Multi-Step Spectrum States Prediction Based on Long Short-Term Memory
2022 IEEE/CIC International Conference on Communications in China (ICCC Workshops)(2022)
摘要
To support increasing number of wireless devices under limited spectrum resources, it is of great significance to explore more efficient spectrum usage strategies. In this paper, a spectrum states prediction approach based on long short-term memory (LSTM) has been proposed to reduce latency and improve spectrum efficiency. We first determine the threshold adaptively by the kernel density estimation (KDE) method, and then data preprocessing is performed on the retrieved dataset. After that, the preprocessed data is fed into the LSTM network for training and validation. The proposed scheme is capable of single-step and multi-step prediction with different time resolutions. At last, simulation results are presented and discussed to show the effectiveness of the proposed approach. By taking advantage of the information in historical data, the devices can perform channel pre-selection and avoid conflict efficiently.
更多查看译文
关键词
Dynamic spectrum access,long short-term memory,power spectrum density,spectrum states prediction
AI 理解论文
溯源树
样例
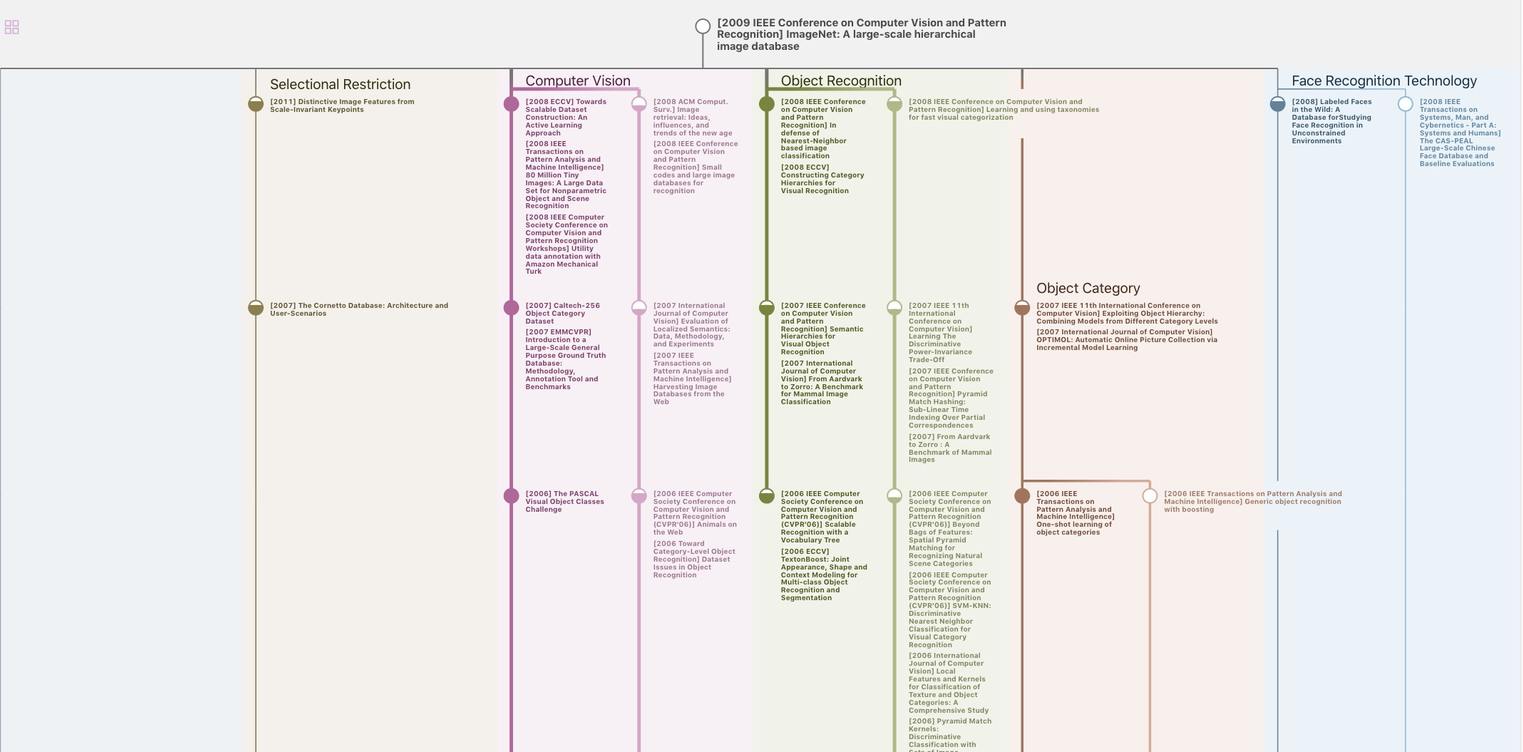
生成溯源树,研究论文发展脉络
Chat Paper
正在生成论文摘要