TEMPPO: Twin Entropy Maximized Proximal Policy Optimization
2022 27th International Computer Conference, Computer Society of Iran (CSICC)(2022)
Abstract
In recent years the Deep Reinforcement learning has been progressing so fast and more and more complicated reinforcement learning environments have been examined with new deep reinforcement algorithms. The game of football is a complicated game with a sparse reward environment. ActorCritic methods are gaining more popularity, but these methods have two important challenges. One challenge is the overestimation error and another challenge is how to explore more effectively in the big environments with sparse rewards. As to tackle the overestimation error, this paper proposes to use twin critics and as for the exploration, this paper proposes a new way to use the entropy in the objective function and cost function. This new method name is TEMPPO and it is based on the Proximal Policy optimization algorithm. In this paper, the results of the TEMPPO are tested on the Google Research Football environment.
MoreTranslated text
Key words
Actor-Critic,Deep Reinforcement Learning,Google Research Football,PPO,TEMPPO
AI Read Science
Must-Reading Tree
Example
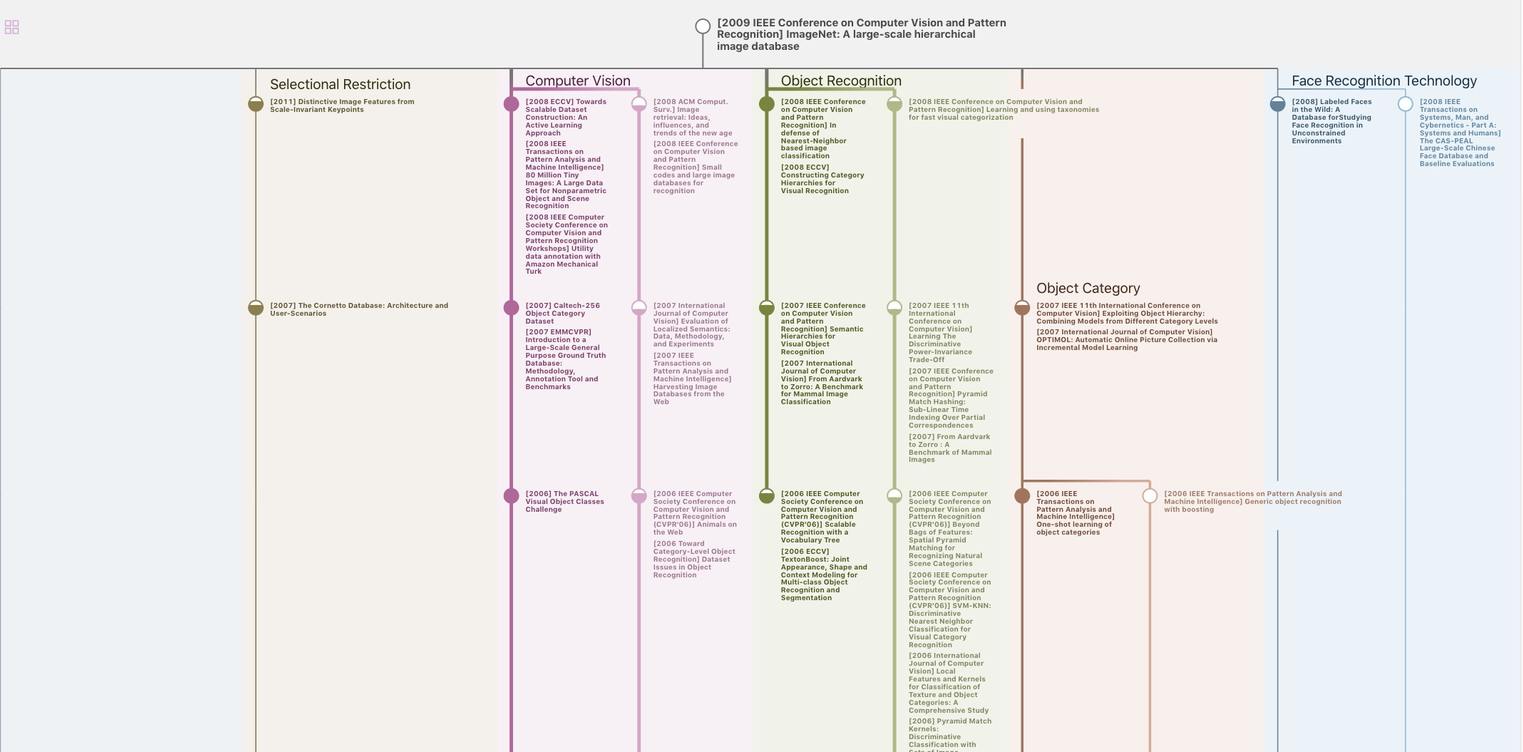
Generate MRT to find the research sequence of this paper
Chat Paper
Summary is being generated by the instructions you defined