The Failure of Deep Neural Networks to Capture Human Language’s Cognitive Core
2021 IEEE 20th International Conference on Cognitive Informatics & Cognitive Computing (ICCI*CC)(2021)
摘要
Current deep neural networks have made remarkable advances in their ability to analyze and use natural language, with great apparent engineering success. But how well do these systems mirror the cognitive constraints associated with human language? In this talk we show that there are three essential core computations that characterize human language as an engine of human thought. One is "digital infinity"– the fact that we can produce an open-ended countably infinite number of sentences. The second is that sentences are hierarchically structured, rather than being arranged in a linear array. The third property is that human language computations always admit the possibility of "displacement" – a word or phrase can be pronounced at a place distinct from its usual location of semantic interpretation. All three properties can be shown to follow from a single, simple, recursive combinatorial operation. We provide empirical evidence for all three properties, both from concrete developmental examples as well as psycholinguistic and brain imaging experiments.What about current "deep neural network" systems? Although they perform very well after large-scale training, their success appears to be grounded on accurate table-lookup–memorization–without truly mirroring the three key computational principles of human language cognition. By "stress testing" currently available deep neural network processors, we show that they are, perhaps surprisingly very fragile when presented even with simple examples that deviate modestly from the examples on which they were trained. In particular, they fail to properly represent hierarchical structure and they cannot reliably reconstruct examples of sentences with "displacement" if the examples go just a bit beyond the complexity of their training set data. For example, while a deep neural network system might work on "Which cookie did Bob want," it fails on, "Which cookie did Bob want to eat." Such failures indicate that the neural net systems have not generalized in the same sense that children do, since children can easily handle such examples after receiving much more limited training data.
更多查看译文
关键词
natural language,cognitive constraints,human language computations,recursive combinatorial operation,concrete developmental examples,psycholinguistic brain imaging experiments,human language cognition,deep neural network processors,hierarchical structure,neural net systems
AI 理解论文
溯源树
样例
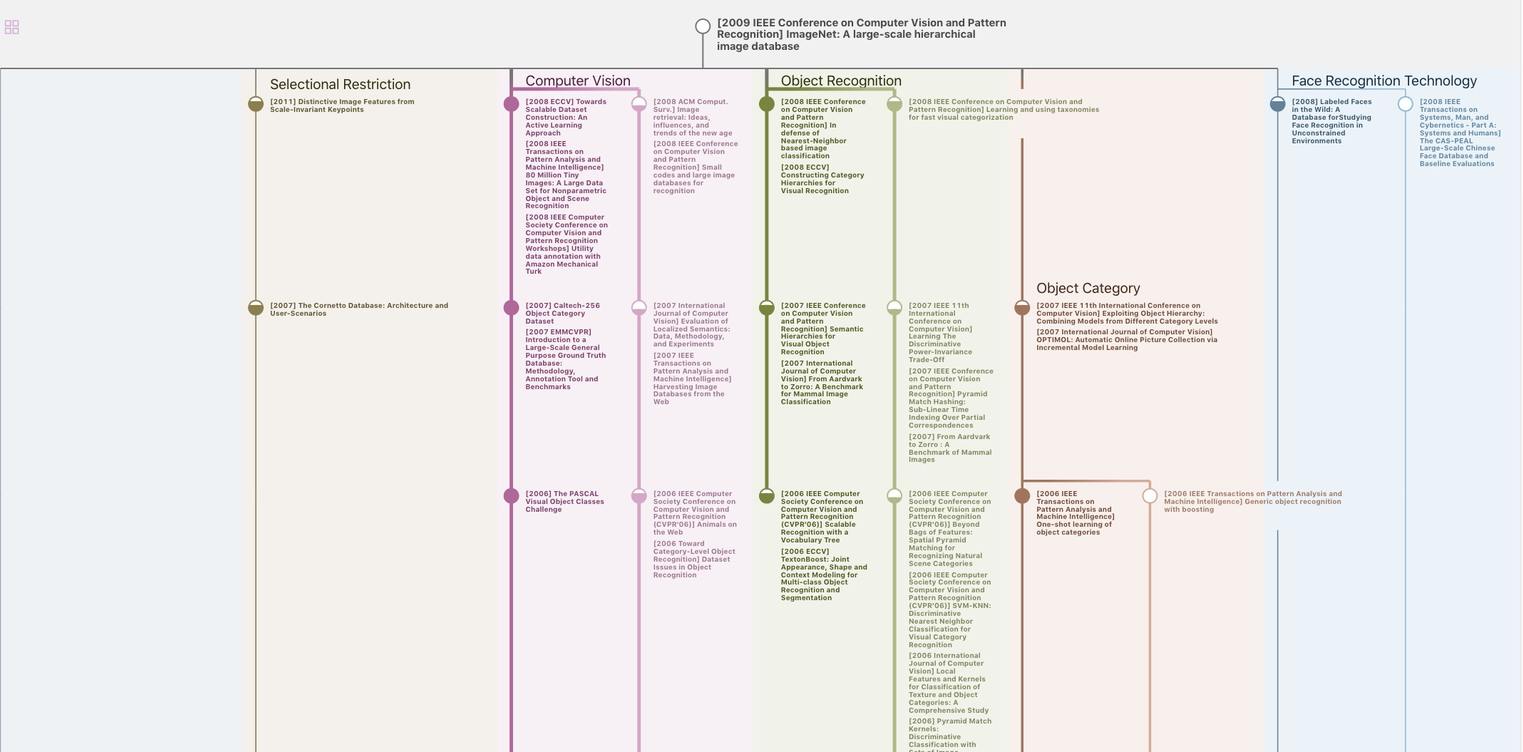
生成溯源树,研究论文发展脉络
Chat Paper
正在生成论文摘要