{\mu}Split: efficient image decomposition for microscopy data
CoRR(2023)
Abstract
We present uSplit, a dedicated approach for trained image decomposition in the context of fluorescence microscopy images. We find that best results using regular deep architectures are achieved when large image patches are used during training, making memory consumption the limiting factor to further improving performance. We therefore introduce lateral contextualization (LC), a memory efficient way to train powerful networks and show that LC leads to consistent and significant improvements on the task at hand. We integrate LC with U-Nets, Hierarchical AEs, and Hierarchical VAEs, for which we formulate a modified ELBO loss. Additionally, LC enables training deeper hierarchical models than otherwise possible and, interestingly, helps to reduce tiling artefacts that are inherently impossible to avoid when using tiled VAE predictions. We apply uSplit to five decomposition tasks, one on a synthetic dataset, four others derived from real microscopy data. LC achieves SOTA results (average improvements to the best baseline of 2.36 dB PSNR), while simultaneously requiring considerably less GPU memory.
MoreTranslated text
Key words
Medical Image Analysis,Image Processing,Cellular Imaging,Meta-Learning,Semi-Supervised Learning
AI Read Science
Must-Reading Tree
Example
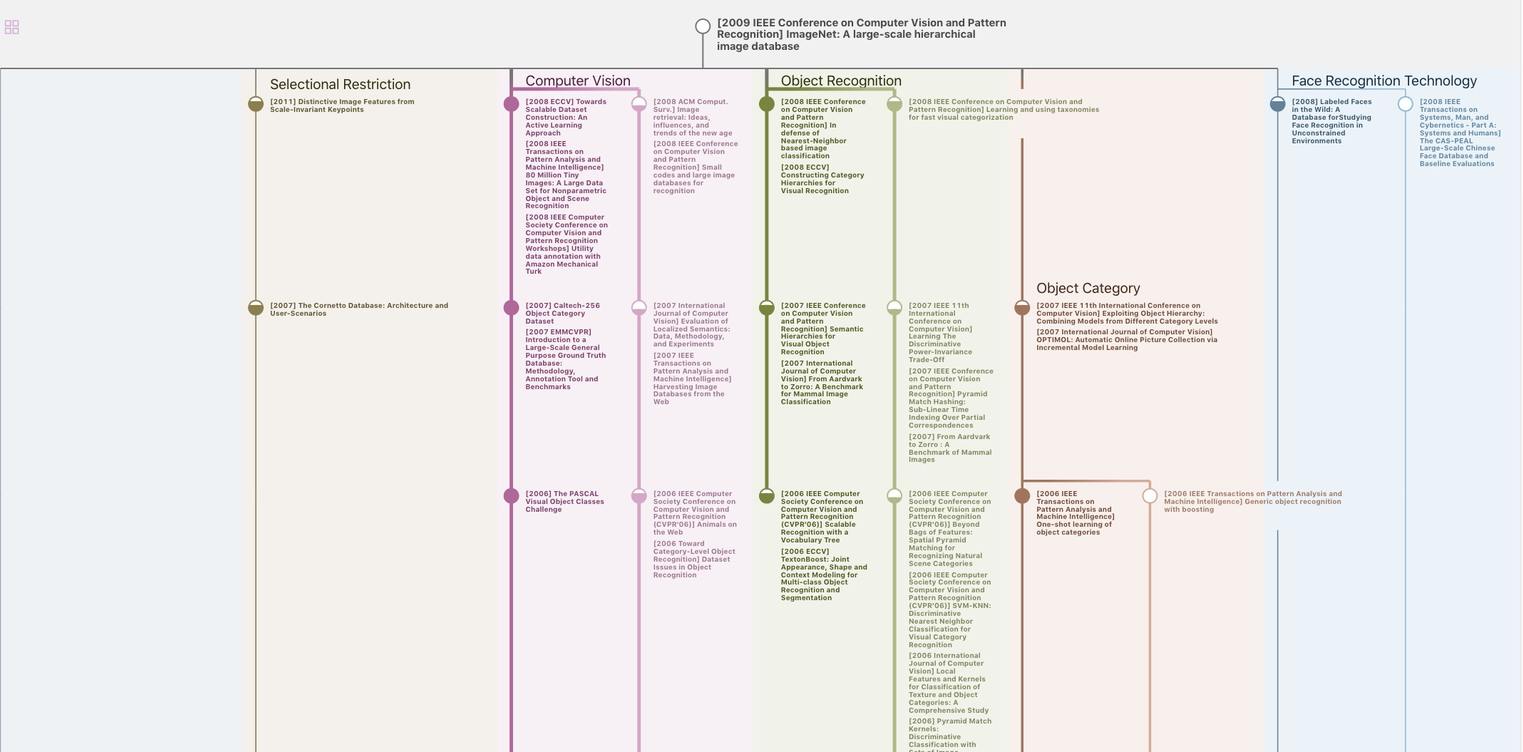
Generate MRT to find the research sequence of this paper
Chat Paper
Summary is being generated by the instructions you defined