Deep progressive learning achieves whole-body low-dose F-18-FDG PET imaging
EJNMMI physics(2022)
Abstract
Objectives To validate a total-body PET-guided deep progressive learning reconstruction method (DPR) for low-dose F-18-FDG PET imaging. Methods List-mode data from the retrospective study (n = 26) were rebinned into short-duration scans and reconstructed with DPR. The standard uptake value (SUV) and tumor-to-liver ratio (TLR) in lesions and coefficient of variation (COV) in the liver in the DPR images were compared to the reference (OSEM images with full-duration data). In the prospective study, another 41 patients were injected with 1/3 of the activity based on the retrospective results. The DPR images (DPR_1/3(p)) were generated and compared with the reference (OSEM images with extended acquisition time). The SUV and COV were evaluated in three selected organs: liver, blood pool and muscle. Quantitative analyses were performed with lesion SUV and TLR, furthermore on small lesions (<= 10 mm in diameter). Additionally, a 5-point Likert scale visual analysis was performed on the following perspectives: contrast, noise and diagnostic confidence. Results In the retrospective study, the DPR with one-third duration can maintain the image quality as the reference. In the prospective study, good agreement among the SUVs was observed in all selected organs. The quantitative results showed that there was no significant difference in COV between the DPR_1/3(p) group and the reference, while the visual analysis showed no significant differences in image contrast, noise and diagnostic confidence. The lesion SUVs and TLRs in the DPR_1/3(p) group were significantly enhanced compared with the reference, even for small lesions. Conclusions The proposed DPR method can reduce the administered activity of F-18-FDG by up to 2/3 in a real-world deployment while maintaining image quality.
MoreTranslated text
Key words
PET, Deep progressive learning, Low dose, Image quality
AI Read Science
Must-Reading Tree
Example
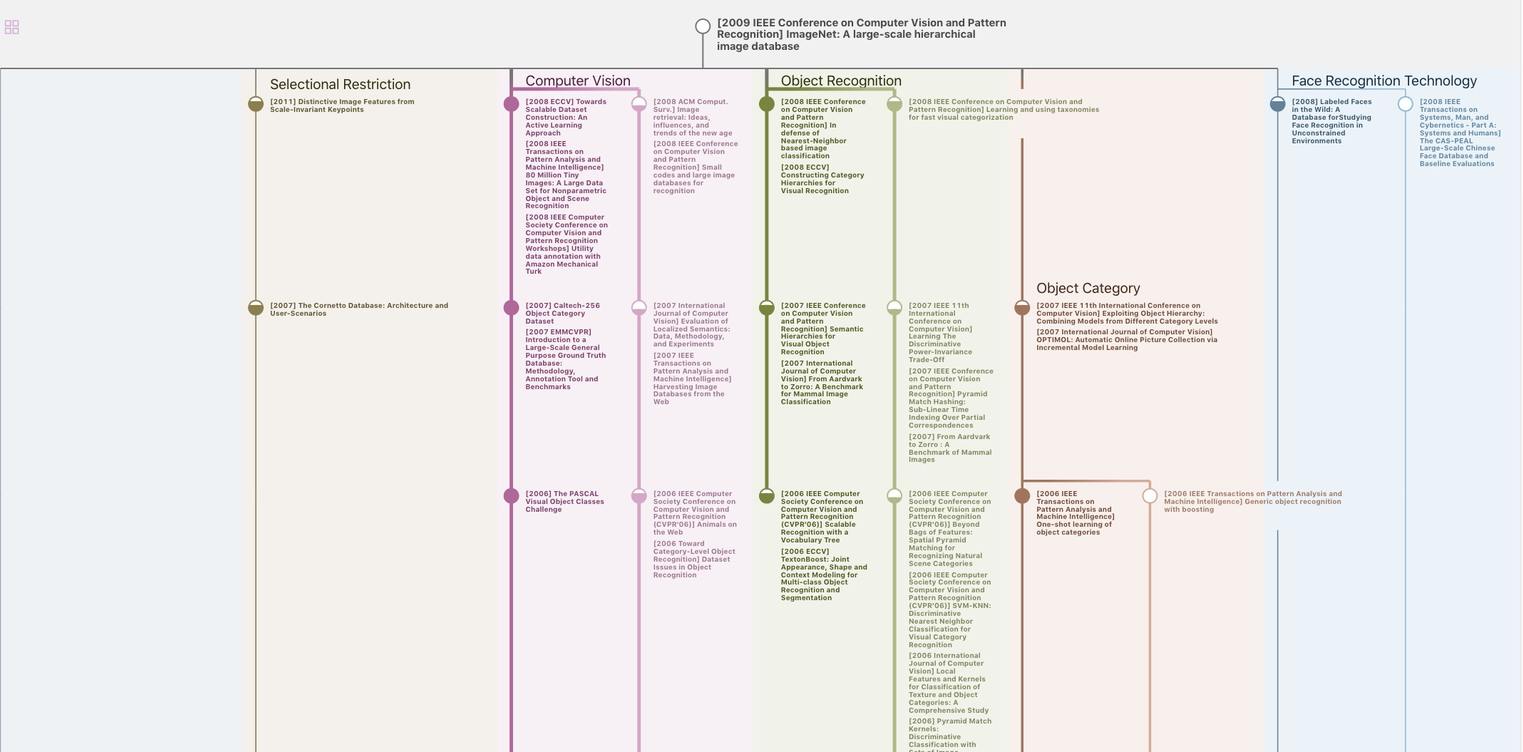
Generate MRT to find the research sequence of this paper
Chat Paper
Summary is being generated by the instructions you defined