A Moment-Matching Approach to Testable Learning and a New Characterization of Rademacher Complexity
PROCEEDINGS OF THE 55TH ANNUAL ACM SYMPOSIUM ON THEORY OF COMPUTING, STOC 2023(2023)
摘要
A remarkable recent paper by Rubinfeld and Vasilyan (2022) initiated the study of testable learning, where the goal is to replace hard-to-verify distributional assumptions (such as Gaussianity) with efficiently testable ones and to require that the learner succeed whenever the unknown distribution passes the corresponding test. In this model, they gave an efficient algorithm for learning halfspaces under testable assumptions that are provably satisfied by Gaussians. In this paper we give a powerful new approach for developing algorithms for testable learning using tools from moment matching and metric distances in probability. We obtain efficient testable learners for any concept class that admits low-degree sandwiching polynomials, capturing most important examples for which we have ordinary agnostic learners. We recover the results of Rubinfeld and Vasilyan as a corollary of our techniques while achieving improved, near-optimal sample complexity bounds for a broad range of concept classes and distributions. Surprisingly, we show that the information-theoretic sample complexity of testable learning is tightly characterized by the Rademacher complexity of the concept class, one of the most well-studied measures in statistical learning theory. In particular, uniform convergence is necessary and sufficient for testable learning. This leads to a fundamental separation from (ordinary) distribution-specific agnostic learning, where uniform convergence is sufficient but not necessary.
更多查看译文
关键词
PAC learning,moment matching,sandwiching polynomials,generalization,Rademacher complexity
AI 理解论文
溯源树
样例
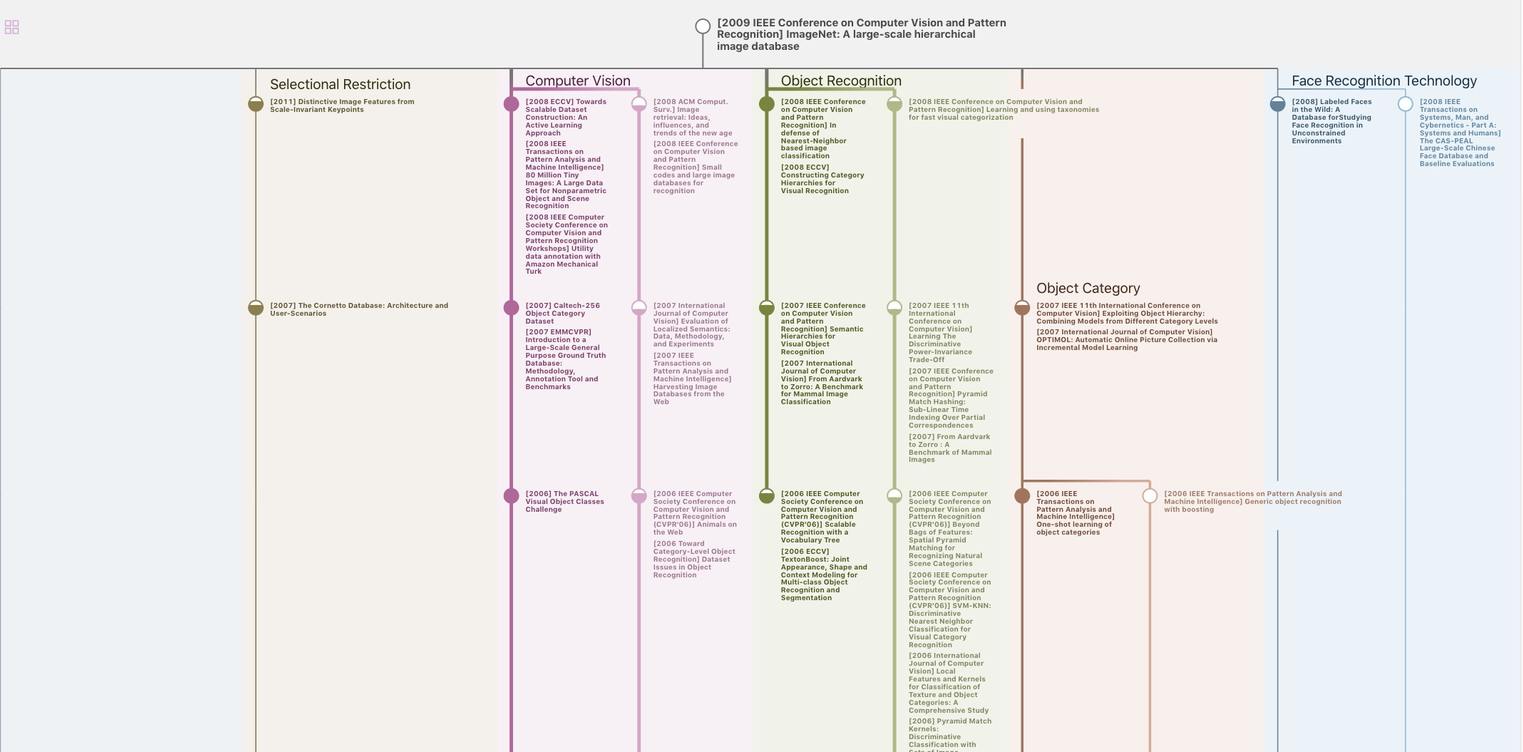
生成溯源树,研究论文发展脉络
Chat Paper
正在生成论文摘要