HiddenPose: Non-Line-of-Sight 3D Human Pose Estimation
2022 IEEE INTERNATIONAL CONFERENCE ON COMPUTATIONAL PHOTOGRAPHY (ICCP)(2022)
摘要
Nearly all existing human pose estimation techniques address the problem under the line-of-sight (LOS) setting. Many real-life applications such as rescue missions and autonomous driving, in contrast, require estimating the pose of hidden subjects. In this paper, we present a non-line-of-sight (NLOS) pose estimator, which produces a skeletal representation of hidden human poses. A brute-force approach would first conduct albedo reconstruction of a hidden subject and then apply LOS pose estimation. We show that such an implementation does not effectively exploit features unique to NLOS and subsequently yields artifacts such as missing joints. We instead first generate a comprehensive NLOS human pose dataset of 19 subjects under 9 motions. We then present a spatially aware deep learning technique based on convolutional neural networks that explicitly employ NLOS features. Comprehensive experiments on both synthetic and real data show that our new estimator is both effective and robust and can be seamlessly integrated into learning-based NLOS scene reconstruction. Our HiddenPose transient dataset contains synthetic transients with ground-truths of the volumes and the joints and real-world transients captured from our NLOS imaging system. Extensive assessments demonstrate that the HiddenPose transient dataset is valuable for effective NLOS research. We will make our data and code publicly available.
更多查看译文
关键词
Computational Photography
AI 理解论文
溯源树
样例
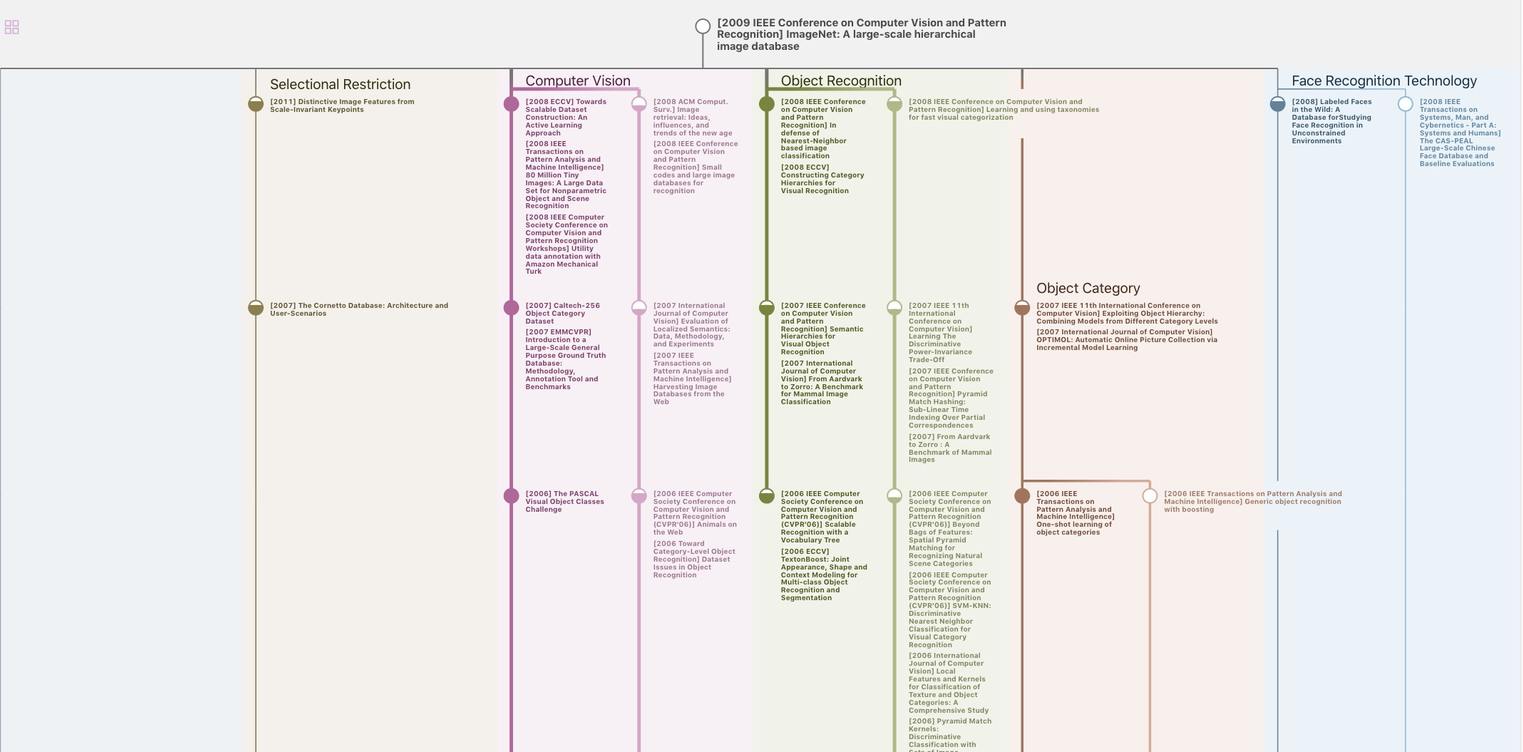
生成溯源树,研究论文发展脉络
Chat Paper
正在生成论文摘要