Multi-layer guilt-by-association-based drug repurposing by integrating clinical knowledge on biological heterogeneous networks
biorxiv(2022)
摘要
Computational drug repurposing attempts to leverage rapidly accumulating high-throughput data to discover new indications for existing drugs, often by clarifying biological mechanisms with relevant genes. Leveraging the Guilt-by-association (GBA), the principle of "similar genes share similar functions," we introduced clinical neighbors of drug and disease entities while learning their mechanisms on the biological network. To overcome the hurdle of connecting drugs and diseases through large and dense gene-gene network and simultaneously realize the concept of "semantic multi-layer GBA", we present a random walk-based algorithm with a novel clinical-knowledge guided teleport. As a result, drug-disease association prediction accuracy increased up to 8.7% compared to existing state-of-the-art models. In addition, exploration of the generated embedding space displays harmony between biological and clinical contexts. Through repurposing case studies for breast carcinoma and Alzheimer's disease, we demonstrate the potential power of multi-layer GBA, a novel perspective for predicting clinical-level associations on heterogeneous biomedical networks.
### Competing Interest Statement
The authors have declared no competing interest.
更多查看译文
关键词
networks,clinical knowledge,drug,multi-layer,guilt-by-association-based
AI 理解论文
溯源树
样例
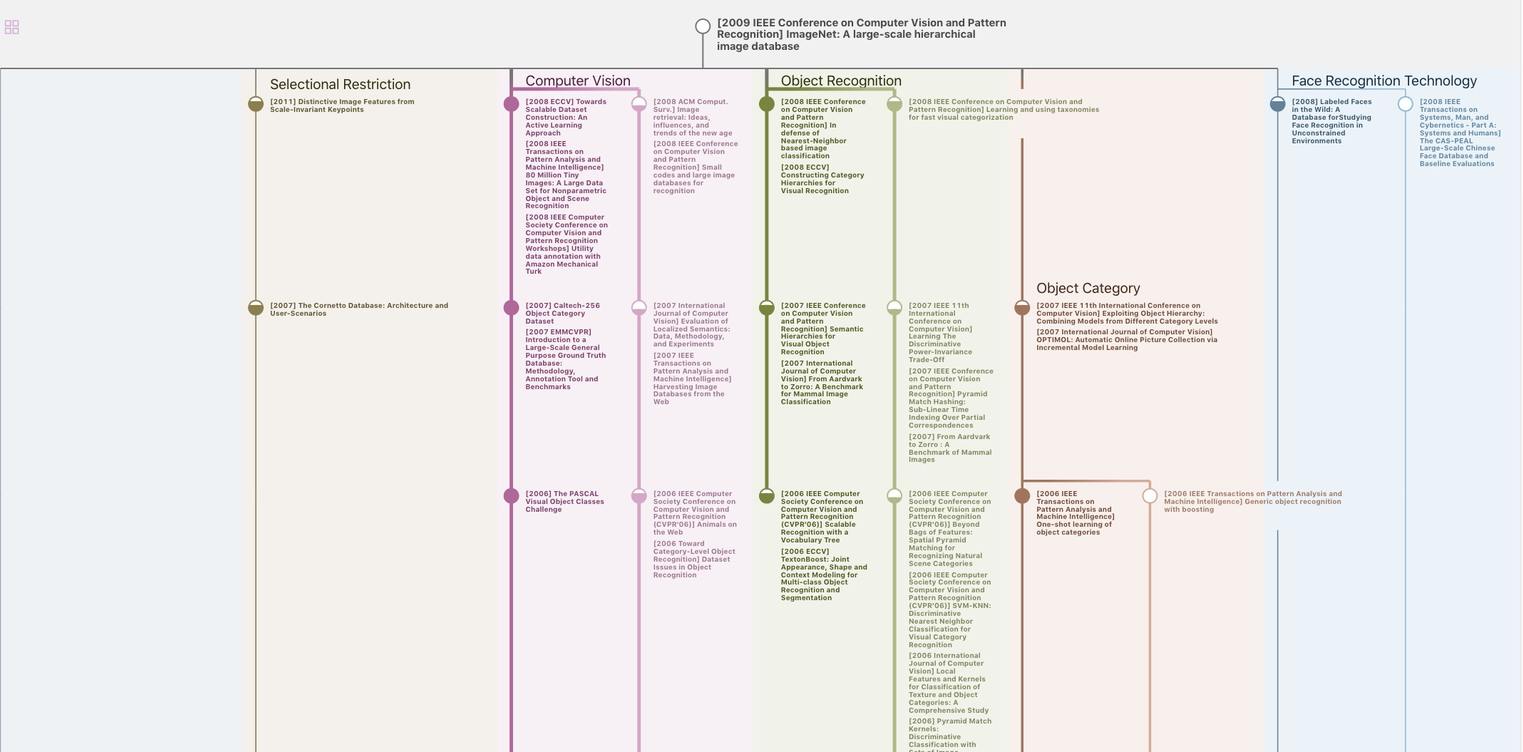
生成溯源树,研究论文发展脉络
Chat Paper
正在生成论文摘要