Video-Tensor Completion using a Deep Learning approach
2020 IEEE Colombian Conference on Applications of Computational Intelligence (IEEE ColCACI 2020)(2020)
摘要
The tensor completion problem solves the recovery of corrupted data in a multidimensional array named as a tensor. The traditional approaches in tensor completion are based on the transform tensor singular value decomposition(tt-SVD). These approaches minimize the tensor nuclear norm in a domain of an orthogonal transformation to induce low tensorial rank representation. Hence, they require previous knowledge of the data to ensure a low tensor rank representation and, therefore, to ensure a good quality reconstruction. On the other hand, based on the wide progress of deep learning in diverse contexts, this paper presents a 3DU-Net architecture for tensor data recovery in the problem of grayscale videos. The proposed method consists of convolutional layers with 3D filters to take advantage of the information at the spatio-temporal dimensions. The experimental results show that the proposed method has better performance in relative error (RE), peak-to-signal-ratio (PSNR), and less runtime compared with the state-of-the-art solutions. In particular, in the presence of noise, our proposed approach improves the recovery in up to 5.99 dB, and 0.09 in the RE with an 85% of corrupted pixels. In the noiseless case, the proposed architecture improves in 4.39 dB and 0.07 in the RE, when an 85% of the data is lost. Furthermore, the proposed method shows to be faster than the state-of-the-art in the reconstruction time in at least 2.5 times.
更多查看译文
关键词
Tensor Completion,Video Completion,Unet,CNN,tt-SVD
AI 理解论文
溯源树
样例
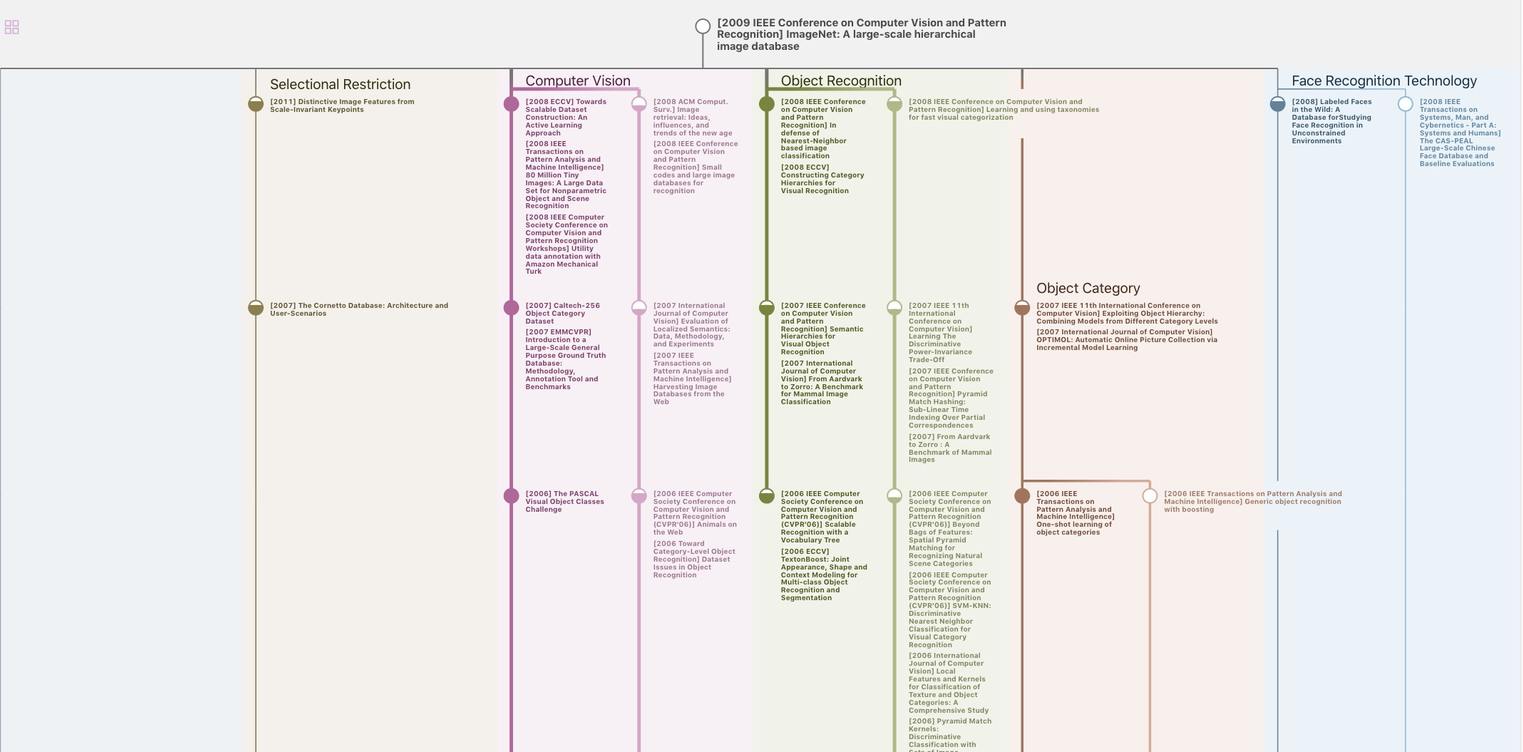
生成溯源树,研究论文发展脉络
Chat Paper
正在生成论文摘要