Feature Grouping for No-reference Image Quality Assessment
2022 7th International Conference on Automation, Control and Robotics Engineering (CACRE)(2022)
Abstract
No-reference image quality assessment (NR-IQA) is to evaluate the quality of the acquired distorted images by simulating the human subjective vision of a model. Currently, most NR-IQA methods research shallow features of images and do not pay attention to detail-rich deep features; moreover, the information exchange between sub-features extracted from deep features plays an important role in quality-aware features. Therefore, this paper proposes a novel, grouping feature-based NR-IQA model, which relies on sub-features from different levels combined with attention mechanisms. It consists of four parts: backbone network, grouping proposal, attention module, and head network. The backbone network extracts distorted image-related features and performs top-down and bottom-up architectures to complete feature grouping. The image distortion quality information is generated by filtering through the group attention module to obtain the quality features, and then the final distorted image quality is obtained by regression. The experimental results show that the proposed grouping architecture model (FGIQNet) outperforms other advanced NR-IQA models in four IQA databases of synthetic distortion and real distortion, and achieves excellent performance in cross-database evaluation, which proves the effectiveness and generalizability of the proposed model.
MoreTranslated text
Key words
NR-IQA,feature grouping,attention,quality features
AI Read Science
Must-Reading Tree
Example
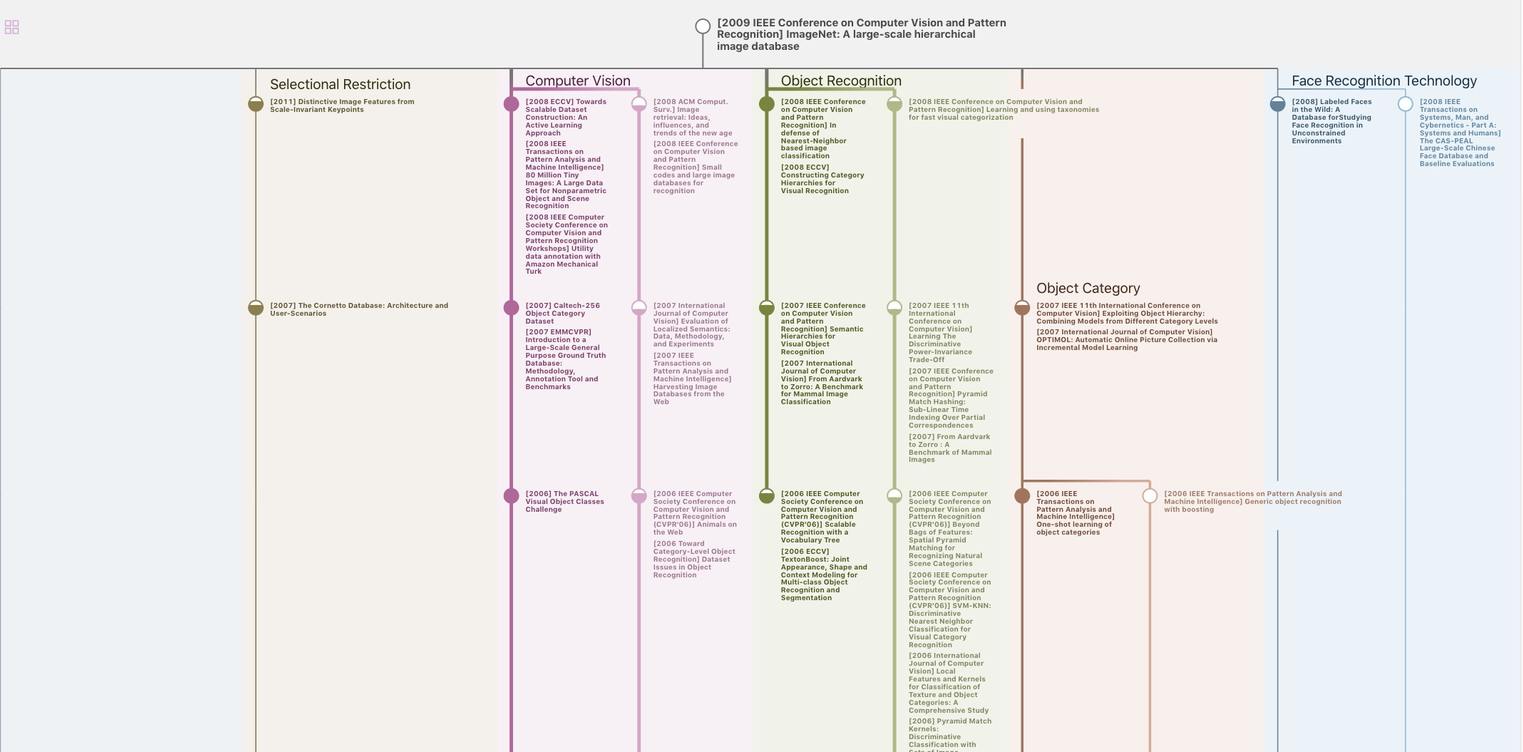
Generate MRT to find the research sequence of this paper
Chat Paper
Summary is being generated by the instructions you defined