Deep Neural Networks for Direction of Arrival Estimation of Multiple Targets With Sparse Prior for Line-of-Sight Scenarios
IEEE Transactions on Vehicular Technology(2023)
摘要
Received signal Direction of Arrival (DOA) estimation represents a significant problem with multiple applications, ranging from wireless communications to radars. This problem presents significant challenges, mainly given by a large number of closely located transmitters being difficultly separable. Currently available state of the art approaches fail in providing sufficient resolution to separate and recognize the DOA of closely located transmitters, unless using a large number of antennas and hence increasing the deployment and operation costs. In this paper, we present a deep learning framework for DOA estimation under Line-of-Sight scenarios, which able to distinguish a number of closely located sources higher than the number of receivers' antennas. We first propose a formulation that maps the received signal to a higher dimensional space that allows for better identification of signal sources. Secondly, we introduce a Deep Neural Network that learns the mapping from the receiver antenna space to the extended space to avoid relying on specific receiver antenna array structures. Thanks to our approach, we reduce the hardware complexity compared to state of the art solutions and allow reconfigurability of the receiver channels. Via extensive numerical simulations, we demonstrate the superiority of our proposed method compared to state-of-the-art deep learning-based DOA estimation methods, especially in demanding scenarios with low Signal-to-Noise Ratio and limited number of snapshots.
更多查看译文
关键词
Direction-of-arrival estimation,Antenna arrays,Estimation,Receiving antennas,Costs,Deep learning,Covariance matrices,DOA estimation,deep neural network,sparse representation,multiple targets
AI 理解论文
溯源树
样例
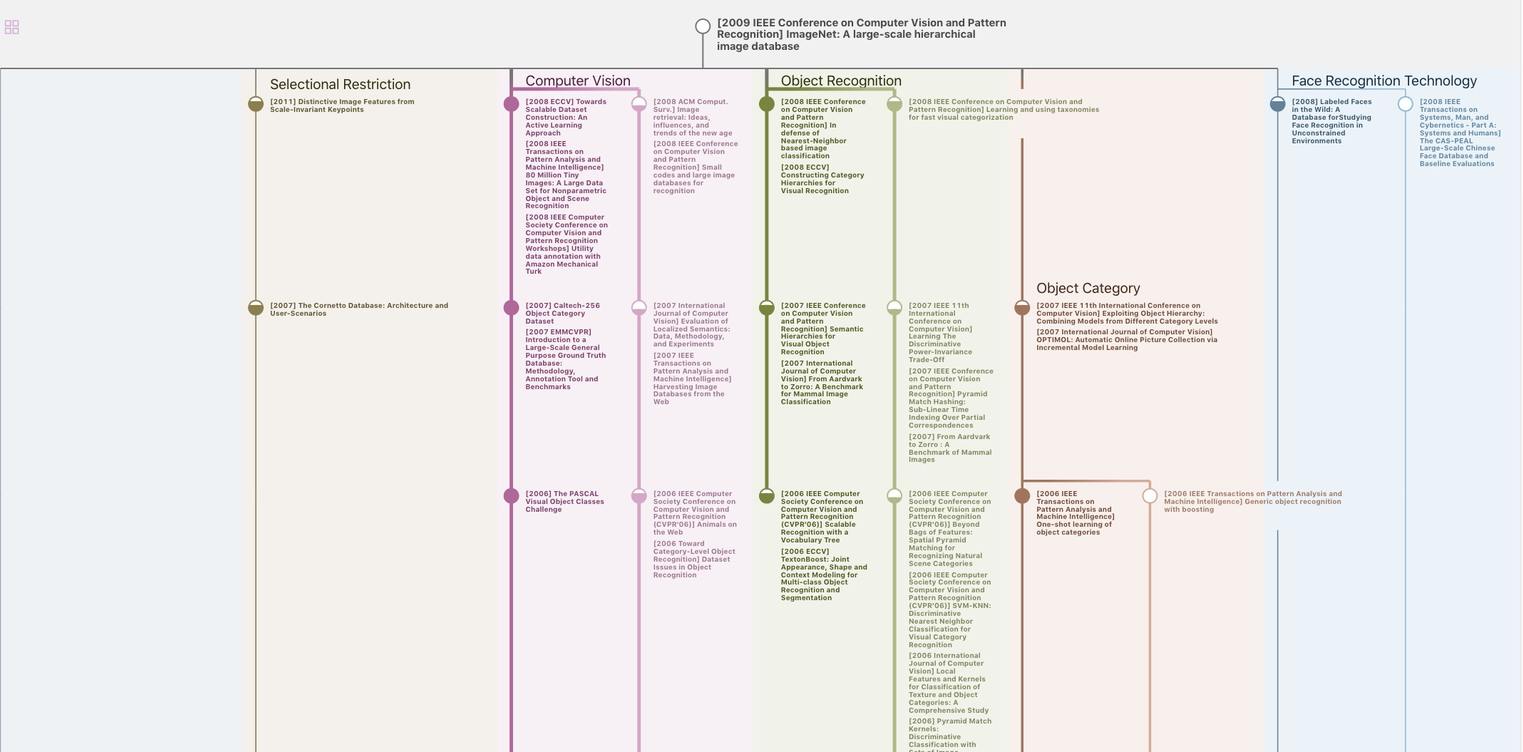
生成溯源树,研究论文发展脉络
Chat Paper
正在生成论文摘要