Adversarial Attacks are a Surprisingly Strong Baseline for Poisoning Few-Shot Meta-Learners
arxiv(2022)
摘要
This paper examines the robustness of deployed few-shot meta-learning systems when they are fed an imperceptibly perturbed few-shot dataset. We attack amortized meta-learners, which allows us to craft colluding sets of inputs that are tailored to fool the system's learning algorithm when used as training data. Jointly crafted adversarial inputs might be expected to synergistically manipulate a classifier, allowing for very strong data-poisoning attacks that would be hard to detect. We show that in a white box setting, these attacks are very successful and can cause the target model's predictions to become worse than chance. However, in opposition to the well-known transferability of adversarial examples in general, the colluding sets do not transfer well to different classifiers. We explore two hypotheses to explain this: 'overfitting' by the attack, and mismatch between the model on which the attack is generated and that to which the attack is transferred. Regardless of the mitigation strategies suggested by these hypotheses, the colluding inputs transfer no better than adversarial inputs that are generated independently in the usual way.
更多查看译文
关键词
poisoning
AI 理解论文
溯源树
样例
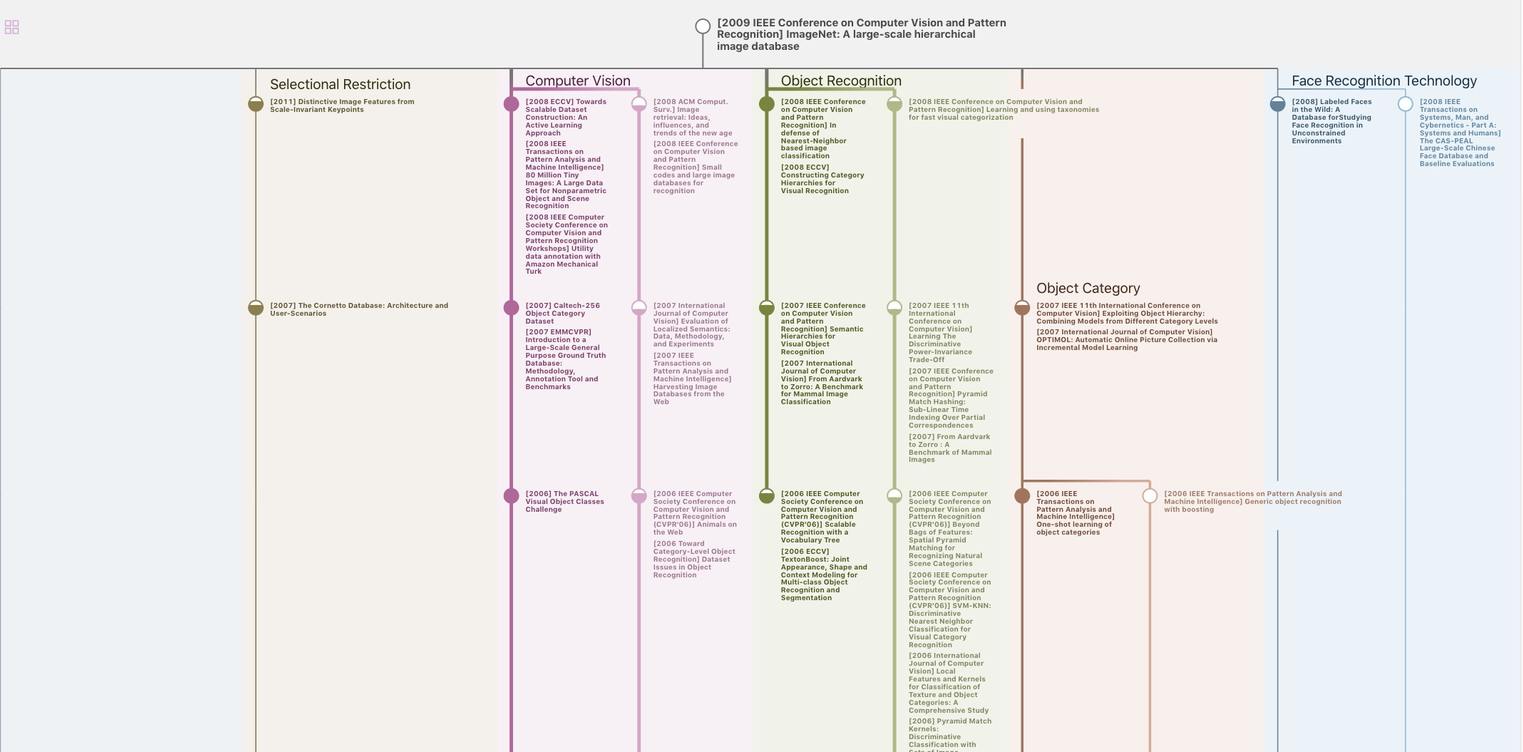
生成溯源树,研究论文发展脉络
Chat Paper
正在生成论文摘要