A Data-Driven Approach for the Localization of Interacting Agents via a Multi-Modal Dynamic Bayesian Network Framework
2022 18th IEEE International Conference on Advanced Video and Signal Based Surveillance (AVSS)(2022)
Abstract
This paper proposes a multi-modal situational inter-action model for collaborative agents by fusing multi-sensorial information in a Multi-Agent Hierarchical Dynamic Bayesian Network (MAH-DBN) framework. The proposed model is learned in a data-driven methodology to estimate the states of interacting agents only from video sequences. This can be regarded as a two-fold methodology for improving visual-based localization and interaction between autonomous agents. In the learning stage, the odometry model is used to drive the video learning model for a robust localization and interaction modeling. During the testing phase, the learned Multi-Agent Hierarchical DBN (MAH-DBN) model is used for the localization of collaborative agents only from video sequences by proposing an inference method called Multi-Agent Coupled Markov Jump Particle Filter (MAC-MJPF).
MoreTranslated text
AI Read Science
Must-Reading Tree
Example
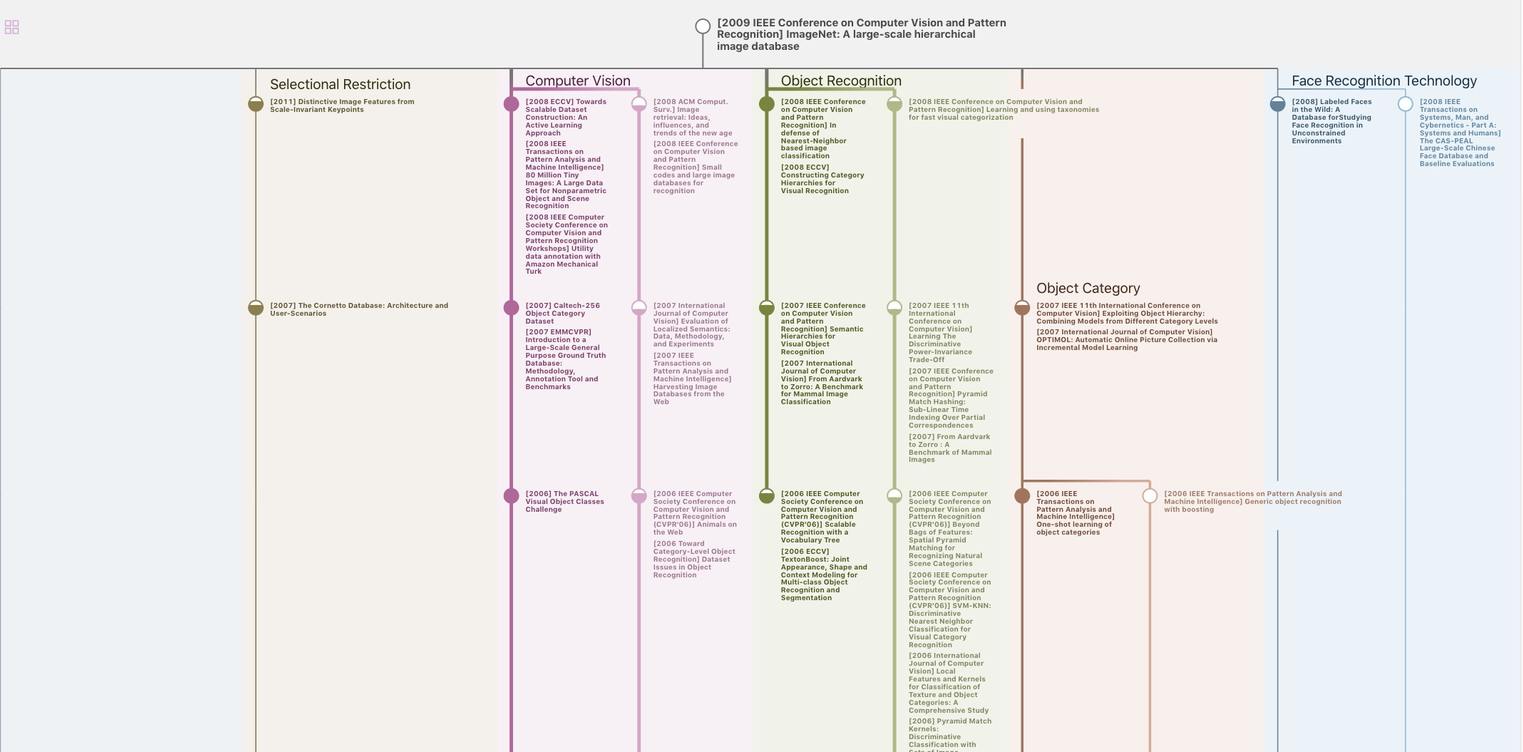
Generate MRT to find the research sequence of this paper
Chat Paper
Summary is being generated by the instructions you defined