Learning from Long-Tailed Noisy Data with Sample Selection and Balanced Loss
CoRR(2022)
摘要
The success of deep learning depends on large-scale and well-curated training data, while data in real-world applications are commonly long-tailed and noisy. Many methods have been proposed to deal with long-tailed data or noisy data, while a few methods are developed to tackle long-tailed noisy data. To solve this, we propose a robust method for learning from long-tailed noisy data with sample selection and balanced loss. Specifically, we separate the noisy training data into clean labeled set and unlabeled set with sample selection, and train the deep neural network in a semi-supervised manner with a novel balanced loss based on model bias. Experiments on benchmarks demonstrate that our method outperforms existing state-of-the-art methods.
更多查看译文
关键词
noisy data,sample selection,learning,long-tailed
AI 理解论文
溯源树
样例
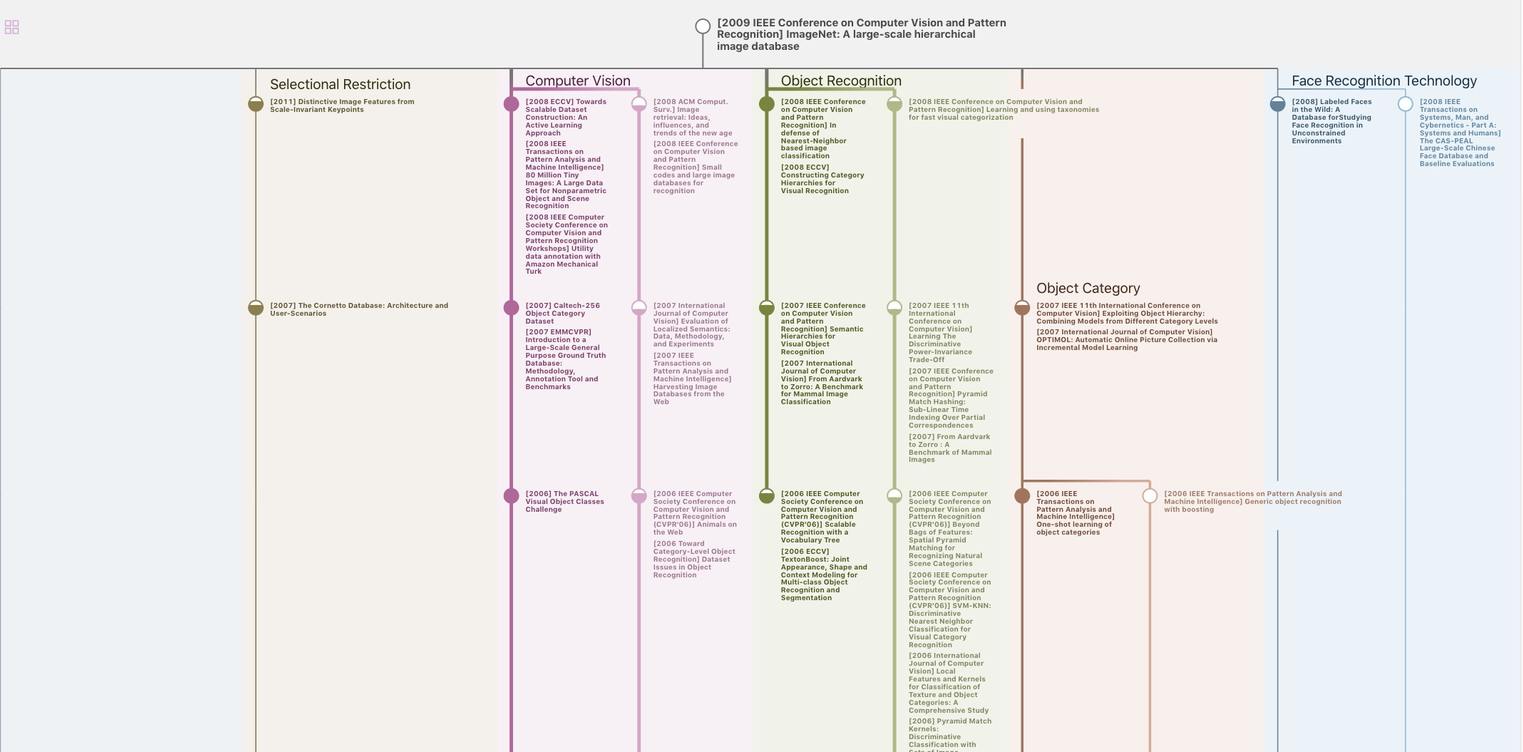
生成溯源树,研究论文发展脉络
Chat Paper
正在生成论文摘要