Adaptive Sampling Temporal Graph Network
2022 4th International Conference on Advances in Computer Technology, Information Science and Communications (CTISC)(2022)
摘要
Graph Neural Networks (GNNs) have recently received a surge of popularity due to their superiority in modeling realistic complex systems into graphs. Better yet, many approaches have made extraordinary contributions to this topic under the context of dynamic graph. However, current dynamic graph models merely focused on the design of the model but didn’t lay enough emphasis on the dynamism of data, which weakened the expressiveness of the output. Hence, we propose the Adaptive Sampling Temporal Graph Network (ASTGN), a Continuous-Time Dynamic Graph (CTDG) algorithm which casts the sampling strategy as a contextual bandit problem. To capture the dynamic information of graphs, we use a change-detection mechanism to keep the sampling strategy up with the times. Besides, we propose an additional constraint to keep our sampling path causal. We experimentally demonstrate the effectiveness of our approaches with six benchmarks and show the superiority over state-of-the-art baselines.
更多查看译文
关键词
GNN,change-detection mechanism,contextual bandit problem,graph dynamic information,CTDG,ASTGN,adaptive sampling temporal graph network,continuous-time dynamic graph algorithm,sampling strategy,graph neural networks
AI 理解论文
溯源树
样例
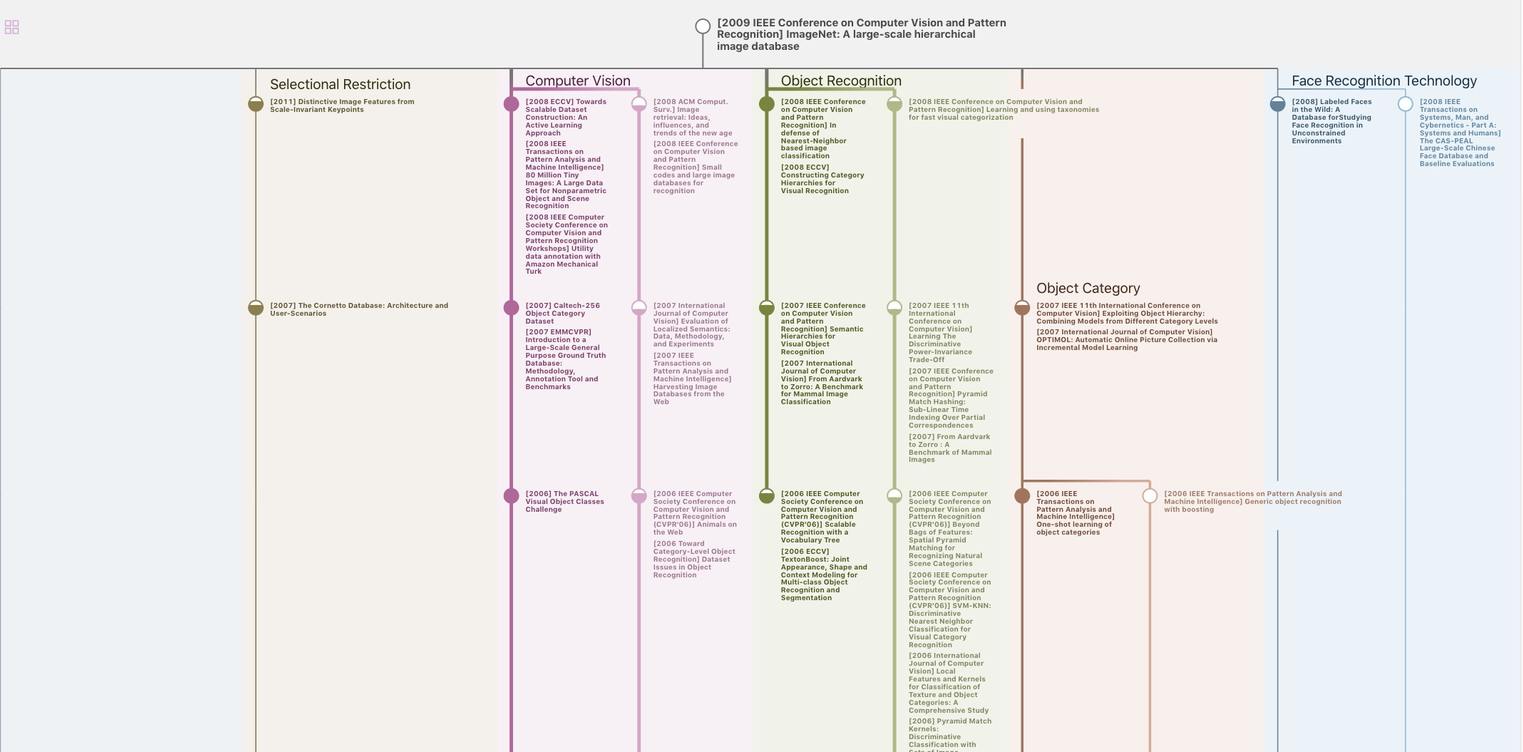
生成溯源树,研究论文发展脉络
Chat Paper
正在生成论文摘要