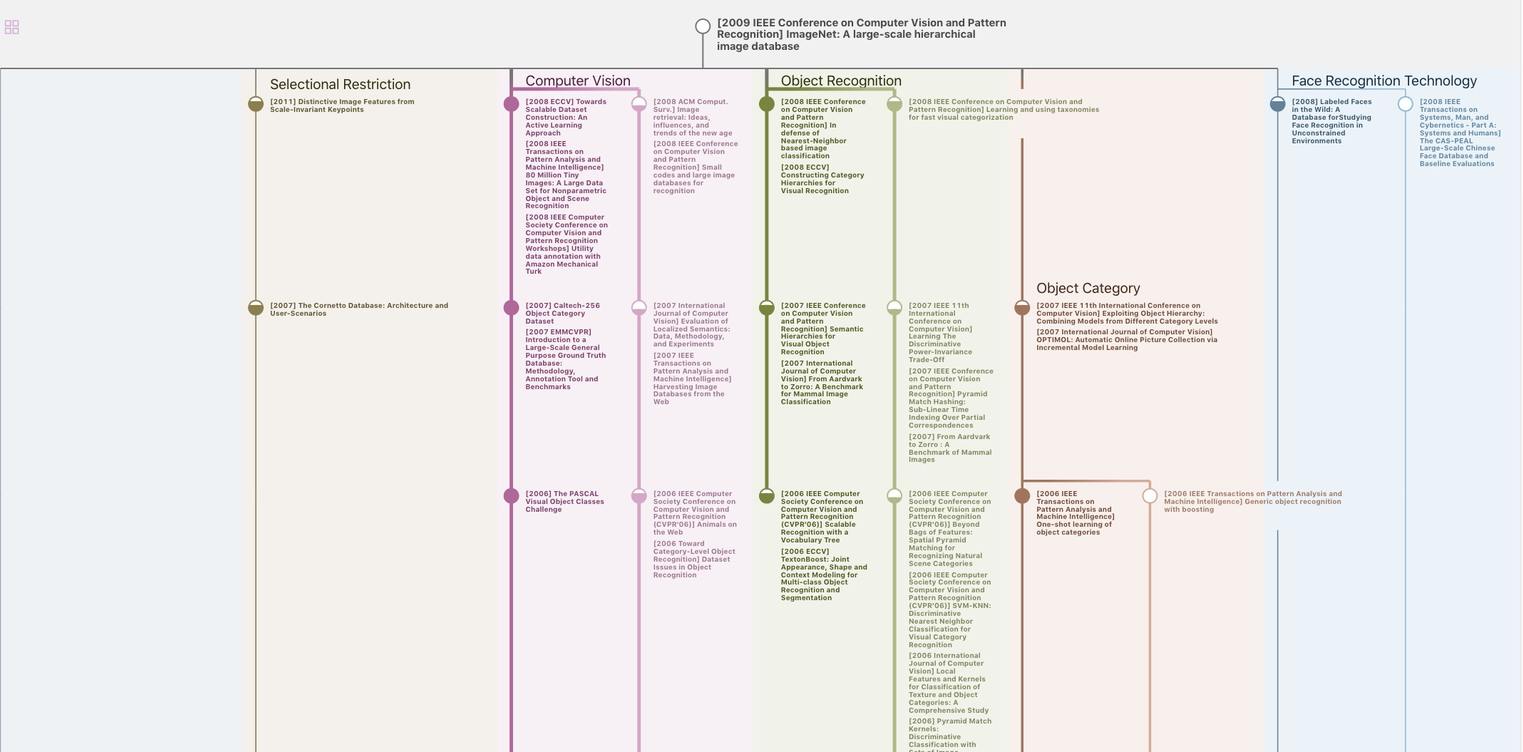
生成溯源树,研究论文发展脉络
Chat Paper
正在生成论文摘要