Blind Knowledge Distillation for Robust Image Classification
arxiv(2022)
摘要
Optimizing neural networks with noisy labels is a challenging task, especially if the label set contains real-world noise. Networks tend to generalize to reasonable patterns in the early training stages and overfit to specific details of noisy samples in the latter ones. We introduce Blind Knowledge Distillation - a novel teacher-student approach for learning with noisy labels by masking the ground truth related teacher output to filter out potentially corrupted knowledge and to estimate the tipping point from generalizing to overfitting. Based on this, we enable the estimation of noise in the training data with Otsus algorithm. With this estimation, we train the network with a modified weighted cross-entropy loss function. We show in our experiments that Blind Knowledge Distillation detects overfitting effectively during training and improves the detection of clean and noisy labels on the recently published CIFAR-N dataset. Code is available at GitHub.
更多查看译文
关键词
classification,robust,knowledge
AI 理解论文
溯源树
样例
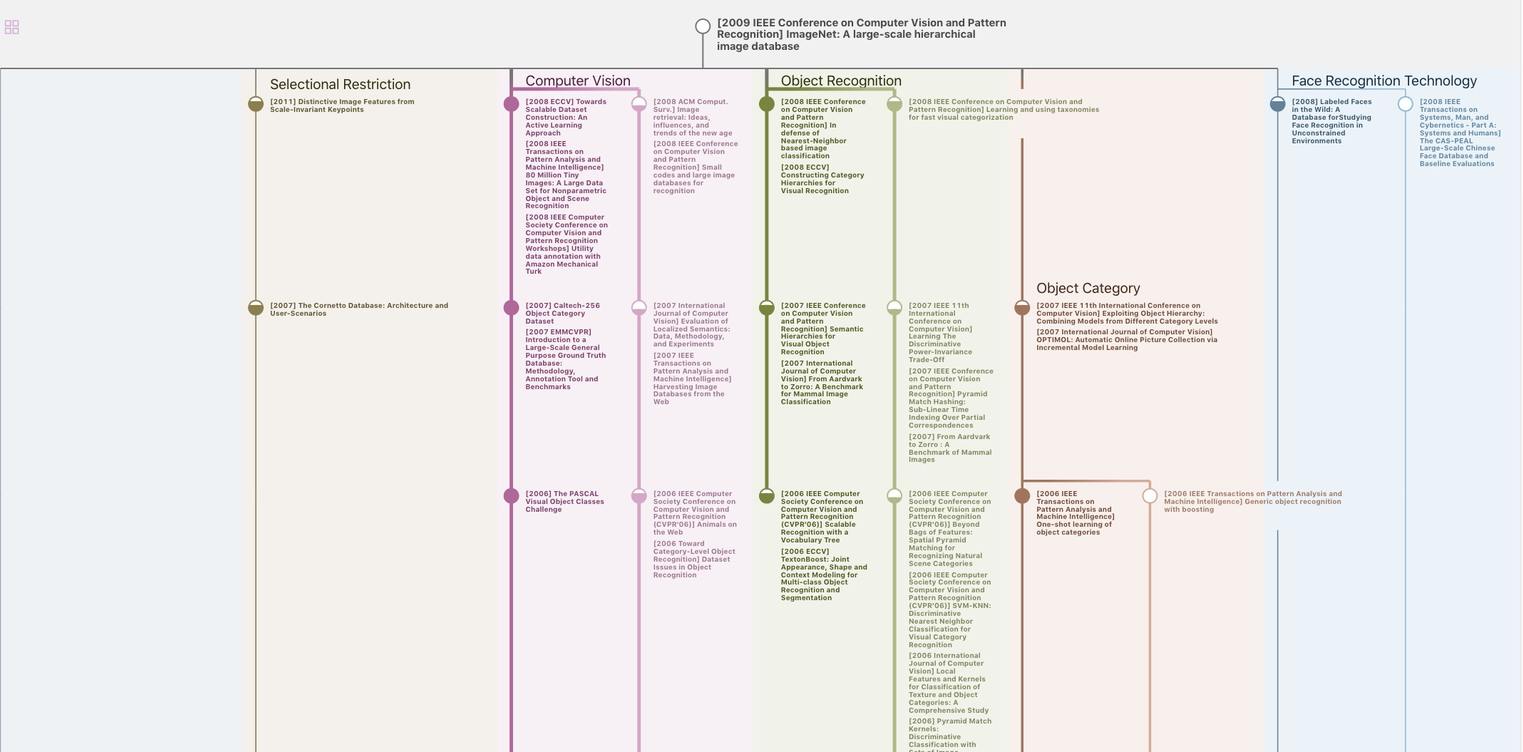
生成溯源树,研究论文发展脉络
Chat Paper
正在生成论文摘要