Dual-Gradients Localization Framework With Skip-Layer Connections for Weakly Supervised Object Localization
IEEE Transactions on Multimedia(2022)
摘要
This article focuses on generating object locations in a given image while only using image-level annotations. Towards this end, we present a simple and effective training-free framework, named Dual-Gradients Localization (DGL) framework. The key idea of the proposed DGL framework is to leverage two kinds of gradients to achieve precise localization on any convolutional layer of a classification model during the testing stage. Concretely, the DGL framework is developed based on two branches: 1) Pixel-level Class Selection, leveraging gradients of the target class to identify the correlation ratio of pixels to the target class within any convolutional feature maps, and 2) Class-aware Enhanced Maps, utilizing linear relationship in gradients of the classification loss function to mine entire target object regions. To further polish the details of objects, we apply the skip-layer connections to the classification model, which concatenates the high- and low-level layers to achieve classification. In such a case, DGL with Skip-layer Connections (DGL-SC) can capture more edge information on the high-level layer. In addition, we propose a Localization Maps Selection method to evaluate the quality of the localization map and provide a way for automatically selecting localization maps produced on different layers. Extensive experiments on public ILSVRC and CUB-200-2011 datasets show the effectiveness of the proposed DGL framework. Especially, our DGL-SC obtains a new state-of-the-art gt-known localization error of 27.35% on the ILSVRC benchmark.
更多查看译文
关键词
Weakly supervised object localization,pixel-level class selection,class-aware enhanced maps,gradients of loss function,gradients of target class,skip-layer connections
AI 理解论文
溯源树
样例
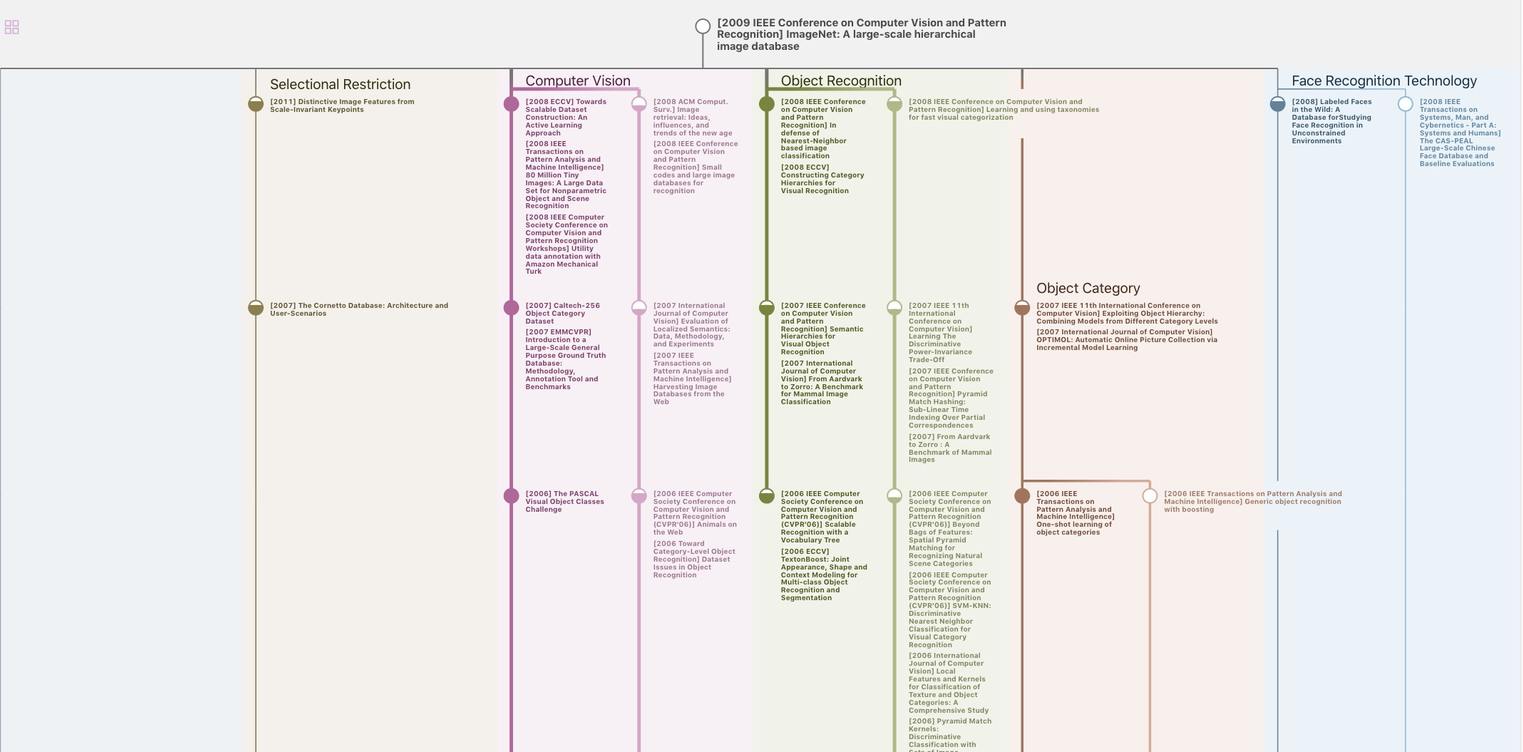
生成溯源树,研究论文发展脉络
Chat Paper
正在生成论文摘要