An Interactive Approach to Bias Mitigation in Machine Learning
2021 IEEE 20th International Conference on Cognitive Informatics & Cognitive Computing (ICCI*CC)(2021)
摘要
Underrepresentation and misrepresentation of protected groups in the training data is a significant source of bias for Machine Learning (ML) algorithms, resulting in decreased confidence and trustworthiness of the generated ML models. Such bias can be mitigated by incorporating both objective as well as subjective (through human users) measures of bias, and compensating for them by means of a suitable selection algorithm over subgroups of training data. In this paper, we propose a methodology of integrating bias detection and mitigation strategies through interactive visualization of machine learning models in selected protected spaces. In this approach, a (partially generated) ML model performance is visualized and evaluated by a human user or a community of human users in terms of potential presence of bias using both objective and subjective criteria. Guided by such human feedback, the ML algorithm can implement a variety of remedial sampling strategies to mitigate the bias using an iterative human-in-the-loop approach. We also provide experimental results with a benchmark ML dataset to demonstrate that such an interactive ML approach holds considerable promise in detecting and mitigating bias in ML models.
更多查看译文
关键词
fairness,bias,machine learning,visualization,human-computer interaction
AI 理解论文
溯源树
样例
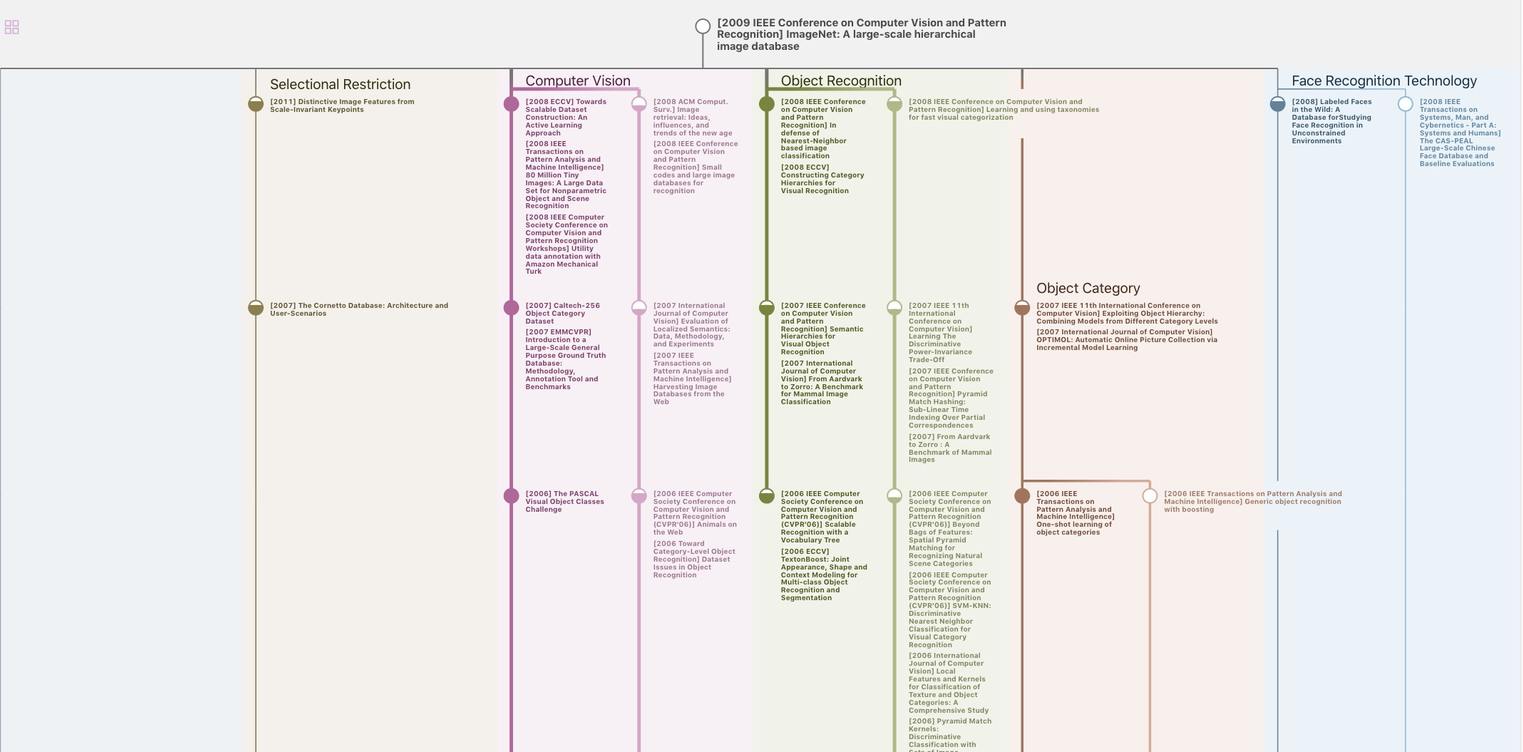
生成溯源树,研究论文发展脉络
Chat Paper
正在生成论文摘要