Deep Reinforcement Learning-Based Scheduling for Multiband Massive MIMO
IEEE ACCESS(2022)
摘要
Fifth-generation (5G) cellular communication systems have embraced massive multiple-input-multiple-output (MIMO) in the low- and mid-band frequencies. In a multiband system, the base station can serve different users in each band, while the user equipment can operate only in a single band simultaneously. This paper considers a massive MIMO system where channels are dynamically allocated in different frequency bands. We treat multiband massive MIMO as a scheduling and resource allocation problem and propose deep reinforcement learning (DRL) agents to perform user scheduling. The DRL agents use buffer and channel information to compose their observation space, and the agent's reward function maximizes the transmitted throughput and minimizes the packet loss rate. We compare the proposed DRL algorithms with traditional baselines, such as maximum throughput and proportional fairness. The results show that the DRL models outperformed baselines obtaining a 20% higher network sum rate and an 84% smaller packet loss rate. Moreover, we compare different DRL algorithms focusing on training time to assess the online implementation of the DRL agents, showing that the best agent needs about 50K training steps to converge.
更多查看译文
关键词
Multiband scheduling,MIMO,DRL-based scheduling,mmWave
AI 理解论文
溯源树
样例
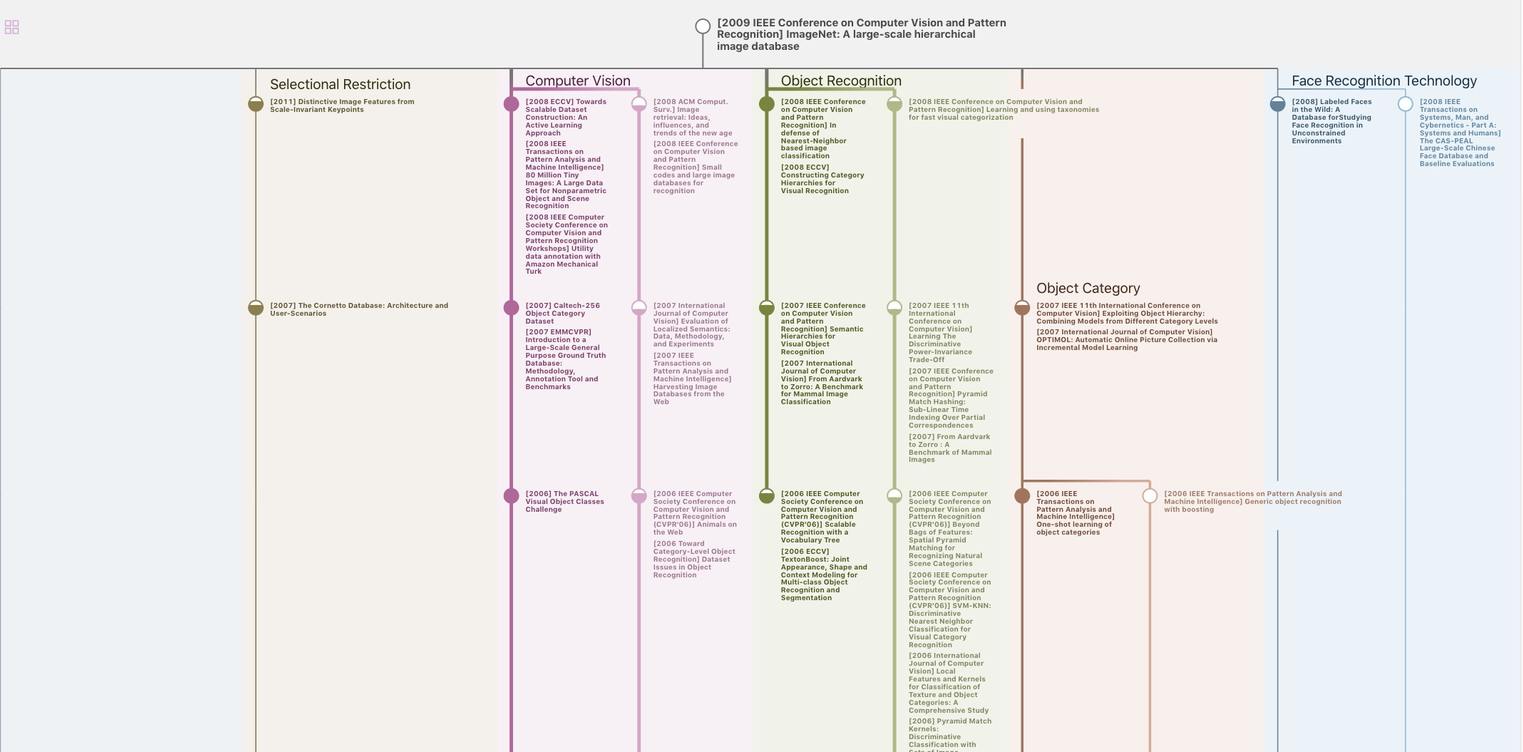
生成溯源树,研究论文发展脉络
Chat Paper
正在生成论文摘要