A Hamilton-Jacobi-based Proximal Operator
PROCEEDINGS OF THE NATIONAL ACADEMY OF SCIENCES OF THE UNITED STATES OF AMERICA(2023)
摘要
First-order optimization algorithms are widely used today. Two standard building blocks in these algorithms are proximal operators (proximals) and gradients. Although gradients can be computed for a wide array of functions, explicit proximal formulas are only known for limited classes of functions. We provide an algorithm, HJ-Prox, for accurately approximating such proximals. This is derived from a collection of relations between proximals, Moreau envelopes, Hamilton-Jacobi (HJ) equations, heat equations, and Monte Carlo sampling. In particular, HJ-Prox smoothly approximates the Moreau envelope and its gradient. The smoothness can be adjusted to act as a denoiser. Our approach applies even when functions are only accessible by (possibly noisy) blackbox samples. We show HJ-Prox is effective numerically via several examples.
更多查看译文
关键词
Cole–Hopf,Hamilton–Jacobi,Moreau,proximal,zeroth-order
AI 理解论文
溯源树
样例
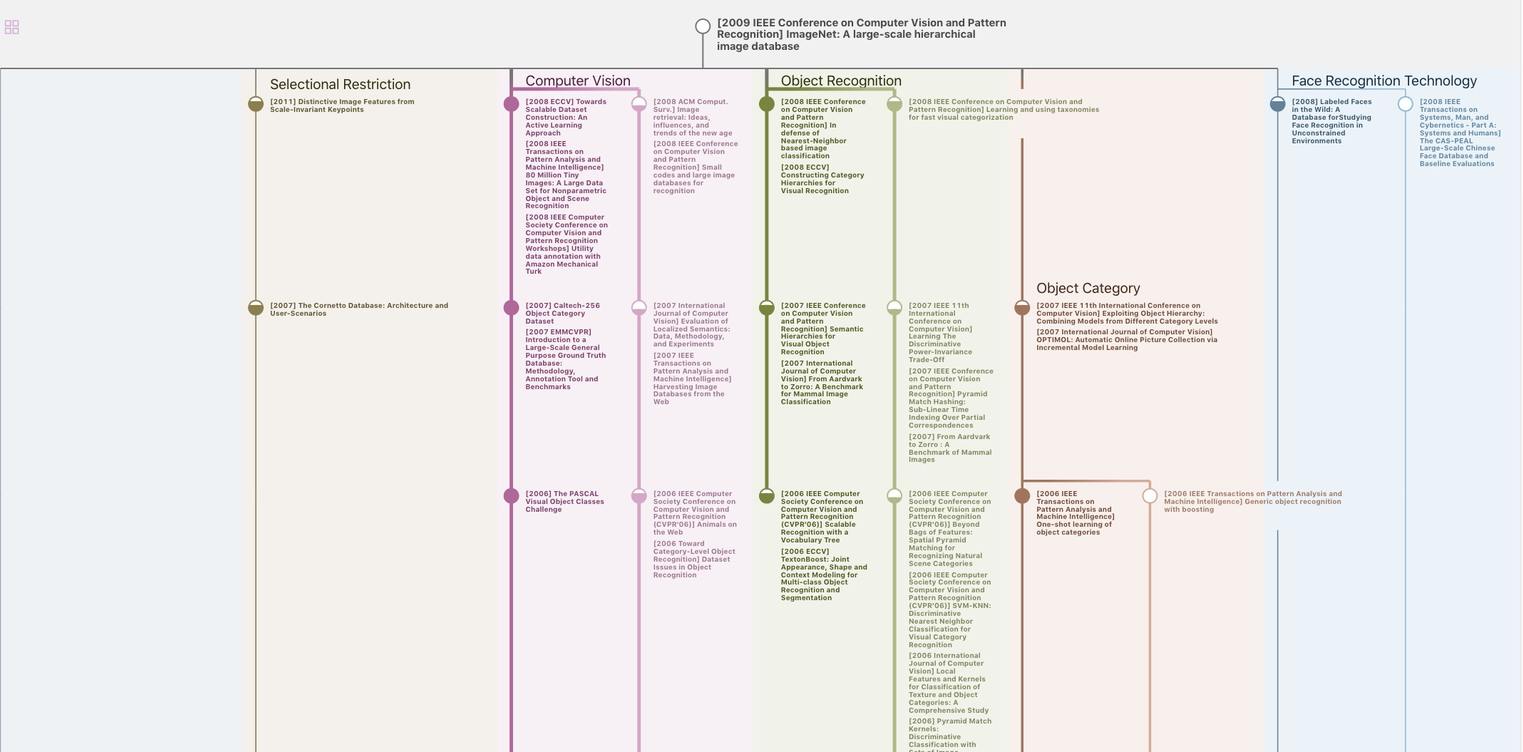
生成溯源树,研究论文发展脉络
Chat Paper
正在生成论文摘要