Safe reinforcement learning for affine nonlinear systems with state constraints and input saturation using control barrier functions
Neurocomputing(2023)
摘要
This paper provides a novel safe reinforcement learning (RL) control algorithm to solve safe optimal problems for discrete-time affine nonlinear systems, while the safety and convergence of the control algorithm are proven. The algorithm is proposed based on an adjusted policy iteration (PI) framework using only the measured data along the system trajectories in the environment. The adjusted PI algorithm combines with the system predictive information. Unlike most PI algorithms, an effective method of obtaining an initial safe and stable control policy is given here. In addition, control barrier functions (CBFs) and an input constraint function are introduced to augment reward functions. And the monotonically nonincreasing property of the iterative value function maintains the safe set forward invariant in the PI framework. Moreover, the safety and convergence of the proposed algorithm are proven in theory. Then, the design and implementation of the proposed algorithm are presented based on the identifier-actor-critic structure, where neural networks are employed to approximate the system dynamics, the iterative control policy, and the iterative value function, respectively. Finally, the simulation results illustrate the effectiveness and safety of the proposed algorithm.
更多查看译文
关键词
Reinforcement learning,Policy iteration,Control barrier function,Safety,State constraints,Input saturation
AI 理解论文
溯源树
样例
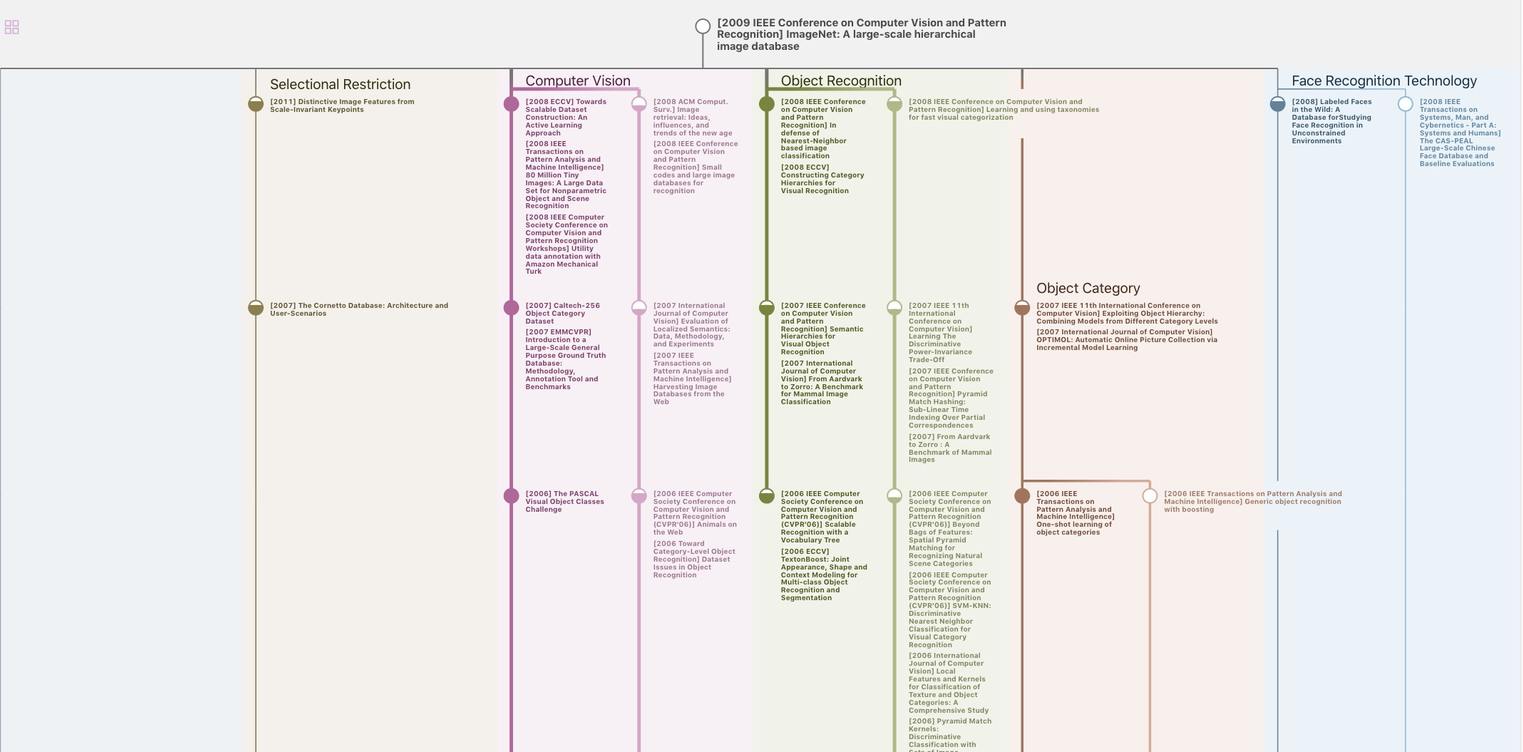
生成溯源树,研究论文发展脉络
Chat Paper
正在生成论文摘要